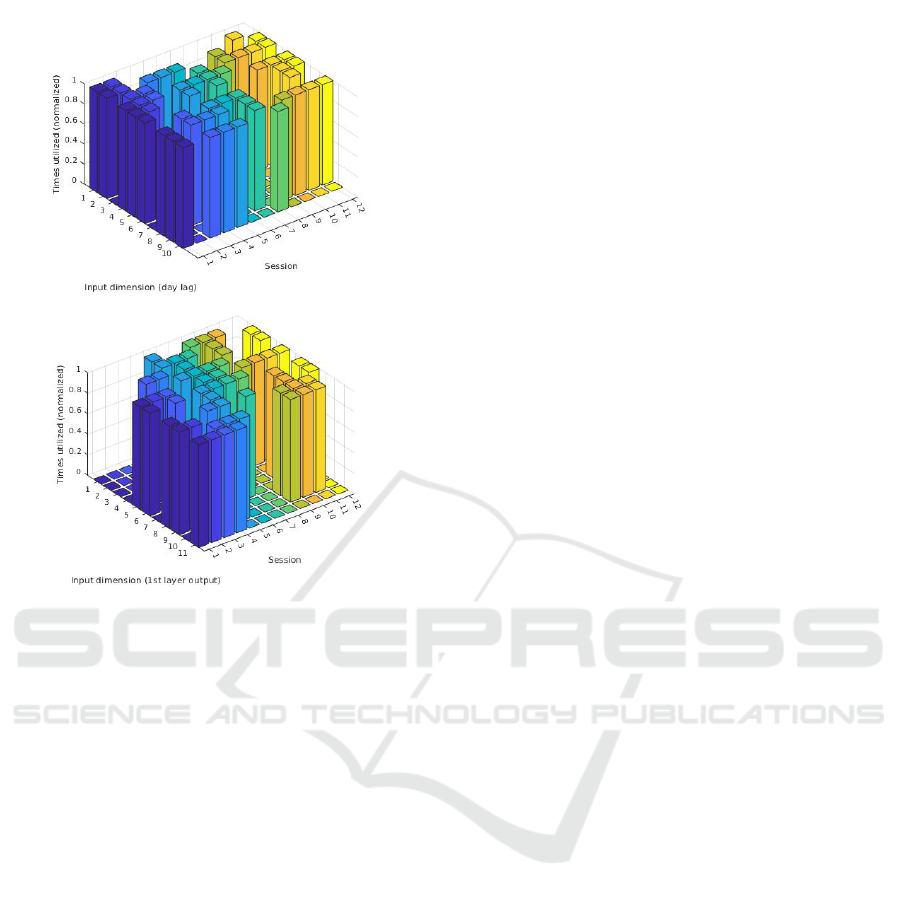
Figure 4: Non-zero channels generated by the KGates per
session. Top - by the 1
st
, bottom - by the 2
nd
KGate.
REFERENCES
Akashi, S. (2001). Application of ε-entropy theory to
kolmogorov—arnold representation theorem. Reports
on Mathematical Physics, 48(1):19–26. Proceedings
of the XXXII SYMPOSIUM ON MATHEMATICAL
PHYSICS.
Akter, M. and Jakaite, L. (2019). Extraction of texture fea-
tures from x-ray images: Case of osteoarthritis detec-
tion. In Yang, X.-S., Sherratt, S., Dey, N., and Joshi,
A., editors, Third International Congress on Informa-
tion and Communication Technology, pages 143–150,
Singapore. Springer Singapore.
Beheim, L., Zitouni, A., Belloir, F., and de la Housse, C.
d. M. (2004). New rbf neural network classifier with
optimized hidden neurons number. WSEAS Transac-
tions on Systems, (2):467–472.
Bender, E. M., Gebru, T., McMillan-Major, A., and
Shmitchell, S. (2021). On the dangers of stochastic
parrots: Can language models be too big? In Pro-
ceedings of the 2021 ACM Conference on Fairness,
Accountability, and Transparency, FAccT ’21, page
610–623, New York, NY, USA. Association for Com-
puting Machinery.
Blodgett, S. L. and Madaio, M. (2021). Risks of AI foun-
dation models in education. CoRR, abs/2110.10024.
Bommasani, R., Hudson, D. A., Adeli, E., Altman, R.,
Arora, S., von Arx, S., Bernstein, M. S., Bohg, J.,
Bosselut, A., Brunskill, E., Brynjolfsson, E., Buch,
S., Card, D., Castellon, R., Chatterji, N. S., Chen,
A. S., Creel, K., Davis, J. Q., Demszky, D., Don-
ahue, C., Doumbouya, M., Durmus, E., Ermon, S.,
Etchemendy, J., Ethayarajh, K., Fei-Fei, L., Finn, C.,
Gale, T., Gillespie, L., Goel, K., Goodman, N. D.,
Grossman, S., Guha, N., Hashimoto, T., Henderson,
P., Hewitt, J., Ho, D. E., Hong, J., Hsu, K., Huang,
J., Icard, T., Jain, S., Jurafsky, D., Kalluri, P., Karam-
cheti, S., Keeling, G., Khani, F., Khattab, O., Koh,
P. W., Krass, M. S., Krishna, R., Kuditipudi, R., and
et al. (2021). On the opportunities and risks of foun-
dation models. CoRR, abs/2108.07258.
Broomhead, D. S. and Lowe, D. (1988). Radial basis
functions, multi-variable functional interpolation and
adaptive networks. Technical report, Royal Signals
and Radar Establishment Malvern (United Kingdom).
Brown, T. B., Mann, B., Ryder, N., Subbiah, M., Kaplan, J.,
Dhariwal, P., Neelakantan, A., Shyam, P., Sastry, G.,
Askell, A., Agarwal, S., Herbert-Voss, A., Krueger,
G., Henighan, T., Child, R., Ramesh, A., Ziegler,
D. M., Wu, J., Winter, C., Hesse, C., Chen, M., Sigler,
E., Litwin, M., Gray, S., Chess, B., Clark, J., Berner,
C., McCandlish, S., Radford, A., Sutskever, I., and
Amodei, D. (2020). Language models are few-shot
learners.
Cho, K., van Merrienboer, B., G
¨
ulc¸ehre, C¸ ., Bougares, F.,
Schwenk, H., and Bengio, Y. (2014). Learning phrase
representations using RNN encoder-decoder for sta-
tistical machine translation. CoRR, abs/1406.1078.
Ciemny Marcin, S. S. (2022). Gmdh-type neural networks
for predicting financial time series: A study of infor-
mational efficiency of stock markets. In Advances
in Systems Engineering. Proceedings of the 28th In-
ternational Conference on Systems Engineering, IC-
SEng 2021, December 14-16, Wrocław, Poland. Lec-
ture Notes in Networks and Systems, volume 364,
pages 141–150. Springer International Publishing.
Dauphin, Y. and Grangier, D. (2016). Predicting dis-
tributions with linearizing belief networks. CoRR,
abs/1511.05622.
Dauphin, Y. N., Fan, A., Auli, M., and Grangier, D.
(2016). Language modeling with gated convolutional
networks. CoRR, abs/1612.08083.
Farlow, S. J. (1981). The gmdh algorithm of ivakhnenko.
The American Statistician, 35(4):210–215.
Fedus, W., Zoph, B., and Shazeer, N. (2021). Switch trans-
formers: Scaling to trillion parameter models with
simple and efficient sparsity. CoRR, abs/2101.03961.
Field, H. (2021). At Stanford’s “foundation models” work-
shop, large language model debate resurfaces. Morn-
ing Brew.
Girosi, F. and Poggio, T. (1989). Representation proper-
ties of networks: Kolmogorov’s theorem is irrelevant.
Neural Computation, 1(4):465–469.
Hilbert, D. (1902). Mathematical problems. Bulletin of the
American Mathematical Society, 8(10):437–479.
Hinton, G. E., Srivastava, N., Krizhevsky, A., Sutskever,
I., and Salakhutdinov, R. (2012). Improving neural
networks by preventing co-adaptation of feature de-
tectors. CoRR, abs/1207.0580.
ICEIS 2022 - 24th International Conference on Enterprise Information Systems
498