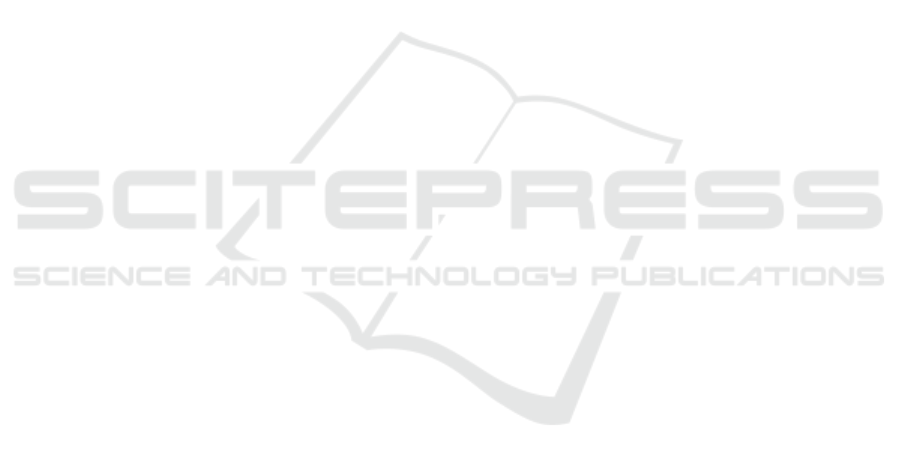
REFERENCES
Abedi, A. and Khan, S. (2021a). Affect-driven engage-
ment measurement from videos. arXiv preprint
arXiv:2106.10882.
Abedi, A. and Khan, S. S. (2021b). Improving state-of-the-
art in detecting student engagement with resnet and
tcn hybrid network. The 18th Conference on Robots
and Vision.
Arjovsky, M., Chintala, S., and Bottou, L. (2017). Wasser-
stein generative adversarial networks. In Proceed-
ings of the 34th International Conference on Ma-
chine Learning - Volume 70, ICML’17, page 214–223.
JMLR.org.
Ashwin, T. and Guddeti, R. M. R. (2019). Automatic de-
tection of students’ affective states in classroom envi-
ronment using hybrid convolutional neural networks.
Education and Information Technologies, pages 1–29.
Baltru
ˇ
saitis, T., Robinson, P., and Morency, L.-P. (2016).
Openface: an open source facial behavior analysis
toolkit. In Applications of Computer Vision (WACV),
2016 IEEE Winter Conference on, pages 1–10. IEEE.
Cao, Q., Shen, L., Xie, W., Parkhi, O. M., and Zisserman,
A. (2018). Vggface2: A dataset for recognising faces
across pose and age. In 2018 13th IEEE International
Conference on Automatic Face Gesture Recognition
(FG 2018), pages 67–74.
Carreira, J. and Zisserman, A. (2017). Quo vadis, action
recognition? a new model and the kinetics dataset.
In proceedings of the IEEE Conference on Computer
Vision and Pattern Recognition, pages 6299–6308.
Drossos, K., Magron, P., and Virtanen, T. (2019). Unsu-
pervised adversarial domain adaptation based on the
wasserstein distance for acoustic scene classification.
arXiv preprint arXiv:1904.10678.
Geng, L., Xu, M., Wei, Z., and Zhou, X. (2019). Learning
deep spatiotemporal feature for engagement recogni-
tion of online courses. In 2019 IEEE Symposium
Series on Computational Intelligence (SSCI), pages
442–447. IEEE.
Grafsgaard, J., Wiggins, J. B., Boyer, K. E., Wiebe, E. N.,
and Lester, J. (2013). Automatically recognizing fa-
cial expression: Predicting engagement and frustra-
tion. In Educational Data Mining 2013.
Gupta, A., D’Cunha, A., Awasthi, K., and Balasubra-
manian, V. (2016). Daisee: Towards user en-
gagement recognition in the wild. arXiv preprint
arXiv:1609.01885.
Gupta, S. K., Ashwin, T., and Guddeti, R. M. R. (2019).
Students’ affective content analysis in smart class-
room environment using deep learning techniques.
Multimedia Tools and Applications, pages 1–28.
He, K., Zhang, X., Ren, S., and Sun, J. (2016). Deep resid-
ual learning for image recognition. In Proceedings of
the IEEE conference on computer vision and pattern
recognition, pages 770–778.
Huang, T., Mei, Y., Zhang, H., Liu, S., and Yang, H. (2019).
Fine-grained engagement recognition in online learn-
ing environment. In 2019 IEEE 9th international
conference on electronics information and emergency
communication (ICEIEC), pages 338–341. IEEE.
Liao, J., Liang, Y., and Pan, J. (2021). Deep facial spa-
tiotemporal network for engagement prediction in on-
line learning. Applied Intelligence, pages 1–13.
Long, M., Zhu, H., Wang, J., and Jordan, M. I. (2017).
Deep transfer learning with joint adaptation networks.
In Proceedings of the 34th International Conference
on Machine Learning-Volume 70, pages 2208–2217.
JMLR. org.
Nezami, O. M., Dras, M., Hamey, L., Richards, D., Wan, S.,
and Paris, C. (2019). Automatic recognition of student
engagement using deep learning and facial expression.
In Joint European Conference on Machine Learning
and Knowledge Discovery in Databases, pages 273–
289. Springer.
Raca, M. (2015). Camera-based estimation of student’s at-
tention in class. Technical report, EPFL.
Thomas, C. and Jayagopi, D. B. (2017). Predicting stu-
dent engagement in classrooms using facial behavioral
cues. In Proceedings of the 1st ACM SIGCHI interna-
tional workshop on multimodal interaction for educa-
tion, pages 33–40.
Thomas, C., Nair, N., and Jayagopi, D. B. (2018). Predict-
ing engagement intensity in the wild using temporal
convolutional network. In Proceedings of the 2018 on
International Conference on Multimodal Interaction,
pages 604–610. ACM.
Wang, M. and Deng, W. (2018). Deep visual domain adap-
tation: A survey. Neurocomputing, 312:135–153.
Wang, Y., Kotha, A., Hong, P.-h., and Qiu, M. (2020). Au-
tomated student engagement monitoring and evalua-
tion during learning in the wild. In 2020 7th IEEE In-
ternational Conference on Cyber Security and Cloud
Computing (CSCloud)/2020 6th IEEE International
Conference on Edge Computing and Scalable Cloud
(EdgeCom), pages 270–275. IEEE.
Whitehill, J., Serpell, Z., Lin, Y.-C., Foster, A., and Movel-
lan, J. R. (2014). The faces of engagement: Auto-
matic recognition of student engagementfrom facial
expressions. IEEE Transactions on Affective Comput-
ing, 5(1):86–98.
Wilson, G. and Cook, D. J. (2020). A survey of unsuper-
vised deep domain adaptation. ACM Transactions on
Intelligent Systems and Technology (TIST), 11(5):1–
46.
Yang, J., Wang, K., Peng, X., and Qiao, Y. (2018). Deep
recurrent multi-instance learning with spatio-temporal
features for engagement intensity prediction. In Pro-
ceedings of the 2018 on International Conference on
Multimodal Interaction, pages 594–598. ACM.
Zhang, H., Xiao, X., Huang, T., Liu, S., Xia, Y., and Li,
J. (2019). An novel end-to-end network for auto-
matic student engagement recognition. In 2019 IEEE
9th International Conference on Electronics Informa-
tion and Emergency Communication (ICEIEC), pages
342–345. IEEE.
Student Engagement from Video using Unsupervised Domain Adaptation
125