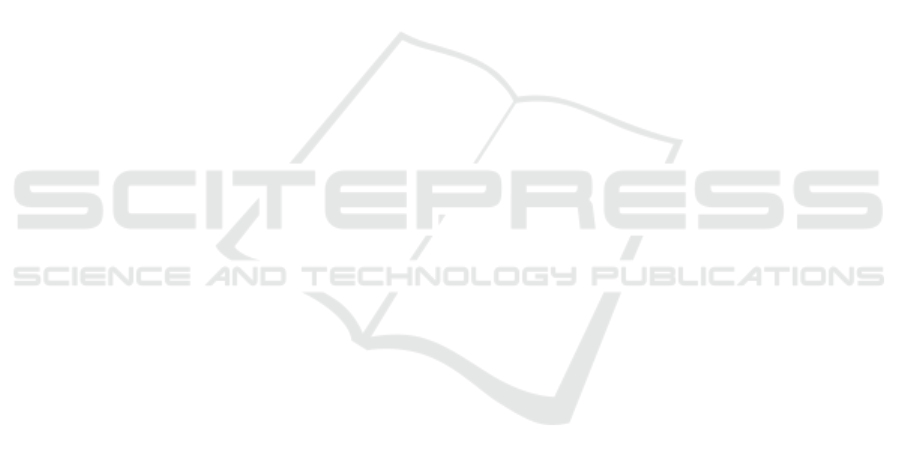
nearby locations to estimate the value of virtual sen-
sors.
ACKNOWLEDGEMENT
This work was financed in part by the Coordenac¸
˜
ao
de Aperfeic¸oamento de Pessoal de N
´
ıvel Superior -
Brasil (CAPES) – Finance Code 001. Also, this work
was partially supported by Conselho Nacional de
Desenvolvimento Cient
´
ıfico e Tecnol
´
ogico – CNPq
– 313111/2019-7. This work also received fund-
ing from S
˜
ao Paulo Research Foundation (FAPESP)
– 2018/23092-1, 2020/05183-0, 2020/05115-4; and
Rio Grande do Sul Research Foundation (FAPERGS)
– 19/2551-0001266-7, 19/2551-0001224-1, 19/2551-
0001689-1, 21/2551-0000688-9.
REFERENCES
Asy’ari, M. K., Musyafa’, A., Noriyati, R. D., and Indri-
awati, K. (2019). Soft sensor design of solar irra-
diance using multiple linear regression. In 2019 In-
ternational Seminar on Intelligent Technology and Its
Applications (ISITIA), pages 56–60.
Chew, L. P. (1989). Constrained delaunay triangulations.
Algorithmica, 4(1-4):97–108.
Cristaldi, L., Ferrero, A., Macchi, M., Mehrafshan, A., and
Arpaia, P. (2020). Virtual sensors: a tool to improve
reliability. In 2020 IEEE International Workshop on
Metrology for Industry 4.0 IoT, pages 142–145.
Erturk, M. A. and Vollero, L. (2020). Gsp for virtual sen-
sors in ehealth applications. In 2020 IEEE 44th An-
nual Computers, Software, and Applications Confer-
ence (COMPSAC), pages 1683–1688.
Fanti, M. P., Mangini, A. M., Roccotelli, M., Nolich, M.,
and Ukovich, W. (2018). Modeling virtual sensors for
electric vehicles charge services. In 2018 IEEE Inter-
national Conference on Systems, Man, and Cybernet-
ics (SMC), pages 3853–3858.
Fix, E. and Hodges, J. L. (1989). Discriminatory analy-
sis. nonparametric discrimination: Consistency prop-
erties. International Statistical Review/Revue Interna-
tionale de Statistique, 57(3):238–247.
Flores, H., Hui, P., Tarkoma, S., Li, Y., Anagnostopoulos,
T., Kostakos, V., Luo, C., and Su, X. (2018). Sen-
sorclone: A framework for harnessing smart devices
with virtual sensors. In Proceedings of the 9th ACM
Multimedia Systems Conference, MMSys ’18, page
328–338, New York, NY, USA. Association for Com-
puting Machinery.
Franke, R. (1982). Scattered data interpolation: tests
of some methods. Mathematics of computation,
38(157):181–200.
Gupta, A. and Mukherjee, N. (2016). Rationale behind the
virtual sensors and their applications. In 2016 Interna-
tional Conference on Advances in Computing, Com-
munications and Informatics (ICACCI), pages 1608–
1614.
Ilyas, E. B., Fischer, M., Iggena, T., and T
¨
onjes, R. (2020).
Virtual sensor creation to replace faulty sensors us-
ing automated machine learning techniques. In 2020
Global Internet of Things Summit (GIoTS), pages 1–6.
Liu, L., Kuo, S. M., and Zhou, M. (2009). Virtual sensing
techniques and their applications. In 2009 Interna-
tional Conference on Networking, Sensing and Con-
trol, pages 31–36.
Mahmoudi, C., Mourlin, F., and Battou, A. (2018). Formal
definition of edge computing: An emphasis on mo-
bile cloud and iot composition. In 2018 Third Inter-
national Conference on Fog and Mobile Edge Com-
puting (FMEC), pages 34–42.
Memon, M. H., Kumar, W., Memon, A., Chowdhry, B. S.,
Aamir, M., and Kumar, P. (2016). Internet of things
(iot) enabled smart animal farm. In 2016 3rd Inter-
national Conference on Computing for Sustainable
Global Development (INDIACom), pages 2067–2072.
Pait, F. M. (2018). The barycenter method for direct opti-
mization. arXiv preprint arXiv:1801.10533.
Shao, W., Chen, S., and Harris, C. J. (2018). Adaptive soft
sensor development for multi-output industrial pro-
cesses based on selective ensemble learning. IEEE
Access, 6:55628–55642.
S
´
anchez-Molina, J., Rodr
´
ıguez, F., Guzm
´
an, J., and
Ram
´
ırez-Arias, J. (2015). Water content virtual sen-
sor for tomatoes in coconut coir substrate for irriga-
tion control design. Agricultural Water Management,
151:114–125. New proposals in the automation and
remote control of water management in agriculture:
agromotic systems.
Sutarya, D. and Mahendra, A. (2015). Virtual sensor for
time series prediction of hydrogen safety parameter
in degussa sintering furnace. In 2015 2nd Interna-
tional Conference on Information Technology, Com-
puter, and Electrical Engineering (ICITACEE), pages
81–86.
Tong, W. and Zewen, D. (2017). Soft sensor modeling
method of dynamic liquid level based on improved ks
algorithm. In 2017 29th Chinese Control And Deci-
sion Conference (CCDC), pages 6510–6515.
Wang, Z., Zhao, Z., Li, D., and Cui, L. (2015). Data-
driven soft sensor modeling for algal blooms moni-
toring. IEEE Sensors Journal, 15(1):579–590.
Yuan, X., Zhou, J., Huang, B., Wang, Y., Yang, C., and
Gui, W. (2020). Hierarchical quality-relevant feature
representation for soft sensor modeling: A novel deep
learning strategy. IEEE Transactions on Industrial In-
formatics, 16(6):3721–3730.
Zhang, M.-Z., Wang, L.-M., and Xiong, S.-M. (2020). Us-
ing machine learning methods to provision virtual sen-
sors in sensor-cloud. Sensors, 20(7).
Multivariate Interpolation at the Edge to Infer Faulty IoT Sensor Metrics
287