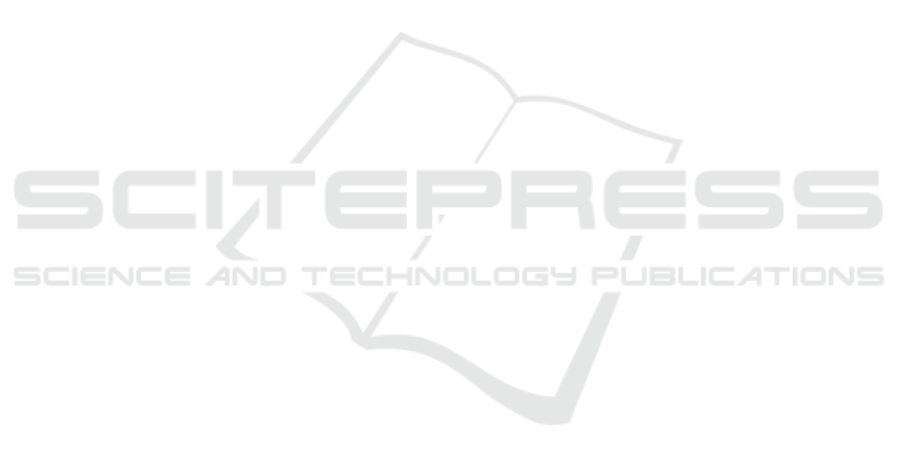
sponsible for generating a sentence and keep improv-
ing our dataset with better translations. We hope that
this work will encourage future works on image cap-
tioning for the Portuguese language.
ACKNOWLEDGEMENTS
Authors would like to thank FAPESB TIC PROJECT
Number TIC0002/2015, CAPES Finantial Code 001
and CNPQ for financial support.
REFERENCES
Bahdanau, D., Cho, K., and Bengio, Y. (2016). Neural ma-
chine translation by jointly learning to align and trans-
late.
Bender, E. (2019). English isn’t generic for language, de-
spite what nlp papers might lead you to believe. In
Symposium and Data Science and Statistics. [Online;
accessed 15-may-2020].
Bender, E. M. (2009). Linguistically na
¨
ıve != language in-
dependent: Why NLP needs linguistic typology. In
Proceedings of the EACL 2009 Workshop on the In-
teraction between Linguistics and Computational Lin-
guistics: Virtuous, Vicious or Vacuous?, pages 26–32,
Athens, Greece. Association for Computational Lin-
guistics.
Bernardi, R., Cakici, R., Elliott, D., Erdem, A., Erdem, E.,
Ikizler-Cinbis, N., Keller, F., Muscat, A., and Plank,
B. (2017). Automatic description generation from im-
ages: A survey of models, datasets, and evaluation
measures.
Cho, K., van Merrienboer, B., Gulcehre, C., Bahdanau, D.,
Bougares, F., Schwenk, H., and Bengio, Y. (2014).
Learning phrase representations using rnn encoder-
decoder for statistical machine translation.
dos Santos, G. O., Colombini, E. L., and Avila, S. (2021).
#pracegover: A large dataset for image captioning in
portuguese.
Hodosh, M., Young, P., and Hockenmaier, J. (2013). Fram-
ing image description as a ranking task: Data, models
and evaluation metrics. Journal of Artificial Intelli-
gence Research, 47:853–899.
Huang, L., Wang, W., Chen, J., and Wei, X.-Y. (2019). At-
tention on attention for image captioning.
Klein, G., Kim, Y., Deng, Y., Senellart, J., and Rush,
A. (2017). OpenNMT: Open-source toolkit for neu-
ral machine translation. In Proceedings of ACL
2017, System Demonstrations, pages 67–72, Vancou-
ver, Canada. Association for Computational Linguis-
tics.
Lavie, A. and Agarwal, A. (2007). Meteor: An automatic
metric for mt evaluation with high levels of correlation
with human judgments. In Proceedings of the Second
Workshop on Statistical Machine Translation, StatMT
’07, page 228–231, USA. Association for Computa-
tional Linguistics.
Li, X., Yin, X., Li, C., Zhang, P., Hu, X., Zhang, L., Wang,
L., Hu, H., Dong, L., Wei, F., Choi, Y., and Gao, J.
(2020). Oscar: Object-semantics aligned pre-training
for vision-language tasks.
Lin, T.-Y., Maire, M., Belongie, S., Bourdev, L., Girshick,
R., Hays, J., Perona, P., Ramanan, D., Zitnick, C. L.,
and Doll
´
ar, P. (2015). Microsoft coco: Common ob-
jects in context.
Lu, J., Xiong, C., Parikh, D., and Socher, R. (2017). Know-
ing when to look: Adaptive attention via a visual sen-
tinel for image captioning.
Papineni, K., Roukos, S., Ward, T., and Zhu, W.-J. (2002).
Bleu: A method for automatic evaluation of ma-
chine translation. In Proceedings of the 40th Annual
Meeting on Association for Computational Linguis-
tics, ACL ’02, page 311–318, USA. Association for
Computational Linguistics.
Pennington, J., Socher, R., and Manning, C. D. (2014).
Glove: Global vectors for word representation. In
Empirical Methods in Natural Language Processing
(EMNLP), pages 1532–1543.
Simonyan, K. and Zisserman, A. (2015). Very deep convo-
lutional networks for large-scale image recognition.
Socher, R. and Fei-Fei, L. (2010). Connecting modalities:
Semi-supervised segmentation and annotation of im-
ages using unaligned text corpora. In 2010 IEEE Com-
puter Society Conference on Computer Vision and
Pattern Recognition, pages 966–973.
Szegedy, C., Liu, W., Jia, Y., Sermanet, P., Reed, S.,
Anguelov, D., Erhan, D., Vanhoucke, V., and Rabi-
novich, A. (2014). Going deeper with convolutions.
Tan, M. and Le, Q. V. (2020). Efficientnet: Rethinking
model scaling for convolutional neural networks.
Vinyals, O., Toshev, A., Bengio, S., and Erhan, D. (2015).
Show and tell: A neural image caption generator.
Xu, K., Ba, J., Kiros, R., Cho, K., Courville, A., Salakhut-
dinov, R., Zemel, R., and Bengio, Y. (2016). Show,
attend and tell: Neural image caption generation with
visual attention.
Yao, B. Z., Yang, X., Lin, L., Lee, M. W., and Zhu, S.-C.
(2010). I2t: Image parsing to text description. Pro-
ceedings of the IEEE, 98(8):1485–1508.
Young, P., Lai, A., Hodosh, M., and Hockenmaier, J.
(2014). From image descriptions to visual denota-
tions: New similarity metrics for semantic inference
over event descriptions. TACL, 2:67–78.
Towards Image Captioning for the Portuguese Language: Evaluation on a Translated Dataset
393