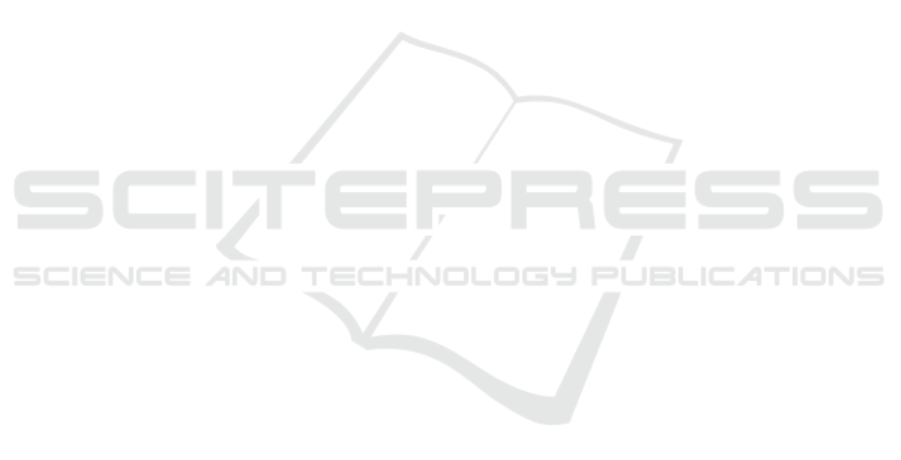
De Hoog, J., Mercelis, S., and Hellinckx, P. (2019). Improv-
ing machine learning-based decision-making through
inclusion of data quality. CEUR Workshop Proceed-
ings, 2491.
Deja, K. (2019). Using machine learning techniques for
data quality monitoring in cms and alice. Proceedings
of Science, 350.
Efron, B. (2020). Prediction, estimation, and attribu-
tion. Journal of the American Statistical Association,
115(530):636–655.
Ehrlinger, L., Haunschmid, V., Palazzini, D., and Lettner,
C. (2019). A daql to monitor data quality in machine
learning applications. In Database and Expert Sys-
tems Applications, pages 227–237.
El Ioini, N., H
¨
astbacka, D., Pahl, C., and Taibi, D. (2021).
Platforms for serverless at the edge: A review. In
Advances in Service-Oriented and Cloud Computing.
Springer.
Fang, D., Liu, X., Romdhani, I., Jamshidi, P., and Pahl, C.
(2016). An agility-oriented and fuzziness-embedded
semantic model for collaborative cloud service search,
retrieval and recommendation. Future Gener. Comput.
Syst., 56:11–26.
Femminella, M. and Reali, G. (2019). Gossip-based mon-
itoring of virtualized resources in 5g networks. In
IEEE INFOCOM 2019 - IEEE Conference on Com-
puter Communications Workshops, INFOCOM Work-
shops 2019, pages 378–384. IEEE.
Gand, F., Fronza, I., Ioini, N. E., Barzegar, H. R., Azimi,
S., and Pahl, C. (2020). A fuzzy controller for self-
adaptive lightweight container orchestration. In In-
ternational Conference on Cloud Computing and Ser-
vices Science CLOSER.
Hong, C. and Varghese, B. (2019). Resource management
in fog/edge computing: A survey on architectures,
infrastructure, and algorithms. ACM Comput. Surv.,
52(5):97:1–97:37.
Jamshidi, P., Sharifloo, A. M., Pahl, C., Metzger, A., and
Estrada, G. (2015). Self-learning cloud controllers:
Fuzzy q-learning for knowledge evolution. In 2015
International Conference on Cloud and Autonomic
Computing, pages 208–211. IEEE Computer Society.
Javed, A., Malhi, A., and Fr
¨
amling, K. (2020). Edge
computing-based fault-tolerant framework: A case
study on vehicular networks. In Intl Wireless
Communications and Mobile Computing Conference,
IWCMC 2020. IEEE.
Karkouch, A., Mousannif, H., Moatassime, H. A., and
Noel, T. (2016). Data quality in internet of things: A
state-of-the-art survey. Journal of Network and Com-
puter Applications, 73:57 – 81.
Lwakatare, L. E., Raj, A., Bosch, J., Olsson, H. H., and
Crnkovic, I. (2019). A taxonomy of software engi-
neering challenges for machine learning systems: An
empirical investigation. In Agile Processes in Soft-
ware Engineering and Extreme Programming - 20th
International Conference. Springer.
Mantri, D., Prasad, N. R., and Prasad, R. (2013). BHCDA:
bandwidth efficient heterogeneity aware cluster based
data aggregation for wireless sensor network. In Intl
Confe on Advances in Computing, Communications
and Informatics. IEEE.
Mendonca, N. C., Jamshidi, P., Garlan, D., and Pahl, C.
(2021). Developing self-adaptive microservice sys-
tems: Challenges and directions. IEEE Software,
38(2):70–79.
Mohammedameen, I., Mkwawa, I., and Sun, L. (2019).
Follow-me prefetching for video streaming over mo-
bile edge computing networks. In 2019 IEEE Smart-
World, pages 1937–1942. IEEE.
Pahl, C. and Azimi, S. (2021). Constructing dependable
data-driven software with machine learning. IEEE
Softw., 38(6):88–97.
Pahl, C., Jamshidi, P., and Zimmermann, O. (2018). Archi-
tectural principles for cloud software. ACM Transac-
tions on Internet Technology (TOIT), 18(2):17.
Pomalo, M., Le, V. T., Ioini, N. E., Pahl, C., and Barzegar,
H. R. (2020). Service migration in multi-domain cel-
lular networks based on machine learning approaches.
In Intl Conf on Internet of Things: Systems, Manage-
ment and Security, IOTSMS 2020. IEEE.
Scolati, R., Fronza, I., Ioini, N. E., Samir, A., and Pahl,
C. (2019). A containerized big data streaming archi-
tecture for edge cloud computing on clustered single-
board devices. In Intl Conf on Cloud Computing and
Services Science.
Sheng, V. S., Provost, F. J., and Ipeirotis, P. G. (2008). Get
another label? improving data quality and data mining
using multiple, noisy labelers. In 14th SIGKDD In-
ternational Conference on Knowledge Discovery and
Data Mining, pages 614–622. ACM.
Sicari, S., Rizzardi, A., Miorandi, D., Cappiello, C., and
Coen-Porisini, A. (2016). A secure and quality-aware
prototypical architecture for the internet of things. In-
formation Systems, 58:43 – 55.
Sridhar, V., Subramanian, S., Arteaga, D., Sundararaman,
S., Roselli, D. S., and Talagala, N. (2018). Model
governance: Reducing the anarchy of production ml.
In USENIX Annual Technical Conference.
Taibi, D., Lenarduzzi, V., and Pahl, C. (2020). Microser-
vices Anti-patterns: A Taxonomy. Springer.
Tokunaga, K., Kawamura, K., and Takaya, N. (2016). High-
speed uploading architecture using distributed edge
servers on multi-rat heterogeneous networks. In IEEE
International Symposium on Local and Metropolitan
Area Networks, LANMAN 2016, pages 1–2. IEEE.
von Leon, D., Miori, L., Sanin, J., Ioini, N. E., Helmer, S.,
and Pahl, C. (2019). A lightweight container middle-
ware for edge cloud architectures. In Fog and Edge
Computing, pages 145–170. Wiley.
Wang, F., Zhang, M., Wang, X., Ma, X., and Liu, J. (2020).
Deep learning for edge computing applications: A
state-of-the-art survey. IEEE Access, 8:58322–58336.
Zhao, H., Yi, D., Zhang, M., Wang, Q., Xinyue, S., and Zhu,
H. (2019). Multipath transmission workload balanc-
ing optimization scheme based on mobile edge com-
puting in vehicular heterogeneous network. IEEE Ac-
cess, 7:116047–116055.
CLOSER 2022 - 12th International Conference on Cloud Computing and Services Science
312