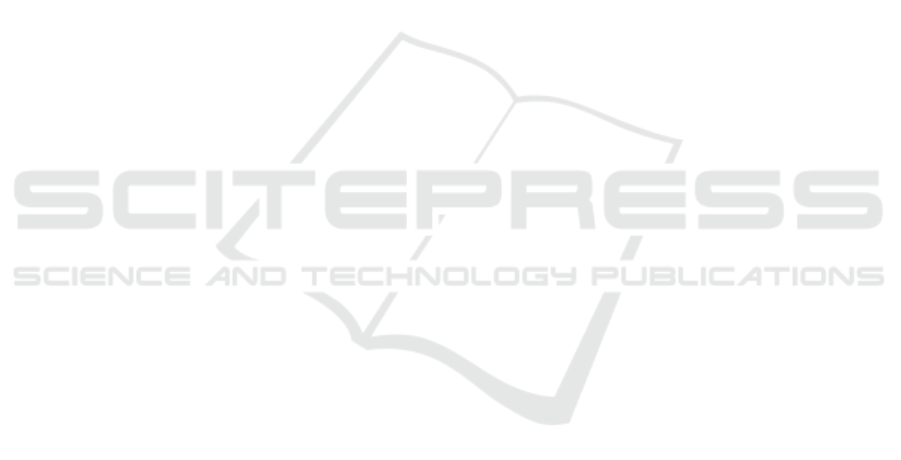
6 CONCLUSION & FUTURE
WORK
In this paper, we have presented a new method of us-
ing relational data based on relation extraction to en-
hance image captioning. This method uses two differ-
ent relational labels and the relational features, align-
ing the image and language modalities to enhance the
shared semantic space. We validate the schema by
pre-training Oscar models with relation extraction on
a public corpus with 6.5 million text-image pairs. The
VLP models based on our proposed method archive
new results on image captioning.
In the future, the sequence of visual region fea-
tures will be a breakthrough, due to the more accurate
semantic representation of image could lead to more
accurate alignment for image and text representation.
The lasted research Oscar+(Zhang et al., 2021) could
also bring more potential improvement. In the rela-
tion extraction perspective, more standard relation ex-
traction for image caption will be needed, which may
influence key components proposed in this paper.
REFERENCES
Anderson, P., He, X., Buehler, C., Teney, D., Johnson, M.,
Gould, S., and Zhang, L. (2018). Bottom-up and
top-down attention for image captioning and visual
question answering. In Proceedings of the IEEE con-
ference on computer vision and pattern recognition,
pages 6077–6086.
Chen, Y.-C., Li, L., Yu, L., El Kholy, A., Ahmed, F., Gan,
Z., Cheng, Y., and Liu, J. (2020). Uniter: Universal
image-text representation learning. In European Con-
ference on Computer Vision, pages 104–120. Springer.
Devlin, J., Chang, M.-W., Lee, K., and Toutanova, K.
(2018). Bert: Pre-training of deep bidirectional trans-
formers for language understanding. arXiv preprint
arXiv:1810.04805.
Han, X., Gao, T., Yao, Y., Ye, D., Liu, Z., and Sun, M.
(2019). OpenNRE: An open and extensible toolkit for
neural relation extraction. In Proceedings of EMNLP-
IJCNLP: System Demonstrations, pages 169–174.
Hao, W., Li, C., Li, X., Carin, L., and Gao, J. (2020).
Towards learning a generic agent for vision-and-
language navigation via pre-training. In Proceedings
of the IEEE/CVF Conference on Computer Vision and
Pattern Recognition, pages 13137–13146.
Li, G., Duan, N., Fang, Y., Gong, M., and Jiang, D. (2020a).
Unicoder-vl: A universal encoder for vision and lan-
guage by cross-modal pre-training. In Proceedings of
the AAAI Conference on Artificial Intelligence, vol-
ume 34, pages 11336–11344.
Li, L. H., Yatskar, M., Yin, D., Hsieh, C.-J., and Chang,
K.-W. (2019). Visualbert: A simple and performant
baseline for vision and language. arXiv preprint
arXiv:1908.03557.
Li, X., Yin, X., Li, C., Zhang, P., Hu, X., Zhang, L., Wang,
L., Hu, H., Dong, L., Wei, F., et al. (2020b). Os-
car: Object-semantics aligned pre-training for vision-
language tasks. In European Conference on Computer
Vision, pages 121–137. Springer.
Lin, T.-Y., Maire, M., Belongie, S., Hays, J., Perona, P., Ra-
manan, D., Doll
´
ar, P., and Zitnick, C. L. (2014). Mi-
crosoft COCO: Common objects in context. In Euro-
pean conference on computer vision, pages 740–755.
Springer.
Lu, J., Batra, D., Parikh, D., and Lee, S. (2019). Vilbert:
Pretraining task-agnostic visiolinguistic representa-
tions for vision-and-language tasks. arXiv preprint
arXiv:1908.02265.
Ranzato, M., Chopra, S., Auli, M., and Zaremba, W. (2015).
Sequence level training with recurrent neural net-
works. arXiv preprint arXiv:1511.06732.
Ren, S., He, K., Girshick, R., and Sun, J. (2015). Faster
r-cnn: Towards real-time object detection with region
proposal networks. arXiv preprint arXiv:1506.01497.
Rennie, S. J., Marcheret, E., Mroueh, Y., Ross, J., and Goel,
V. (2017). Self-critical sequence training for image
captioning. In Proceedings of the IEEE conference on
computer vision and pattern recognition, pages 7008–
7024.
Su, W., Zhu, X., Cao, Y., Li, B., Lu, L., Wei, F., and
Dai, J. (2019). Vl-bert: Pre-training of generic
visual-linguistic representations. arXiv preprint
arXiv:1908.08530.
Sun, C., Myers, A., Vondrick, C., Murphy, K., and Schmid,
C. (2019). Videobert: A joint model for video and
language representation learning. In Proceedings of
the IEEE/CVF International Conference on Computer
Vision, pages 7464–7473.
Tan, H. and Bansal, M. (2019). Lxmert: Learning cross-
modality encoder representations from transformers.
arXiv preprint arXiv:1908.07490.
Vaswani, A., Shazeer, N., Parmar, N., Uszkoreit, J., Jones,
L., Gomez, A. N., Kaiser, Ł., and Polosukhin, I.
(2017). Attention is all you need. In Advances in
neural information processing systems, pages 5998–
6008.
Vinyals, O., Toshev, A., Bengio, S., and Erhan, D. (2015).
Show and tell: A neural image caption generator. In
Proceedings of the IEEE conference on computer vi-
sion and pattern recognition, pages 3156–3164.
Zhang, P., Li, X., Hu, X., Yang, J., Zhang, L., Wang, L.,
Choi, Y., and Gao, J. (2021). Vinvl: Revisiting vi-
sual representations in vision-language models. arXiv
preprint arXiv:2101.00529.
Zhou, L., Palangi, H., Zhang, L., Hu, H., Corso, J., and
Gao, J. (2020). Unified vision-language pre-training
for image captioning and vqa. In Proceedings of
the AAAI Conference on Artificial Intelligence, vol-
ume 34, pages 13041–13049.
Learning Cross-modal Representations with Multi-relations for Image Captioning
353