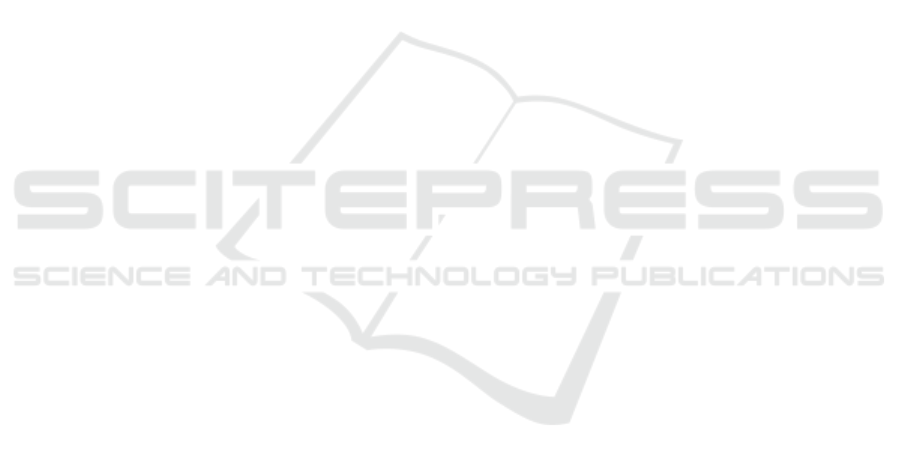
vative, and wants to see clear value from initiatives.
Huttunen et al. (Huttunen et al., 2019) showed
that shared spaces for data sharing are not necessar-
ily needed, bilateral solutions may give just as high
value. The advantage is that those may result in less
complex agreements, and remove some of the more
challenging competition and privacy issues. Eventu-
ally this was the outcome of this project, two compan-
ies collaborating on data about car insurance.
In the initial research questions for the project we
aimed to get insights into what kind of solutions were
possible in such a project. What we observed is that
beyond the restricted black box solution, which is
not yet operative, there are basically are no solutions
found yet. Very much stopped due to the inability to
handle the initial constraint of ownership. Perhaps, if
one had a focused on a demonstration solution from
early on, there would been more engagement in trying
to solve the ownership issue.
This project is a case study with a limited set of
interview respondents, and will of course not general-
ise to other situations, and in particular to other pro-
jects on data sharing for insurance fraud detection.
However, it illustrate some of the problems such a
project may meet, and emphasise the need to focus
on early demonstration of a viable system, focus on
handling trust among data sharers, and commitment
among managers to assign the necessary people re-
sources. As mentioned, insurance fraud has large so-
cietal costs, and solutions to reduce it will have great
value for companies, customers, and society in gen-
eral.
7 CONCLUSION
This paper describes a case study of a data sharing
project in the insurance industry, where data sharing
was hoped to be a driver for innovation. The pro-
ject was in large parts unsuccessful. We have ob-
served how data sharing among competitors becomes
problematic due to several forms of regulations, di-
vergent interests, lack of trust, lack of management
support, and other issues. The case study shows that
data sharing among companies is difficult to realise,
even though there is a large potential for economic
gains for all parties. One observation is that early
demonstrators of solutions, for example, in a regulat-
ory sandbox, could contribute to increased trust, man-
agement support, and engagement.
REFERENCES
Arnaut, C., Pont, M., Scaria, E., Berghmans, A., and Le-
conte, S. (2018). Study on data sharing between com-
panies in Europe. everis Benelux.
Benbasat, I., Goldstein, D. K., and Mead, M. (1987). The
Case Research Strategy in Studies of Information Sys-
tems. MIS Quarterly, 11(3):369–386.
Celtekligil, K. and Adiguzel, Z. (2019). Evaluation of Data
Sharing in Production Firms and Innovation Orienta-
tion in The Effect of Management Capability on Op-
erational Performance. Procedia Computer Science,
158:781–789.
Eckartz, S. M., Hofman, W. J., and Van Veenstra, A. F.
(2014). A Decision Model for Data Sharing. In
Janssen, M., Scholl, H. J., Wimmer, M. A., and Ban-
nister, F., editors, Electronic Government, Lecture
Notes in Computer Science, pages 253–264, Berlin,
Heidelberg. Springer.
Finance Innovation (2021). Detection of Insurance Fraud.
https://financeinnovation.no/innovation-projects/
detection-of-insurance-fraud. Accessed: 2021-08-17.
Finans Norge (2019). Forsikringssvindel i Norge - svikstat-
isikk 2019 (Eng: Insurance fraud in Norway - fraud
statistics 2019).
Grabus, S. and Greenberg, J. (2017). Toward a Metadata
Framework for Sharing Sensitive and Closed Data:
An Analysis of Data Sharing Agreement Attributes.
In Garoufallou, E., Virkus, S., Siatri, R., and Kout-
somiha, D., editors, Metadata and Semantic Research,
Communications in Computer and Information Sci-
ence, pages 300–311, Cham. Springer International
Publishing.
Huttunen, H., Sepp
¨
al
¨
a, T., L
¨
ahteenm
¨
aki, I., and Mattila, J.
(2019). What Are the Benefits of Data Sharing? Unit-
ing Supply Chain and Platform Economy Perspect-
ives. Technical Report 93.
Jetzek, T., Avital, M., and Bjørn-Andersen, N. (2013). Gen-
erating Value from Open Government Data. ICIS 2013
Proceedings.
Power, D. and Power, M. (2015). Sharing and Analyzing
Data to Reduce Insurance Fraud. MWAIS 2015 Pro-
ceedings.
Richter, H. and Slowinski, P. R. (2019). The Data Sharing
Economy: On the Emergence of New Intermediaries.
IIC - International Review of Intellectual Property and
Competition Law, 50(1):4–29.
Tang, C., Plasek, J. M., and Bates, D. W. (2018). Rethinking
Data Sharing at the Dawn of a Health Data Economy:
A Viewpoint. Journal of Medical Internet Research,
20(11):e11519.
ICEIS 2022 - 24th International Conference on Enterprise Information Systems
98