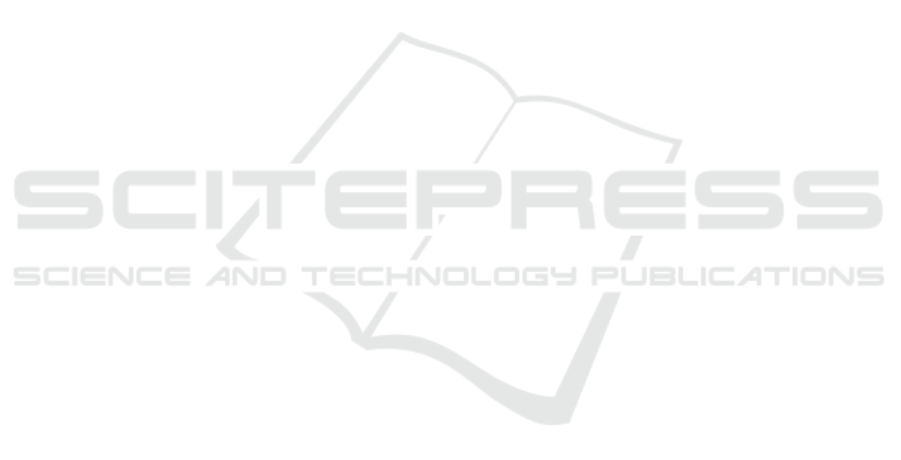
skills and knowledge of understanding and processing
health-related information, the higher the willingness
to use it(Griebel et al., 2018).
Based on the results of this study no statistically
effect could be observed for mHealth apps. The third
research question focused on which user groups can
be formed on the basis of socio-deomgraphic data.
Thus, in this work, a user group was determined in
terms of content on the basis of the positive correla-
tions between two variables, and conversely, another
user group from the negative correlations. The fol-
lowing different characteristics emerged: one group
consists of young, low-educated, however healthy and
app-experienced participants who are willing to use
health apps. The other group consists of older par-
ticipants with good health, little experience in using
mhealth apps however with a higher educational at-
tainment. This group has a low intention to use apps.
Both groups differ significantly from each other. Age
is to be regarded as the decisive factor that influences
all other factors. The older the participants, the less
experience they have in using apps (Searcy et al.,
2019) and expertise in using digital ICT in the health
sector
(van der Vaart and Drossaert, 2017), which are rel-
evant for the acceptance of health apps. Due to their
diversity, the different types of users require techni-
cal and content solutions that are specially adapted to
them and contribute to increasing acceptance.
6 LIMITATION AND OUTLOOK
The findings from the present study have provided in-
sights into acceptance-relevant factors regarding the
intention to use mhealth apps. However, the context
of our study was limited. First of all the study was
mainly conducted in the students surrounding at uni-
versity which resulted in a rather young sample. Even
though results could be detected for exactly this type
of user group, further studies should focus on broader
samples to present a representative whole. Moreover,
this study only evaluated an associated mhealth app.
In order to receive real data in terms of hand-on-
experience, it is necessary to develop a mhealth app
with different features and evaluate the usability with
regard to acceptance-relevant factors. Furthermore,
the app area of mhealth apps is divers and include
all health care areas from information, prevention,
care of complaints or also products, self-management,
therapy, rehabilitation in the form of aftercare and
monitoring. Further studies should consider the dif-
ferent fields and include them in acceptance studies.
The ability to operate a mhealth app is a basic pre-
requisite for the willingness to use it. The acceptance
of mhealth apps must also be investigated on people
who are ill, as otherwise there is an overestimation
of the capability and an underestimation of the actual
needs. In addition, research activities should be inves-
tigated with regard to the needs and requirements of
mhealth apps on behalf of limited users who require
barrier-free access due to their physical, mental or
even cognitive condition. It is important to understand
how the requirements of healthy people for the de-
sign of mhealth apps differ from those of people with
poorer health, in order to develop mhealth solutions
that can be adequately tailored to the corresponding
user groups. Due to lack of evidence so far on the
effectiveness specifically with regard to care and ther-
apeutic apps (Payne et al., 2015), further long-term
studies are needed.
ACKNOWLEDGEMENTS
The authors thank all participants for sharing their
thoughts and opinions. We would also like to thank
the reviewers for their valuable feedback.
REFERENCES
Acquisti, A., Brandimarte, L., and Loewenstein, G. (2015).
Privacy and human behavior in the age of information.
Science, 347(6221):509–514.
Alam, M. Z., Hoque, M. R., Hu, W., and Barua, Z.
(2020). Factors influencing the adoption of mhealth
services in a developing country: A patient-centric
study. International Journal of Information Manage-
ment, 50:128–143.
Applebaum, P. (2002). Privacy in psychiatric treatment:
threats and response. American Journal of Psychia-
try, 159(11):1809–1818.
climedo (2020). climedo. available online under https:
//climedo.de/ last checked on 01.20.2022.
Deng, Z., Hong, Z., Ren, C., Zhang, W., and Xiang, F.
(2018). What predicts patients’ adoption intention to-
ward mhealth services in china: empirical study. JMIR
mHealth and uHealth, 6(8):e172.
Fishbein, M. and Ajzen, I. (1977). Belief, attitude, inten-
tion, and behavior: An introduction to theory and re-
search.
Glass, G. V. (1976). Primary, secondary, and meta-analysis
of research. Educational researcher, 5(10):3–8.
Griebel, L., Enwald, H., Gilstad, H., Pohl, A.-L., More-
land, J., and Sedlmayr, M. (2018). ehealth literacy re-
search—quo vadis? Informatics for Health and Social
Care, 43(4):427–442.
IntHout, J., Ioannidis, J. P., and Borm, G. F. (2014).
The hartung-knapp-sidik-jonkman method for ran-
dom effects meta-analysis is straightforward and
ICT4AWE 2022 - 8th International Conference on Information and Communication Technologies for Ageing Well and e-Health
328