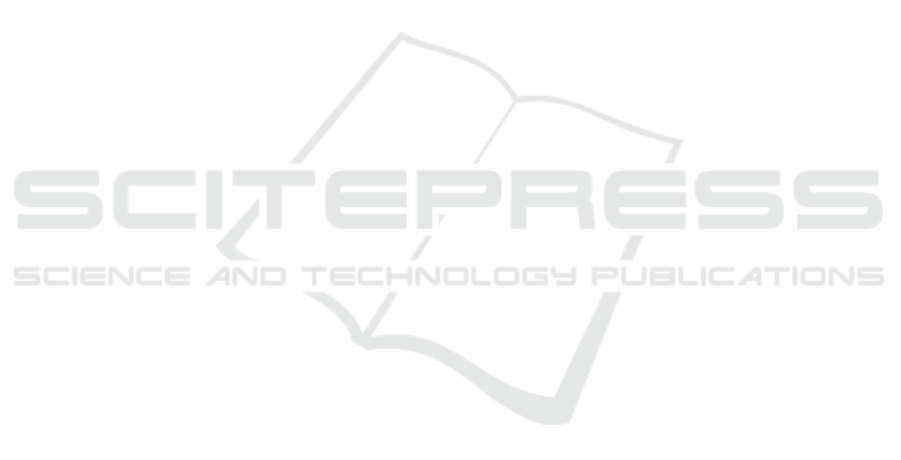
fastKDE. Computational Statistics & Data Analysis,
101:148–160.
Prestele, R., Alexander, P., Rounsevell, M. D. A., Arneth,
A., Calvin, K., Doelman, J., Eitelberg, D. A., En-
gstr
¨
om, K., Fujimori, S., Hasegawa, T., Havlik, P.,
Humpen
¨
oder, F., Jain, A. K., Krisztin, T., Kyle, P.,
Meiyappan, P., Popp, A., Sands, R. D., Schaldach, R.,
Sch
¨
ungel, J., Stehfest, E., Tabeau, A., Meijl, H. V.,
Vliet, J. V., and Verburg, P. H. (2016). Hotspots of
uncertainty in land-use and land-cover change pro-
jections: a global-scale model comparison. Global
Change Biology, 22(12):3967–3983.
Rudemo, M. (1982). Empirical Choice of Histograms and
Kernel Density Estimators. Scandinavian Journal of
Statistics, 9(2):65–78.
Sain, S. R., Baggerly, K. A., and Scott, D. W. (1994). Cross-
validation of multivariate densities. Journal of the
American Statistical Association, 89(427):807–817.
Scott, D. (2015). Multivariate density estimation: Theory,
practice, and visualization: Second edition. Wiley.
Soares-Filho, B. S., Coutinho Cerqueira, G., and
Lopes Pennachin, C. (2002). Dinamica — A stochas-
tic cellular automata model designed to simulate the
landscape dynamics in an Amazonian colonization
frontier. Ecological Modelling, 154(3):217–235.
Terrell, G. (1990). The Maximal Smoothing Principle in
Density Estimation. Journal of the American Statisti-
cal Association, 85(410):470–477.
van Vliet, J., Bregt, A. K., Brown, D. G., van Delden, H.,
Heckbert, S., and Verburg, P. H. (2016). A review
of current calibration and validation practices in land-
change modeling. Environmental Modelling & Soft-
ware, 82:174–182.
van Vliet, J., Malek, Z., and Verbug, P. (2015). The CLU-
Mondo land use change model, manual and exercises.
Vannier, C., Bierry, A., Longaretti, P.-Y., Nettier, B., Cor-
donnier, T., Chauvin, C., Bertrand, N., Qu
´
etier, F.,
Lasseur, R., and Lavorel, S. (2019a). Co-constructing
future land-use scenarios for the Grenoble region,
France. Landscape and Urban Planning, 190:103614.
Vannier, C., Lasseur, R., Crouzat, E., Byczek, C., Lafond,
V., Cordonnier, T., Longaretti, P.-Y., and Lavorel, S.
(2019b). Mapping ecosystem services bundles in a
heterogeneous mountain region. Ecosystems and Peo-
ple, 15:74–88.
Vannier, C., Lefebvre, J., Longaretti, P.-Y., and Lavorel, S.
(2016). Patterns of Landscape Change in a Rapidly
Urbanizing Mountain Region. Cybergeo.
Verburg, P. H., Alexander, P., Evans, T., Magliocca, N. R.,
Malek, Z., Rounsevell, M. D., and van Vliet, J. (2019).
Beyond land cover change: towards a new generation
of land use models. Current Opinion in Environmental
Sustainability, 38:77–85.
Verburg, P. H., de Koning, G. H. J., Kok, K., Veldkamp,
A., and Bouma, J. (1999). A spatial explicit allocation
procedure for modelling the pattern of land use change
based upon actual land use. Ecological Modelling,
116(1):45–61.
Wand, M. and Jones, C. (1994). Multivariate plug-in band-
width selection. Computational Statistics, 9(2):97–
116.
Wand, M. P. (1992). Error analysis for general multtvariate
kernel estimators. Journal of Nonparametric Statis-
tics, 2(1):1–15.
Wells, J. R. and Ting, K. M. (2019). A new simple and
efficient density estimator that enables fast systematic
search. Pattern Recognition Letters, 122:92–98.
APPENDIX
Case Study Transition Probability
Function
The parameters used to define the function P
∗
(v|u,y)
in Eq. (4) are the following:
µ = (150, 0,0) (5)
Σ =
25
2
843 325
843 10
2
−16.3
325 −16.3 10
2
(6)
D = {y | 0 ≤ y
0
≤ 616, 0 ≤ y
1
≤ 15, 0 ≤ y
2
≤ 60}
(7)
Models Parameters
In section 5, we use various land use change models
with the following parameters.
Dinamica EGO
We have used version 5.2.1 and all the calculations
were performed on a single CPU. The binning param-
eters are the following. The parameter increment is
fixed at 15 meters for the elevation, 5
o
for the slope
and 10 meters for the distance to urban areas. The
minimum delta, the maximum delta and the tolerance
angle are respectively fixed at 50, 500,000 and 5.0 for
all explanatory variables.
Idrisi LCM
We have an Idrisi Selva license, which is relatively old
(17.00). The estimation by logistic regression is done
without sampling. The parameters of SimWeight are
the default ones with notably the sample size fixed at
1000. All the default parameters of MLP are kept.
CLUMondo
We have used version 1.4.0. The sampling parameter
is fixed to 30% of all observations. The number of
cells distance between samples is fixed to 2 with no
data values excluding and balanced sample enabled.
Clumpy
The KDE parameter q (section 3.1.2) is fixed to 51.
This is the only user-defined parameter in CLUMPY.
This default value should be appropriate for most ap-
plications.
GISTAM 2022 - 8th International Conference on Geographical Information Systems Theory, Applications and Management
36