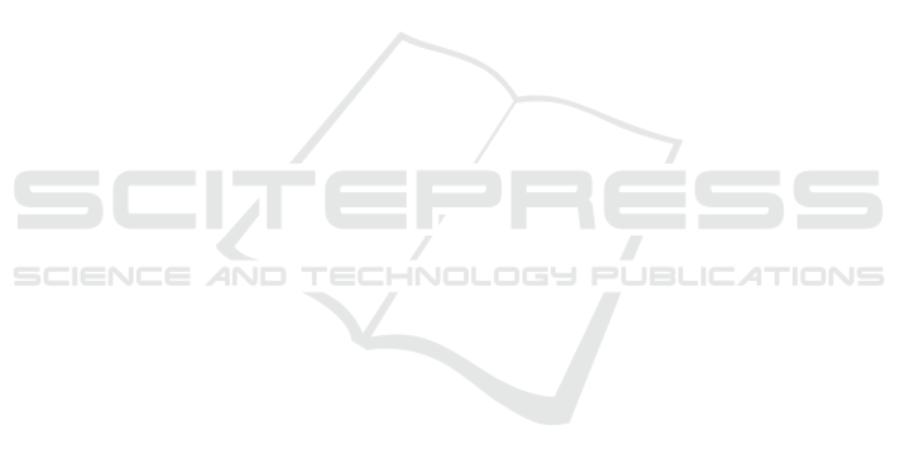
REFERENCES
3GPP (2016). Study on enhancement of 3gpp support for
5g v2x services (release 15). Tech. Report TR, 22.
Ahmed, L., Ahmad, K., Said, N., Qolomany, B., Qadir, J.,
and Al-Fuqaha, A. (2020). Active learning based fed-
erated learning for waste and natural disaster image
classification. IEEE Access, 8:208518–208531.
Barbieri, L., Savazzi, S., and Nicoli, M. (2021). Decen-
tralized federated learning for road user classifica-
tion in enhanced v2x networks. In 2021 IEEE Inter-
national Conference on Communications Workshops
(ICC Workshops), pages 1–6. IEEE.
Boban, M., Kousaridas, A., Manolakis, K., Eichinger, J.,
and Xu, W. (2018). Connected roads of the future:
Use cases, requirements, and design considerations
for vehicle-to-everything communications. IEEE ve-
hicular technology magazine, 13(3):110–123.
Boualouache, A. and Engel, T. (2021). Federated learning-
based scheme for detecting passive mobile attackers
in 5g vehicular edge computing. Annals of Telecom-
munications, pages 1–20.
Brendan McMahan, Daniel Ramage, R. S. (2017). Feder-
ated learning: Collaborative machine learning without
centralized training data.
Dickson, B. (2020). Why deep learning won’t give us level
5 self-driving cars.
Ding, A. Y., Peltonen, E., Meuser, T., Aral, A., Becker,
C., Dustdar, S., Hiessl, T., Kranzlmuller, D., Liyan-
age, M., Magshudi, S., et al. (2021). Roadmap
for edge ai: A dagstuhl perspective. arXiv preprint
arXiv:2112.00616.
Du, Z., Wu, C., Yoshinaga, T., Yau, K.-L. A., Ji, Y., and Li,
J. (2020). Federated learning for vehicular internet of
things: Recent advances and open issues. IEEE Open
Journal of the Computer Society, 1:45–61.
Elbir, A. M., Soner, B., and Coleri, S. (2020). Feder-
ated learning in vehicular networks. arXiv preprint
arXiv:2006.01412.
ETSI (2019). Etsi tr 103 562.
Fukatsu, R. and Sakaguchi, K. (2019). Millimeter-wave v2v
communications with cooperative perception for auto-
mated driving. In 2019 IEEE 89th Vehicular Technol-
ogy Conference (VTC2019-Spring), pages 1–5. IEEE.
Kone
ˇ
cn
`
y, J., McMahan, H. B., Yu, F. X., Richt
´
arik, P.,
Suresh, A. T., and Bacon, D. (2016). Federated learn-
ing: Strategies for improving communication effi-
ciency. arXiv preprint arXiv:1610.05492.
L
¨
offler, R. (2021). How many cars are in the world?
Li, T., Sahu, A. K., Talwalkar, A., and Smith, V. (2020).
Federated learning: Challenges, methods, and fu-
ture directions. IEEE Signal Processing Magazine,
37(3):50–60.
Meuser, T. (2020). Data management in vehicular
networks-relevance-aware networking for advanced
driver assistance systems.
Nasr, M., Shokri, R., and Houmansadr, A. (2019). Compre-
hensive privacy analysis of deep learning: Passive and
active white-box inference attacks against centralized
and federated learning. In 2019 IEEE symposium on
security and privacy (SP), pages 739–753. IEEE.
NEIL, C., THOMPSON, K., GREENEWALD, K., LEE, G.,
and MANSO, F. (2021). Deep learning’s diminishing
returns.
Nguyen, A., Do, T., Tran, M., Nguyen, B. X., Duong, C.,
Phan, T., Tjiputra, E., and Tran, Q. D. (2021). Deep
federated learning for autonomous driving. arXiv
preprint arXiv:2110.05754.
Otoum, S., Al Ridhawi, I., and Mouftah, H. T. (2020).
Blockchain-supported federated learning for trustwor-
thy vehicular networks. In GLOBECOM 2020-2020
IEEE Global Communications Conference, pages 1–
6. IEEE.
Pilz, C., Ulbel, A., and Steinbauer-Wagner, G. (2021). The
components of cooperative perception-a proposal for
future works. In 2021 IEEE International Intelligent
Transportation Systems Conference (ITSC), pages 7–
14. IEEE.
Shan, M., Narula, K., Wong, Y. F., Worrall, S., Khan, M.,
Alexander, P., and Nebot, E. (2021). Demonstrations
of cooperative perception: safety and robustness in
connected and automated vehicle operations. Sensors,
21(1):200.
Sjoberg, K., Andres, P., Buburuzan, T., and Brakemeier, A.
(2017). Cooperative intelligent transport systems in
europe: Current deployment status and outlook. IEEE
Vehicular Technology Magazine, 12(2):89–97.
Vyas, J., Das, D., and Das, S. K. (2020). Vehicular edge
computing based driver recommendation system us-
ing federated learning. In 2020 IEEE 17th Interna-
tional Conference on Mobile Ad Hoc and Sensor Sys-
tems (MASS), pages 675–683. IEEE.
Yu, Z., Hu, J., Min, G., Xu, H., and Mills, J. (2020). Proac-
tive content caching for internet-of-vehicles based on
peer-to-peer federated learning. In 2020 IEEE 26th
International Conference on Parallel and Distributed
Systems (ICPADS), pages 601–608. IEEE.
Yuan, X., Chen, J., Zhang, N., Fang, X., and Liu, D. (2021).
A federated bidirectional connection broad learning
scheme for secure data sharing in internet of vehicles.
China Communications, 18(7):117–133.
Zhao, L., Ran, Y., Wang, H., Wang, J., and Luo, J. (2021).
Towards cooperative caching for vehicular networks
with multi-level federated reinforcement learning. In
ICC 2021-IEEE International Conference on Commu-
nications, pages 1–6. IEEE.
VEHITS 2022 - 8th International Conference on Vehicle Technology and Intelligent Transport Systems
352