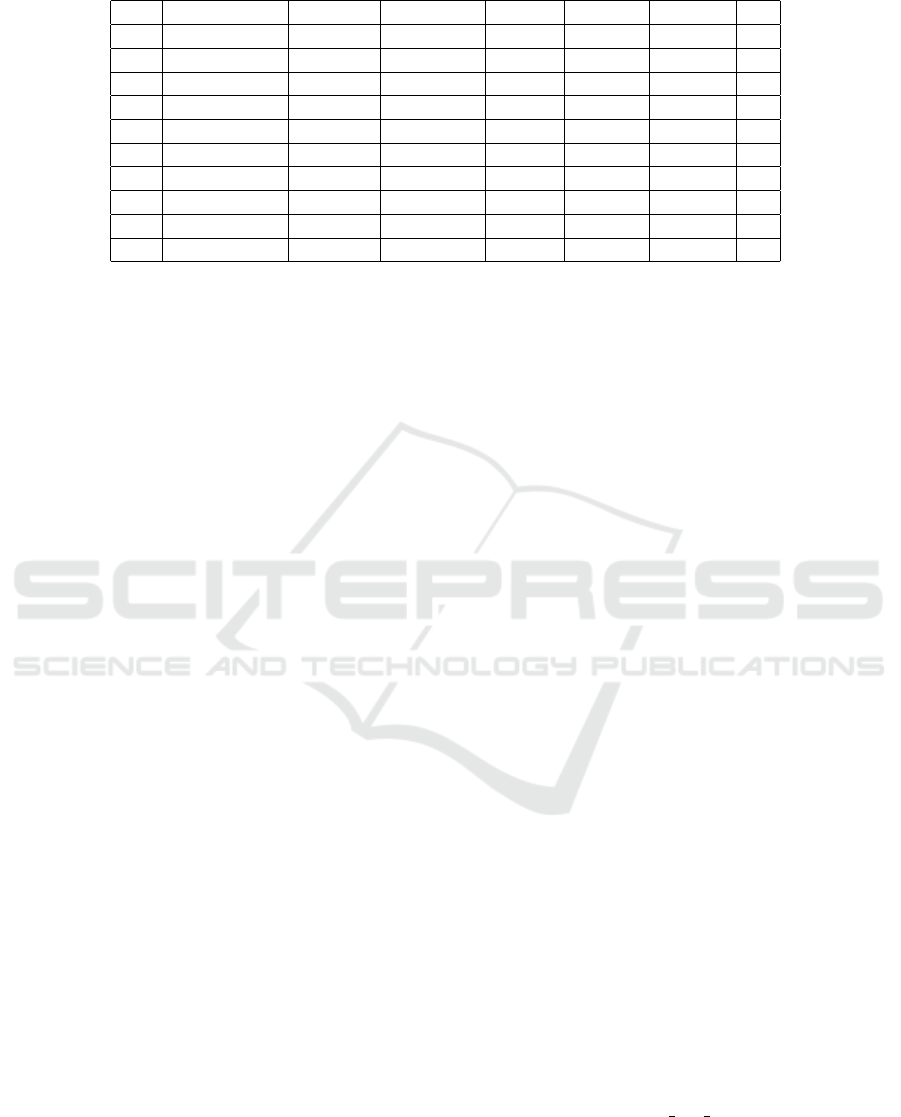
Table 1: Some example rows for data.
age job marital education default balance housing y
58 management married tertiary no 2143 yes no
44 technician single secondary no 29 yes no
33 entrepreneur married secondary no 2 yes no
47 blue-collar married unknown no 1506 yes no
33 unknown single unknown no 1 no no
35 management married tertiary no 231 yes no
28 management single tertiary no 447 yes no
42 entrepreneur divorced tertiary yes 2 yes no
58 retired married primary no 121 yes no
43 technician single secondary no 593 yes no
selection and after feature selection. Getting the
best algorithm is Random Forest to predict credit
defaulters compared to Decision Tree, Gradient
Boosting, and Extra Tree Classifier. They compared
the accuracy of prediction in defaulting payment
using machine learning techniques. In paper (Zhu
et al., 2019), they got a study for predicting loan de-
fault base on machine learning algorithms. The result
proving the performance of Random Forest showed
the best answer compared to the other algorithms
such as Decision Tree, SVM, and Logistic Regres-
sion. In the paper (Gupta et al., 2020), they applied
machine learning for predicting bank loan systems
by using Logistic Regression and Random Forest
and they did not compare to the other algorithms.
In the paper (Rahman and Kumar, 2020), they also
used KNN, SVM, Decision Tree, and Random Forest
to predict in machine learning based on customer
churn prediction. However, all of the papers have not
applied deep machine learning to predicting or got a
comparison for the models in deep machine learning.
In this paper, we got data (as shown in Fig 1) and
applied deep machine learning to take an accuracy
comparison using the LSTM model, GRU model,
and other algorithm models. We did try many
different algorithms and chose the best performance
for predicting a customer depositing in a bank. After
trying all the algorithms, we chose for performance
five best algorithms like Long-Short Term Memory
(LSTM), Gated Recurrent Unit (GRU), Bidirectional
Long-Short Term Memory (BiLSTM), Bidirectional
Gated Recurrent Unit (BiGRU) and Simple Re-
current Neuron Network (SimpleRNN). The main
purpose is to find a new comparison to generate
the customers’ information especially using deep
machine learning. Nowadays, banking is a useful
channel for relation in finance. They have the trend to
solve the financial work through the bank especially
in the economic crisis. Through Coivid-19, many
things have changed, the working is limited and they
tend to live at home and solve the problem online
where deposit banking is examined by customers.
In unprecedented circumstances, the banks have to
make phone calls to confirm the customers and have
some instructions to invite them to make a banking
term deposit. With machine learning, the banks could
solve the problems faster and decide the matters
rightly. This paper is aimed to confirm a customer
whether they could make a deposit or not using deep
machine learning. It is also convenient for the bank
to solve with an enormously large of customers in a
short time.
In this paper, we got input data from
the following website: https://www.kagg
le.com/vinicius150987/bank-full-machine-
learning/data. We also used the data set with
45,211 records and a total of 16 attributes. 1 shows
some examples extracted from data. The data consist
of numerical columns such as age, balance, day,
duration, campaign, pdays, and previous and other
columns are strings such as job, marital, default,
housing, loan, month, and deposit (y) which will be
turned into numeric by the StringIndexer. The term
deposit (y) is choosing for a label in predicting.
2 LITERATURE REVIEWS
Machine learning day by day has become a useful ap-
plication in most traditional stochastic methods espe-
cially in financial market forecasting as well as sup-
port vector machines (Ryll and Seidens, 2019; Ghani
et al., 2019). In this paper, we covered map all the
coulumns to convert the input numeric to string. Af-
ter that, we add all the attributes to the features. In the
next step, we apply train test split to slit the data. We
also try all the algorithms and choose some good algo-
rithms which are suitable to predict such as Gradient-
Boosted Trees, Random Forest, Decision Tree, Logis-
tic Regression, and deep learning machine is LSTM
ICEIS 2022 - 24th International Conference on Enterprise Information Systems
268