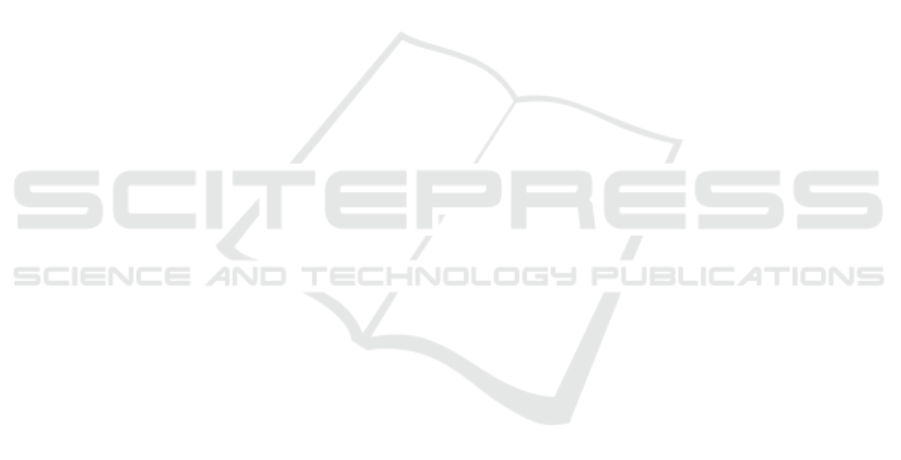
ror between our safety distance and the actual dis-
tance. As a result, our model can reduce the safety
margin between safety and traffic flow compared to
the RSS model. Therefore, we conclude that the RSS
model needs improvement.
Like the follow-up studies on the RSS model, we
will verify our model through various additional sim-
ulations (Chai et al., 2020; Xu et al., 2021).
ACKNOWLEDGEMENTS
This work was supported by Institute for Infor-
mation & communications Technology Planning &
Evaluation(IITP) grant funded by the Korea gov-
ernment(MSIT) (No.2021-0-01352, Development of
technology for validating the autonomous driving ser-
vices in perspective of laws and regulations)
REFERENCES
Bae, I., Moon, J., Jhung, J., Suk, H., Kim, T., Park, H., Cha,
J., Kim, J., Kim, D., and Kim, S. (2020). Self-driving
like a human driver instead of a robocar: Personalized
comfortable driving experience for autonomous vehi-
cles. arXiv preprint arXiv:2001.03908.
Battaglia, P. W., Hamrick, J. B., Bapst, V., Sanchez-
Gonzalez, A., Zambaldi, V., Malinowski, M., Tac-
chetti, A., Raposo, D., Santoro, A., Faulkner, R., et al.
(2018). Relational inductive biases, deep learning, and
graph networks. arXiv preprint arXiv:1806.01261.
Brackstone, M. and McDonald, M. (1999). Car-following:
a historical review. Transportation Research Part F:
Traffic Psychology and Behaviour, 2(4):181–196.
Caesar, H., Bankiti, V., Lang, A. H., Vora, S., Liong, V. E.,
Xu, Q., Krishnan, A., Pan, Y., Baldan, G., and Bei-
jbom, O. (2020). nuscenes: A multimodal dataset for
autonomous driving. In Proceedings of the IEEE/CVF
conference on computer vision and pattern recogni-
tion, pages 11621–11631.
Chai, C., Zeng, X., Wu, X., and Wang, X. (2019). Safety
evaluation of responsibility-sensitive safety (rss) on
autonomous car-following maneuvers based on sur-
rogate safety measurements. In 2019 IEEE In-
telligent Transportation Systems Conference (ITSC),
pages 175–180.
Chai, C., Zeng, X., Wu, X., and Wang, X. (2020). Eval-
uation and optimization of responsibility-sensitive
safety models on autonomous car-following maneu-
vers. Transportation research record, 2674(11):662–
673.
Chen, J., Ma, T., and Xiao, C. (2018). Fastgcn: fast learn-
ing with graph convolutional networks via importance
sampling. arXiv preprint arXiv:1801.10247.
Chen, J., Zhu, J., and Song, L. (2017). Stochastic training of
graph convolutional networks with variance reduction.
arXiv preprint arXiv:1710.10568.
Chiang, W.-L., Liu, X., Si, S., Li, Y., Bengio, S., and Hsieh,
C.-J. (2019). Cluster-gcn: An efficient algorithm for
training deep and large graph convolutional networks.
In Proceedings of the 25th ACM SIGKDD Interna-
tional Conference on Knowledge Discovery & Data
Mining, pages 257–266.
Cranmer, M., Sanchez Gonzalez, A., Battaglia, P., Xu, R.,
Cranmer, K., Spergel, D., and Ho, S. (2020). Discov-
ering symbolic models from deep learning with induc-
tive biases. Advances in Neural Information Process-
ing Systems, 33:17429–17442.
Defferrard, M., Bresson, X., and Vandergheynst, P. (2016).
Convolutional neural networks on graphs with fast lo-
calized spectral filtering. Advances in neural informa-
tion processing systems, 29.
Geiger, A., Lenz, P., Stiller, C., and Urtasun, R. (2013).
Vision meets robotics: The kitti dataset. The Inter-
national Journal of Robotics Research, 32(11):1231–
1237.
Guo, S., Lin, Y., Feng, N., Song, C., and Wan, H. (2019).
Attention based spatial-temporal graph convolutional
networks for traffic flow forecasting. In Proceedings
of the AAAI conference on artificial intelligence, vol-
ume 33, pages 922–929.
Houston, J., Zuidhof, G., Bergamini, L., Ye, Y., Chen,
L., Jain, A., Omari, S., Iglovikov, V., and Ondruska,
P. (2020). One thousand and one hours: Self-
driving motion prediction dataset. arXiv preprint
arXiv:2006.14480.
Kim, M.-J., Yu, S.-H., Kim, T.-H., Kim, J.-U., and Kim, Y.-
M. (2021). On the development of autonomous vehi-
cle safety distance by an rss model based on a variable
focus function camera. Sensors, 21(20):6733.
Kipf, T. N. and Welling, M. (2016). Semi-supervised clas-
sification with graph convolutional networks. arXiv
preprint arXiv:1609.02907.
Krajewski, R., Bock, J., Kloeker, L., and Eckstein, L.
(2018). The highd dataset: A drone dataset of natural-
istic vehicle trajectories on german highways for val-
idation of highly automated driving systems. In 2018
21st International Conference on Intelligent Trans-
portation Systems (ITSC), pages 2118–2125. IEEE.
Lee, S., Kim, J., Song, H., and Kim, S. (2014). A 3-
dimensional real-time traffic simulator considering the
interaction among autonomous and human-driven ve-
hicles. In 17th International IEEE Conference on In-
telligent Transportation Systems (ITSC), pages 1917–
1918. IEEE.
Lefevre, S., Carvalho, A., and Borrelli, F. (2015). Au-
tonomous car following: A learning-based approach.
In 2015 IEEE Intelligent Vehicles Symposium (IV),
pages 920–926. IEEE.
Li, L., Peng, X., Wang, F.-Y., Cao, D., and Li, L. (2018).
A situation-aware collision avoidance strategy for car-
following. IEEE/CAA Journal of Automatica Sinica,
5(5):1012–1016.
Li, Y., Yu, R., Shahabi, C., and Liu, Y. (2017). Diffusion
convolutional recurrent neural network: Data-driven
traffic forecasting. arXiv preprint arXiv:1707.01926.
SIMULTECH 2022 - 12th International Conference on Simulation and Modeling Methodologies, Technologies and Applications
216