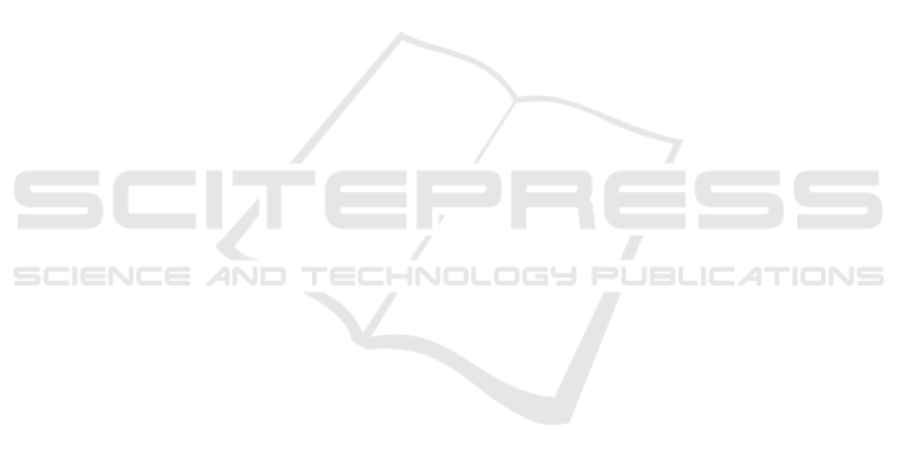
REFERENCES
Banerjee, A. V., Cole, S., Duflo, E., and Linden, L. (2007).
Remedying education: Evidence from two random-
ized experiments in india. The Quarterly Journal of
Economics, 122(3):1235–1264.
Banerjee, A. V. and Duflo, E. (2011). Poor economics : a
radical rethinking of the way to fight global poverty,
chapter 4 - Top of the Class, pages 188–196. Publi-
cAffairs.
Birnbaum, A., Lord, F., and Novick, M. (1968). Statistical
theories of mental test scores. Some latent trait mod-
els and their use in inferring an examinee’s ability.
Addison-Wesley, Reading, MA.
Doignon, J.-P. and Falmagne, J.-C. (2012). Knowledge
spaces. Springer Science & Business Media.
Eggen, T. J. (2012). Computerized adaptive testing item
selection in computerized adaptive learning systems.
Psychometrics in Practice at RCEC, page 11.
ElKomy, M., Abdelrahman, Y., Funk, M., Dingler, T.,
Schmidt, A., and Abdennadher, S. (2017). Abbas: an
adaptive bio-sensors based assistive system. In Pro-
ceedings of the 2017 CHI conference extended ab-
stracts on human factors in computing systems, pages
2543–2550.
Freedle, R. O. and Dur
´
an, R. P. (1987). Cognitive and
linguistic analyses of test performance, volume 22.
Ablex Pub.
Frick, T. W. (1989). Bayesian adaptation during
computer-based tests and computer-guided practice
exercises. Journal of Educational Computing Re-
search, 5(1):89–114.
Frick, T. W. (1992). Computerized adaptive mastery tests
as expert systems. Journal of Educational Computing
Research, 8(2):187–213.
Funk, M., Dingler, T., Cooper, J., and Schmidt, A. (2015).
Stop helping me-i’m bored! why assembly assistance
needs to be adaptive. In Adjunct Proceedings of the
2015 ACM International Joint Conference on Perva-
sive and Ubiquitous Computing and Proceedings of
the 2015 ACM International Symposium on Wearable
Computers, pages 1269–1273.
Giannandrea, L. and Sansoni, M. (2013). A literature re-
view on intelligent tutoring systems and on student
profiling. Learning & Teaching with Media & Tech-
nology, 287:287–294.
Kingsbury, G. G. and Weiss, D. J. (1983). A comparison
of irt-based adaptive mastery testing and a sequential
mastery testing procedure. In New horizons in testing,
pages 257–283. Elsevier.
Linssen, J. (2011). Adaptive learning in an educational
game–adapting game complexity to gameplay in-
creases efficiency of learning. Master’s thesis.
Lord, F. (1980a). Application of item response theory to
practical testing problems. first. Hilsdale, New Jersey,
EUA: Lawrence Erlbaum Associates.
Lord, F. M. (1980b). Applications of item response theory
to practical testing problems. Routledge.
Luk, H. (1991). An empirical comparison of an expert sys-
tems approach and an irt approach to computer-based
adaptive mastery testing.
Pandarova, I., Schmidt, T., Hartig, J., Boubekki, A., Jones,
R. D., and Brefeld, U. (2019). Predicting the difficulty
of exercise items for dynamic difficulty adaptation in
adaptive language tutoring. International Journal of
Artificial Intelligence in Education, 29(3):342–367.
Park, J. Y., Joo, S.-H., Cornillie, F., van der Maas, H. L., and
Van den Noortgate, W. (2019). An explanatory item
response theory method for alleviating the cold-start
problem in adaptive learning environments. Behavior
research methods, 51(2):895–909.
Pearce, J. M., Ainley, M., and Howard, S. (2005). The ebb
and flow of online learning. Computers in human be-
havior, 21(5):745–771.
Pedro, F., Subosa, M., Rivas, A., and Valverde, P. (2019).
Artificial intelligence in education: Challenges and
opportunities for sustainable development.
Pliakos, K., Joo, S.-H., Park, J. Y., Cornillie, F., Vens, C.,
and Van den Noortgate, W. (2019). Integrating ma-
chine learning into item response theory for address-
ing the cold start problem in adaptive learning sys-
tems. Computers & Education, 137:91–103.
Reckase, M. D. (1983). A procedure for decision mak-
ing using tailored testing. In New horizons in testing,
pages 237–255. Elsevier.
Salah, J., Abdelrahman, Y., Dakrouni, A., and Abdennad-
her, S. (2018). Judged by the cover: Investigating the
effect of adaptive game interface on the learning expe-
rience. In Proceedings of the 17th International Con-
ference on Mobile and Ubiquitous Multimedia, pages
215–225. ACM.
Schmitt, S. A. (1969). Measuring uncertainty: An ele-
mentary introduction to Bayesian statistics. Addison-
Wesley.
Solovey, E. T., Lalooses, F., Chauncey, K., Weaver, D.,
Parasi, M., Scheutz, M., Sassaroli, A., Fantini, S.,
Schermerhorn, P., Girouard, A., et al. (2011). Sens-
ing cognitive multitasking for a brain-based adaptive
user interface. In Proceedings of the SIGCHI Confer-
ence on Human Factors in Computing Systems, pages
383–392.
Spray, J. A. (1993). Multiple-category classification using
a sequential probability ratio test.
Wald, A. (1947). Sequential analysis, john wiley & sons.
New York, NY.
Wauters, K., Desmet, P., and Van Den Noortgate, W. (2010).
Adaptive item-based learning environments based on
the item response theory: Possibilities and challenges.
Journal of Computer Assisted Learning, 26(6):549–
562.
Yoes, M. (1995). An updated comparison of micro-
computer based item parameter estimation procedures
used with the 3-parameter irt model. Saint Paul, MN:
Assessment Systems Corporation.
Zawacki-Richter, O., Mar
´
ın, V. I., Bond, M., and Gou-
verneur, F. (2019). Systematic review of re-
search on artificial intelligence applications in higher
education–where are the educators? International
Journal of Educational Technology in Higher Educa-
tion, 16(1):1–27.
CSEDU 2022 - 14th International Conference on Computer Supported Education
198