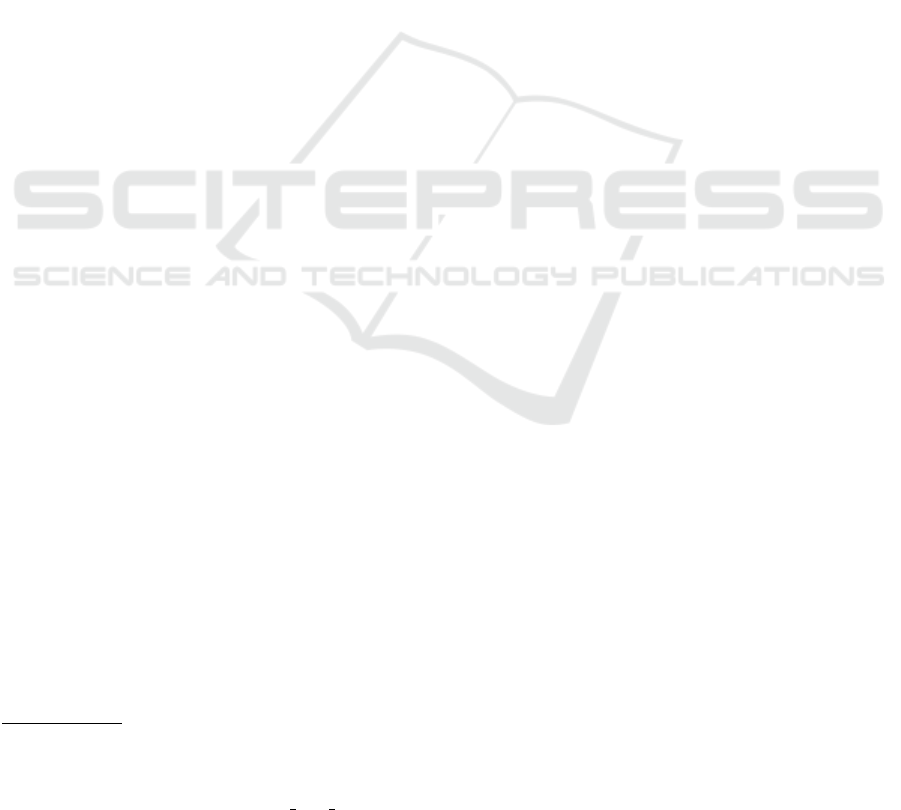
est regulations and protection codes, in contrast to the
need to make data available for scientific research in
critical moments such as the pandemic of COVID-19;
and (ii) to demonstrate, through a use case, that it is
possible to anonymize health records for research and
still track back an individual identification if neces-
sary, with the help of provenance metadata.
The article is organized as follows: In Section 2,
concepts and facts related to the preservation of pri-
vacy are presented to contextualize the reader; in Sec-
tion 3 the main policies and initiatives for research
data are explored; in Section 4, health systems in
Brazil are addressed as well as examples of actions
aimed at making clinical data available for research;
in Section 5, a use case of randomly generated health
records is discussed; and, finally, the last section con-
cludes the paper.
2 BACKGROUND
Advances in automation and digitization of health
systems have brought agility in service, and facili-
tated the retrieval of information and exams, but, on
the other hand, they have also made patient data more
exposed to privacy violations and security breaches.
It is necessary to point out that data security and pri-
vacy are two related concepts, but they should not be
confused. Security refers to aspects of protecting a
system from unauthorized use, including user authen-
tication, information encryption, access control, fire-
wall policies, and intrusion detection. Privacy refers
to ensuring those entitled to control over the avail-
ability and use of their data, through data governance
mechanisms.
To understand policies and initiatives to provide
data security and preserve privacy, it is also neces-
sary to know the already consolidated legislation. The
Health Insurance Portability and Accountability Act
(HIPAA
3
) in USA, the General Data Protection Regu-
lation (GDPR
4
) in Europe, and the General Data Pro-
tection Law (Lei Geral de Protec¸
˜
ao de Dados LGPD
5
,
in portuguese) in Brazil define many requirements
and qualify possible penalties for cases of their viola-
tion. Among these requirements there are guarantees
such as the right to be forgotten (data exclusion) and
the need to collect user consent for the use of their
data (Ferreira, 2020).
3
https://www.hhs.gov/hipaa/for-professionals/index.ht
ml
4
https://gdprinfo.eu/
5
http://www.planalto.gov.br/ccivil\ 03/\ ato2015-201
8/2018/lei/l13709.htm
Pioneeringly, the HIPAA regulation has defined,
in a comprehensive manner, which protective mea-
sures should be used to treat data related to indi-
vidual clinical data. The aforementioned regulation
also defines a group of 18 sensitive attributes con-
sidered identifiers, which may uniquely identify an
individual. These attributes are known as Protected
Health Information (PHI), such as name, date, regis-
tration numbers, IP addresses, photos, and biometric
data, among other demographic information. More
recently, GDPR and LGPD defined the set of personal
data that can lead to the identification of a particular
individual, directly or indirectly. There are also at-
tributes that are classified as semi-identifiers (Brito
and Machado, 2017), such as race, age, schooling,
among others, which may indirectly identify an indi-
vidual when combined with external information. Al-
though there are already many anonymization tools
available to cope with these law requirements and
minimize the risks of identification, these tools still
need to be improved (Carvalho et al., 2020).
On the other hand, according to GDPR Art. 9,
items (h) and (i), and LGPD Art. 7, item (IV ), and
Art. 13, data used for health research and academic
activities are exempt from consent collection. Ad-
ditionally, as a result of the waiving of consent for
research purpose, projects are also exempt from pro-
viding guarantees of data exclusion, since, in prin-
ciple, the data used in research may remain per-
petually available for reuse. Therefore, while pre-
processing personal data using anonymization tech-
niques is strongly recommended, an important re-
quirement for the pre-processing tools is to apply
anonymization in such a way that it should be possi-
ble to have access to the original data when requested
by a restricted group of researchers.
Nowadays, there are different types of anonymiza-
tion techniques, which are usually applied to identi-
fier attributes. It is worth mentioning the technique
known as pseudoanonymization. It consists of any
process of transformation of personal data, carried
out in such a way that these data cannot be associ-
ated with the individual without the use of additional
data, which must be kept separately. It is a process
of desidentification that removes or replaces identi-
fying attributes such as names and identification keys
(IDs) of a given dataset but keeping in a separate place
the data that can directly identify the individual. In
pseudoanonymization, different ids must be used for
each existing domain, such as research, administra-
tive or medical. In this way, the possibility of re-
identification of a given patient is guaranteed when
necessary and by duly authorized persons.
On the other hand, for semi-identifier attributes,
ICEIS 2022 - 24th International Conference on Enterprise Information Systems
318