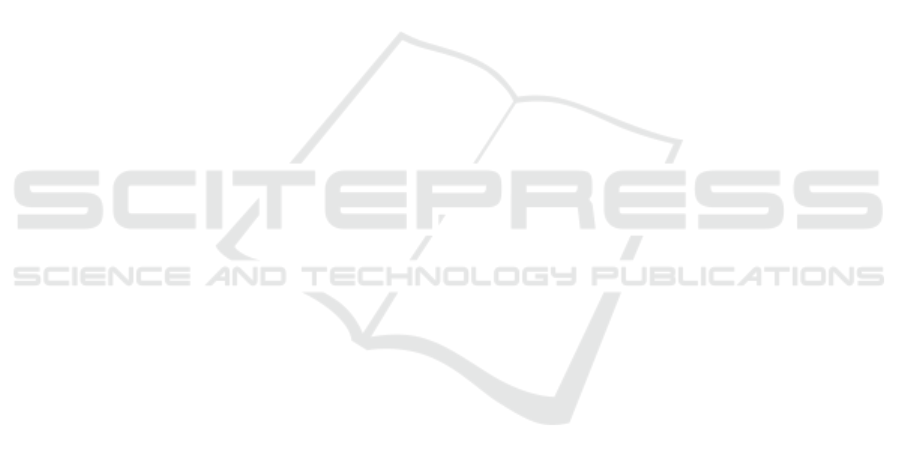
REFERENCES
Akkiraju, N., Edelsbrunner, H., Facello, M., Fu, P., M
¨
ucke,
P., E., and Varela, C. (1995). Alpha Shapes: Definition
and Software. In Proceedings on International Com-
putational Geometry Software Workshop, Minneapo-
lis.
Aslani, M. and Seipel, S. (2020). A fast instance selec-
tion method for support vector machines in building
extraction. Applied Soft Computing, 97:106716.
Aslani, M. and Seipel, S. (2021). Efficient and decision
boundary aware instance selection for support vector
machines. Information Sciences, 577:579–598.
Aslani, M. and Seipel, S. (2022). Automatic identification
of utilizable rooftop areas in digital surface models for
photovoltaics potential assessment. Applied Energy,
306, Part A:118033.
Awrangjeb, M. and Fraser, C. S. (2014). Automatic Seg-
mentation of Raw LIDAR Data for Extraction of
Building Roof. Remote Sensing, 6(5):3716–3751.
B
´
odis, K., Kougias, I., J
¨
ager-Waldau, A., Taylor, N., and
Szab
´
o, S. (2019). A high-resolution geospatial assess-
ment of the rooftop solar photovoltaic potential in the
European Union. Renewable and Sustainable Energy
Reviews, 114:109309.
Byrne, J., Taminiau, J., Kurdgelashvili, L., and Kim, K. N.
(2015). A review of the solar city concept and meth-
ods to assess rooftop solar electric potential, with an
illustrative application to the city of Seoul. Renewable
and Sustainable Energy Reviews, 41:830–844.
Chen, D., Zhang, L., Mathiopoulos, P. T., and Huang, X.
(2014). A Methodology for Automated Segmenta-
tion and Reconstruction of Urban 3-D Buildings from
ALS Point Clouds. IEEE Journal of Selected Topics
in Applied Earth Observations and Remote Sensing,
7(10):4199–4217.
Fu, P. and Rich, P. M. (2000). The Solar Analyst 1.0 Man-
ual. Technical report, Helios Environmental Modeling
Institute (HEMI), USA.
Gassar, A. A. A. and Cha, S. H. (2021). Review of
geographic information systems-based rooftop solar
photovoltaic potential estimation approaches at urban
scales. Applied Energy, 291:116817.
Gribov, A. (2019). Optimal Compression of a Polyline
While Aligning to Preferred Directions. In 2019 In-
ternational Conference on Document Analysis and
Recognition Workshops (ICDARW), volume 1, pages
98–102.
Huang, Y., Chen, Z., Wu, B., Chen, L., Mao, W., Zhao, F.,
Wu, J., Wu, J., and Yu, B. (2015). Estimating Roof
Solar Energy Potential in the Downtown Area Using
a GPU-Accelerated Solar Radiation Model and Air-
borne LiDAR Data. Remote Sensing, 7(12):17212–
17233.
Joshi, S., Mittal, S., Holloway, P., Shukla, P. R.,
´
O Gal-
lach
´
oir, B., and Glynn, J. (2021). High resolu-
tion global spatiotemporal assessment of rooftop solar
photovoltaics potential for renewable electricity gen-
eration. Nature Communications, 12(1):5738.
Kingma, D. P. and Ba, J. (2015). Adam: A Method for
Stochastic Optimization. In International Confer-
ence on Learning Representations (ICLR), San Diego.
Ithaca.
Lingfors, D., Bright, J. M., Engerer, N. A., Ahlberg, J.,
Killinger, S., and Wid
´
en, J. (2017). Comparing the ca-
pability of low- and high-resolution LiDAR data with
application to solar resource assessment, roof type
classification and shading analysis. Applied Energy,
205:1216–1230.
Mohajeri, N., Assouline, D., Guiboud, B., Bill, A., Gud-
mundsson, A., and Scartezzini, J.-L. (2018). A city-
scale roof shape classification using machine learn-
ing for solar energy applications. Renewable Energy,
121:81–93.
Murphy, K. P. (2012). Machine Learning: A Probabilistic
Perspective. MIT Press, Cambridge, Massachusetts.
Qi, C. R., Yi, L., Su, H., and Guibas, L. J. (2017). Point-
Net++: Deep Hierarchical Feature Learning on Point
Sets in a Metric Space. In 31st Conference on Neural
Information Processing Systems (NIPS 2017), Califor-
nia.
Rich, P., Dubayah, R., Hetrick, W., and Saving, S. (1994).
Using Viewshed models to calculate intercepted so-
lar radiation: applications in ecology. American Soci-
ety for Photogrammetry and Remote Sensing Techni-
cal Papers, pages 524–529.
Rusu, R. B. (2009). Semantic 3D Object Maps for Everyday
Manipulation in Human Living Environments. PhD
thesis, Technical University of Munich, Munich, Ger-
many.
Sampath, A. and Shan, J. (2010). Segmentation and Recon-
struction of Polyhedral Building Roofs From Aerial
LiDAR Point Clouds. IEEE Transactions on Geo-
science and Remote Sensing, 48(3):1554–1567.
Shin, Y.-H., Son, K.-W., and Lee, D.-C. (2022). Semantic
Segmentation and Building Extraction from Airborne
LiDAR Data with Multiple Return Using PointNet++.
Applied Sciences, 12(4).
Sundararajan, D. (2017). Digital Image Processing A Sig-
nal Processing and Algorithmic Approach. Springer,
Singapore.
Tasoulis, N. G. P., Hofmeyr, D. P., and K., S. (2016).
Minimum Density Hyperplanes. Journal of Machine
Learning Research, 17(156):1–33.
Thebault, M., Clivill
´
e, V., Berrah, L., and Desthieux, G.
(2020). Multicriteria roof sorting for the integration
of photovoltaic systems in urban environments. Sus-
tainable Cities and Society, 60:102259.
Varney, N., Asari, V. K., and Graehling, Q. (2020). DALES:
A Large-scale Aerial LiDAR Data Set for Seman-
tic Segmentation. In 2020 IEEE/CVF Conference on
Computer Vision and Pattern Recognition Workshops
(CVPRW), pages 717–726.
A Spatially Detailed Approach to the Assessment of Rooftop Solar Energy Potential based on LiDAR Data
63