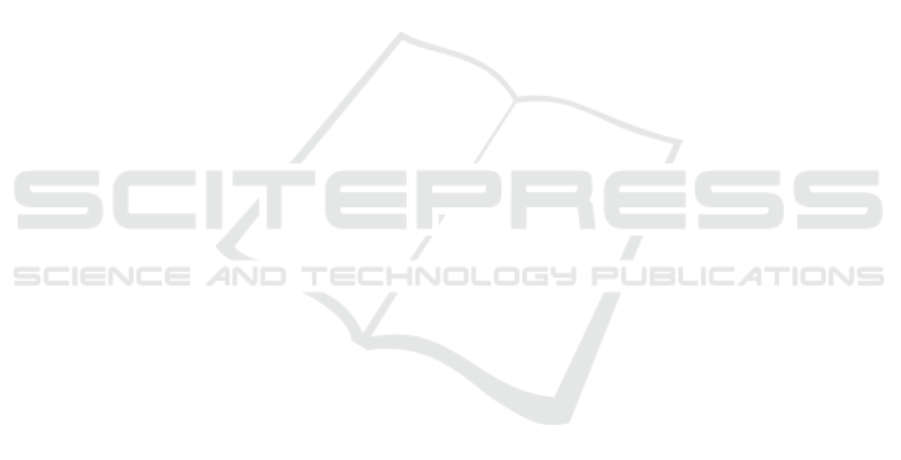
The survey, which collects data monthly, aims to
monitor the socio-economic effects of the pandemic
COVID-19 with real-time evolution. The study is part
of the World Bank’s Living Standards Measurement
Survey program to provide support to countries to
help mitigate the spread and impact of the new coro-
navirus disease.
The data collection is done through several tele-
phone calls to households located throughout Nigeria.
Each month, Nigerian households across the country
are asked about topics that are likely to be affected by
COVID-19 restrictions. Thus, the Nigeria COVID-19
NLPS intends to monitor the socio-economic effects
of the COVID-19 pandemic that will contribute to the
development of policies that will mitigate the negative
impacts on the population.
This paper performs the triadic analysis applied to
this base, using data collected in 3 waves of monthly
interviews, which had as areas of interest (i) access to
basic services, (ii) behavior, (iii) education, and (iv)
access to medical treatment.
The survey was conducted with 1,800 individuals
who are components and residents of the population
of Nigeria, aged eighteen (18) years and above.
In order to analyze the areas of interest mentioned,
four questions were selected related to areas of behav-
ior and status in society, such as: washing hands with
soap, use of mask in public, need for medical treat-
ment, and frequency of children and adolescents with
school activities.
The resulting analysis will allow us to associate
such behaviors with the spread of COVID-19 and the
socioeconomic impacts on the country.
The first wave of Nigeria COVID-19 NLPS tele-
phone interviews considered for the present work was
conducted between June 2 and 16, 2020. The second
took place between July 2-16, 2020, and the third be-
tween the dates of August 9-24, 2020.
To bring the intended result in the present paper,
relating the behavioral results of the Nigeria COVID-
19 NLPS survey to the spread of COVID-19 during
June, July, and August 2020 in Nigeria, the base Nige-
ria: Coronavirus Pandemic Country Profile was used,
which determines the estimate of the effective repro-
duction rate (R) of COVID-19. The reproduction
rate represents the average number of new infections
caused by a single infected individual.
The aforementioned statistics and survey Nige-
ria: Coronavirus Pandemic Country Profile, was con-
ducted by Our World in Data. The database provided,
in addition to the many linked graphs, uses the com-
plete set of data on confirmed cases and deaths from
Johns Hopkins University (JHU) and the European
Centre for Disease Prevention and Control (ECDC).
This Johns Hopkins University dataset is main-
tained by the Center for Systems Science and Engi-
neering (CSSE). Since January 22, 2020, it has pub-
lished updates on confirmed cases and deaths for all
countries and updates its data several times a day. The
data used from this database comes from the govern-
ment, national and subnational agencies in Nigeria.
4.2 Methods
To achieve the goal of the paper, it was necessary to
perform data transformation in a triadic context, us-
ing the Nigeria COVID-19 NLPS database as input
to the concept extraction algorithm. To do this, the
following process was carried out:
1) A preprocessing of the base was performed.
Relevant and consistent questions were filtered and
selected between the collection waves. After selecting
the questions, the respective answers were discretized
according to each context, so that there was no in-
terference in the configuration and purpose of each
question.
2) Grouping the preprocessed data into their
waves. After preparing the questions and discretizing
the answers, the data from each wave were grouped in
the same table, keeping the reference of each wave for
later interpretation of the implication rules generated.
3) For the rule generation the Lattice Miner 2.0
tool was used, a data mining prototype developed un-
der the supervision of Professor Rokia Missaoui by
the LARIM research laboratory of the Universit
´
e du
Qu
´
ebec (Missaoui and Emamirad, 2017). It is a public
domain Java platform whose main functions include
all the low-level operations and structures to represent
and manipulate input data, structures, and association
rules. The platform allows the generation of clusters,
called formal concepts, and association rules, includ-
ing logical implications, given a binary relationship
between a collection of objects and a set of attributes
or properties.
Among the functions made available by Lattice
Miner 2.0, this work focuses on the use of the
rules proposed by Biedermann (1997), these are:
Biedermann Conditional Attribute Association Rule
(BCAAR) and Biedermann Attributional Condition
Association Rule (BACAR).
The first, BCAAR, takes the form (A1 →
A2)C(sup, con f ), where A1, A2 ⊆ M and C ⊆ B. This
rule indicates that every time A1 occurs in the condi-
tions C, then A2 also occurs, with support sup and
confidence conf. The second rule used is BACAR,
which takes the form (C1 → C2)A(sup, con f ), where
C1, C2 ⊆ B and A ⊆ M, that is, every time C1 occurs
for all attributes in A, then for the condition C2, there
Formal Concept Analysis Applied to a Longitudinal Study of COVID-19
151