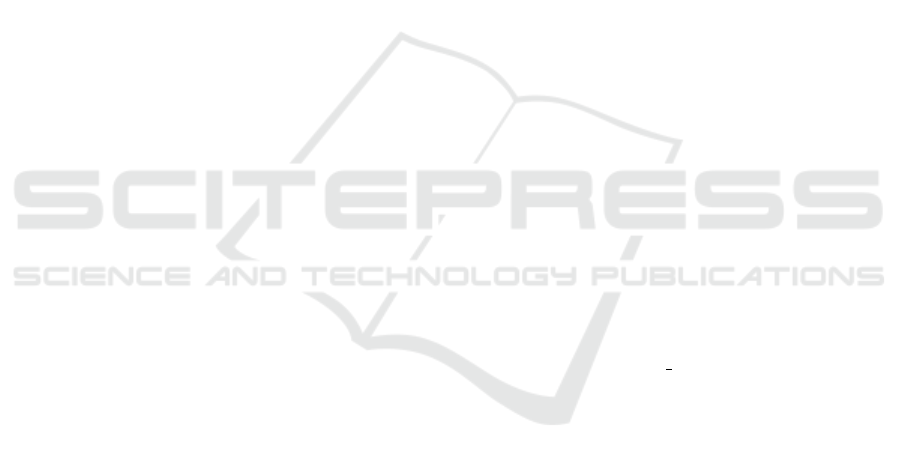
ing. Given the present-day interest and importance of
the topics discussed here, we expect that the proposed
research attracts top computer science and engineer-
ing students. Importantly, the public and private in-
terest in AI, big data, machine learning and model-
ing and simulation, not to speak of forest and wildfire
management, justifies adequate funding for research
and development, while also stimulating innovation
and the creation of value in related areas.
ACKNOWLEDGEMENTS
This work is supported by Fundac¸
˜
ao para a Ci
ˆ
encia
e a Tecnologia under Grant No.: UIDB/04111/2020
(COPELABS)
REFERENCES
Achtemeier, G. L. (2013). Field validation of a free-
agent cellular automata model of fire spread with fire–
atmosphere coupling. International Journal of Wild-
land Fire, 22(2):148–156.
Cardil, A., Monedero, S., Ram
´
ırez, J., and Silva, C. A.
(2019). Assessing and reinitializing wildland fire sim-
ulations through satellite active fire data. Journal of
Environmental Management, 231:996–1003.
Catry, F. X., Rego, F. C., Bac¸
˜
ao, F. L., and Moreira, F.
(2010). Modeling and mapping wildfire ignition risk
in portugal. International Journal of Wildland Fire,
18(8):921–931.
Coakley, S., Gheorghe, M., Holcombe, M., Chin, S., Worth,
D., and Greenough, C. (2012). Exploitation of high
performance computing in the FLAME agent-based
simulation framework. In Proc. of the 14th Int. Conf.
on High Performance Computing and Communica-
tions, pages 538–545, Liverpool, UK. IEEE.
Collins, R. D., de Neufville, R., Claro, J., Oliveira, T., and
Pacheco, A. P. (2013). Forest fire management to
avoid unintended consequences: A case study of Por-
tugal using system dynamics. Journal of Environmen-
tal Management, 130:1–9.
Costa, R. L. C., Miranda, E., Dias, P., and Moreira, J.
(2021). Experience: Quality assessment and improve-
ment on a forest fire dataset. Journal of Data and In-
formation Quality, 13(1):1–13.
David, N., Fachada, N., and Rosa, A. C. (2017). Verifying
and Validating Simulations, pages 173–204. Springer
International Publishing, Cham.
Denham, M., Wendt, K., Bianchini, G., Cort
´
es, A., and
Margalef, T. (2012). Dynamic data-driven genetic al-
gorithm for forest fire spread prediction. Journal of
Computational Science, 3(5):398–404.
Fachada, N., Figueiredo, M. A. T., Lopes, V. V., Martins,
R. C., and Rosa, A. C. (2014). Spectrometric differ-
entiation of yeast strains using minimum volume in-
crease and minimum direction change clustering cri-
teria. Pattern Recognition Letters, 45:55–61.
Fachada, N., Lopes, V. V., Martins, R. C., and Rosa, A. C.
(2015). Towards a standard model for research in
agent-based modeling and simulation. PeerJ Com-
puter Science, 1:e36.
Fachada, N., Lopes, V. V., Martins, R. C., and Rosa, A. C.
(2017a). cf4ocl: a C framework for OpenCL. Science
of Computer Programming, 143:9–19.
Fachada, N., Lopes, V. V., Martins, R. C., and Rosa, A. C.
(2017b). Model-independent comparison of simula-
tion output. Simulation Modelling Practice and The-
ory, 72:131–149.
Fachada, N., Lopes, V. V., Martins, R. C., and Rosa, A. C.
(2017c). Parallelization strategies for spatial agent-
based models. International Journal of Parallel Pro-
gramming, 45(3):449–481.
Fachada, N., Lopes, V. V., and Rosa, A. (2009). Simulating
antigenic drift and shift in influenza A. In Proc. of the
2009 ACM symposium on Applied Computing, SAC
’09, pages 2093–2100, New York, NY, USA. ACM.
Fachada, N., Rodrigues, J., Lopes, V. V., Martins, R. C.,
and Rosa, A. C. (2016). micompr: An R package for
multivariate independent comparison of observations.
The R Journal, 8(2):405–420.
Fachada, N. and Rosa, A. (2018). micompm: A MAT-
LAB/Octave toolbox for multivariate independent
comparison of observations. Journal of Open Source
Software, 3:430.
Fachada, N. and Rosa, A. C. (2017). Assessing the feasibil-
ity of OpenCL CPU implementations for agent-based
simulations. In Proceedings of the 5th International
Workshop on OpenCL, IWOCL 2017, pages 4:1–4:10,
New York, NY, USA. ACM.
Fachada, V., Fachada, N., Turpeinen, T., Rahkila, P., Rosa,
A., and Kainulainen, H. (2012). Topocell – an image
analysis tool to study intracellular topography. The
FASEB Journal, 26(1
supplement):578.2–578.2.
Fernandes, C. M., Fachada, N., Laredo, J. L. J., Merelo, J.-
J., and Rosa, A. C. (2020). Population sizing of cellu-
lar evolutionary algorithms. Swarm and Evolutionary
Computation, 58:100721.
Fernandes, C. M., Fachada, N., Merelo, J.-J., and Rosa,
A. C. (2019). Steady state particle swarm. PeerJ Com-
puter Science, 5:e202.
Fernandes, P. M. A., In
´
acio, P. M. A., Feliciano, H., and
Fachada, N. (2022). SimpAI: Evolutionary heuristics
for the ColorShapeLinks board game competition. In
Barbedo, I., Barroso, B., Leger
´
en, B., Roque, L., and
Sousa, J. P., editors, Videogame Sciences and Arts, VJ
2020, volume 1531 of Communications in Computer
and Information Science, chapter 9, pages 113–126.
Springer International Publishing, Cham.
G
´
omez-Gonz
´
alez, S., Ojeda, F., and Fernandes, P. M.
(2018). Portugal and chile: Longing for sustainable
forestry while rising from the ashes. Environmental
Science & Policy, 81:104–107.
Han, A., Qing, S., Bao, Y., Na, L., Bao, Y., Liu, X., Zhang,
J., and Wang, C. (2021). Short-term effects of fire
A Computational Pipeline for Modeling and Predicting Wildfire Behavior
83