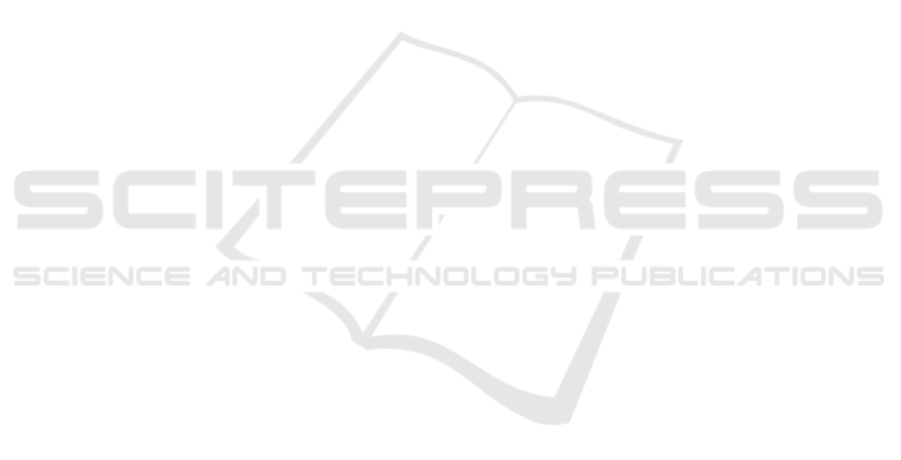
Randolph, J. J. (2009). A guide to writing the dissertation
literature review. Practical Assessment, Research and
Evaluation, 14(13):1–13.
Rojas-Aranda, J. L., Nunez-Varela, J. I., Cuevas-Tello, J. C.,
and Rangel-Ramirez, G. (2020). Fruit classification
for retail stores using deep learning. In Mexican Con-
ference on Pattern Recognition, pages 3–13. Springer.
Rudolph, T., Nagengast, L., Melanie, B., and Bouteiller,
D. (2015). Die Nutzung mobiler Shopping Apps im
Kaufprozess. Marketing Review St. Gallen, 3(32):42–
49.
Sch
¨
utte, R. (2011). Modellierung von handelsinfor-
mationssystemen. Kumulative Habilitationsschrift.
Westf
¨
alische Wilhelms-Universit
¨
at M
¨
unster. M
¨
unster.
Sch
¨
utte, R. (2017). Information systems for retail compa-
nies. In Dubois, E. and Pohl, K., editors, International
Conference on Advanced Information Systems Engi-
neering, pages 13–25, Essen, Deutschland. Springer.
Sch
¨
utte, R. and Vetter, T. (2017). Analyse des digital-
isierungspotentials von handelsunternehmen. In Han-
del 4.0, pages 75–113. Springer.
Singh, P. and Agrawal, V. (2019). A Collaborative Model
for Customer Retention on User Service Experience.
In Bhatia, S. K., Shailesh, T., Mishra, K. K., and Ti-
wari, M. C., editors, Advances in Computer Commu-
nication and Computational Sciences, pages 55–64.
Springer Nature, Singapore, 924 edition.
Suponenkovs, A., Sisojevs, A., Mos
¯
ans, G., Kampars, J.,
Pinka, K., Grabis, J., Locmelis, A., and Taranovs, R.
(2017). Application of image recognition and machine
learning technologies for payment data processing re-
view and challenges. In 5th IEEE Workshop on Ad-
vances in Information, Electronic and Electrical En-
gineering (AIEEE), pages 1–6.
Thornton, C., Hutter, F., Hoos, H. H., and Leyton-Brown,
K. (2013). Auto-weka: Combined selection and
hyperparameter optimization of classification algo-
rithms. Proceedings of the ACM SIGKDD Interna-
tional Conference on Knowledge Discovery and Data
Mining, Part F1288:847–855.
Trinh, H., Fan, Q., Jiyan, P., Gabbur, P., Miyazawa, S., and
Pankanti, S. (2011). Detecting human activities in
retail surveillance using hierarchical finite state ma-
chine. In ICASSP, IEEE International Conference on
Acoustics, Speech and Signal Processing - Proceed-
ings, pages 1337–1340.
Veres, S. M., Molnar, L., Lincoln, N. K., and Morice, C. P.
(2011). Autonomous vehicle control systems - A re-
view of decision making. Proceedings of the Institu-
tion of Mechanical Engineers. Part I: Journal of Sys-
tems and Control Engineering, 225(3):155–195.
Verhoef, P. C., Kannan, P. K., and Inman, J. J. (2015). From
Multi-Channel Retailing to Omni-Channel Retailing.
Introduction to the Special Issue on Multi-Channel
Retailing. Journal of Retailing, 91(2):174–181.
Verhoef, P. C., Lemon, K. N., Parasuraman, A., Roggeveen,
A., Tsiros, M., and Schlesinger, L. A. (2009). Cus-
tomer Experience Creation: Determinants, Dynam-
ics and Management Strategies. Journal of Retailing,
85(1):31–41.
Verstraete, G., Aghezzaf, E. H., and Desmet, B. (2019). A
data-driven framework for predicting weather impact
on high-volume low-margin retail products. Journal
of Retailing and Consumer Services, 48:169–177.
Vinodhini, G. and Chandrasekaran, R. M. (2014). Opinion
mining using principal component analysis based en-
semble model for e-commerce application. CSI Trans-
actions on ICT, 2(3):169–179.
Vom Brocke, J., Simons, A., Niehaves, B., Riemer, K., Plat-
tfaut, R., Cleven, A., Brocke, J. V., and Reimer, K.
(2009). Reconstructing the Giant: On the Importance
of Rigour in Documenting the Literature Search Pro-
cess. In Proceedings of the 17th European Conference
on Information Systems (ECIS ’17), volume 9, page
2206–2217, Verona, Italy.
Wan, M., Ni, J., Misra, R., and McAuley, J. (2020). Ad-
dressing marketing bias in product recommendations.
In WSDM 2020 - Proceedings of the 13th Interna-
tional Conference on Web Search and Data Mining,
pages 618–626.
Wang, L., Fan, H., and Wang, Y. (2018). Site selection of
retail shops based on spatial accessibility and hybrid
bp neural network. ISPRS International Journal of
Geo-Information, 7(6):202.
Weber, F. and Sch
¨
utte, R. (2019). State-of-the-art and
adoption of artificial intelligence in retailing big-
data-analysen im promotion management view project
coming closer to reality-neural correlates of tam view
project digital policy, regulation and governance state-
of-the-art and adop. researchgate.net.
Weber, F. D. and Sch
¨
utte, R. (2019). State-of-the-art and
adoption of artificial intelligence in retailing. Digital
Policy, Regulation and Governance.
Wulfert, T., Betzing, J. H., and Becker, J. (2019). Elic-
iting Customer Preferences for Shopping Companion
Apps: A Service Quality Approach. In Proceedings of
the 14th Internationale Tagung Wirtschaftsinformatik
(WI2019), pages 1220–1234, Siegen, Deutschland.
Xi, N. and Hamari, J. (2019). Vr shopping: A review of
literature. In AMCIS 2019 Proceedings.
Yu, W., Sun, Z., Liu, H., Li, Z., and Zheng, Z. (2018).
Multi-level deep learning based e-commerce product
categorization. In eCOM@ SIGIR.
Zhang, D., Zhou, L., Kehoe, J. L., and Kilic, I. Y. (2016).
What Online Reviewer Behaviors Really Matter? Ef-
fects of Verbal and Nonverbal Behaviors on Detection
of Fake Online Reviews. Journal of Management In-
formation Systems, 33(2):456–481.
A Structured Literature Review on the Application of Machine Learning in Retail
343