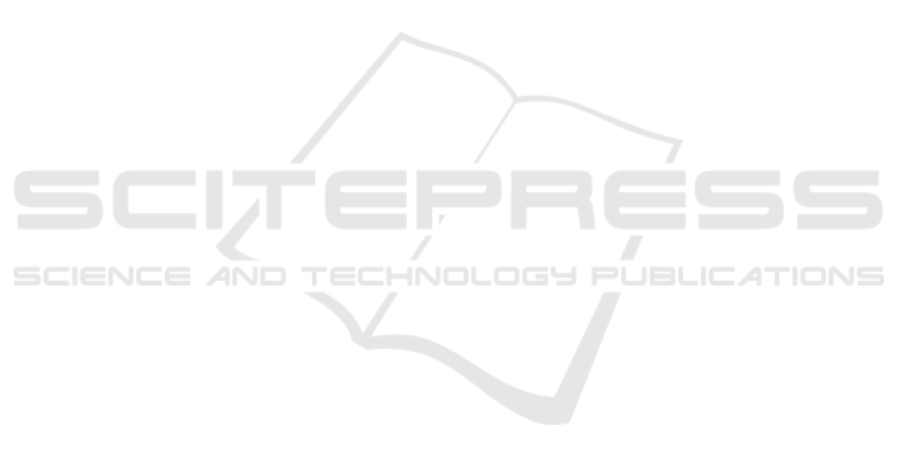
ried out on real cells opens the door to ”diagnosis in
real-time”, through detection using the Raman spec-
trum, a non-invasive and non-destructive technique
for the patient.
The proposed approach, based on the combination
of Raman spectroscopy and the use of machine learn-
ing models, allows obtaining data on the patient’s
cells to be identified as “malignant” or not in a matter
of minutes. This methodology does not aim to replace
the work of the doctor who remains at the center of
the diagnosis and treatment process but is a tool made
available to him.
The main contribution of this work consists in the
use of a dataset containing real information about a
patient under treatment at the National CancerInsti-
tute IRCCS G. Pascale Foundation, whose cells have
been analyzed by the Center for Nanophotonics and
Optoelectronics for Human Health (CNOS).
Therefore, the proposed approach has been tested
on an overall dataset containing 364 wavenumbers
where each corresponds to a sample of amplitude
across the various records of the dataset. The results
show good performance of the Random Forest Clas-
sifier which in the case of data augmentation reached
an accuracy of 89.98%.
The limitation of the study concerns the fact that
the classification was carried out on cells relating to
a single patient, because some were collected in the
tumor area, and others in adjacent but healthy areas.
So, in the future it could be interesting to inves-
tigate in three different directions: classification of
cells from different patients but with the same pathol-
ogy to assess whether the pathology has similar traits
in different patients (i); classification of cells from
different patients but with different tumor patholo-
gies to evaluate if there is an indicator, that is a
set of biological components, common for all onco-
logical pathologies (ii); classification of cells from
healthy patients and patients suffering from oncologi-
cal diseases to understand if some tumor traits are also
present in healthy patients, avoiding the disease with
effective prevention therapy (iii).
REFERENCES
Ardimento, P., Aversano, L., Bernardi, M. L., and Cimitile,
M. (2021). Deep neural networks ensemble for lung
nodule detection on chest ct scans. In 2021 Interna-
tional Joint Conference on Neural Networks (IJCNN),
pages 1–8.
Aversano, L., Bernardi, M. L., Cimitile, M., Iammarino,
M., Macchia, P. E., Nettore, I. C., and Verdone,
C. (2021a). Thyroid disease treatment prediction
with machine learning approaches. Procedia Com-
puter Science, 192:1031–1040. Knowledge-Based
and Intelligent Information and Engineering Sys-
tems: Proceedings of the 25th International Confer-
ence KES2021.
Aversano, L., Bernardi, M. L., Cimitile, M., and Pecori,
R. (2020). Early detection of parkinson disease us-
ing deep neural networks on gait dynamics. In 2020
International Joint Conference on Neural Networks
(IJCNN), pages 1–8.
Aversano, L., Bernardi, M. L., Cimitile, M., and Pecori,
R. (2020). Early detection of parkinson disease us-
ing deep neural networks on gait dynamics. In 2020
International Joint Conference on Neural Networks
(IJCNN), pages 1–8.
Aversano, L., Bernardi, M. L., Cimitile, M., and Pecori,
R. (2021b). Deep neural networks ensemble to de-
tect covid-19 from ct scans. Pattern Recognition,
120:108135.
Breiman, L. (2001). Random forests. Machine Learning,
45(1):5–32.
G., S. S. and K., M. (2019). Diagnosis of diabetes diseases
using optimized fuzzy rule set by grey wolf optimiza-
tion. Pattern Recognition Letters, 125:432 – 438.
Germond, A., Ichimura, T., da Chiu, L., Fujita, K., Watan-
abe, T. M., and Fujita, H. (2018). Cell type discrimina-
tion based on image features of molecular component
distribution. Scientific Reports, 8.
Henschke, C. I., McCauley, D. I., Yankelevitz, D. F.,
Naidich, D. P., McGuinness, G., Miettinen, O. S.,
Libby, D. M., Pasmantier, M. W., Koizumi, J., Altorki,
N. K., and Smith, J. P. (1999). Early lung cancer ac-
tion project: overall design and findings from baseline
screening. The Lancet, 354(9173):99–105.
Hsu, C.-C., Xu, J., Brinkhof, B., Wang, H., Cui, Z., Huang,
W. E., and Ye, H. (2020). A single-cell raman-based
platform to identify developmental stages of human
pluripotent stem cell-derived neurons. Proceedings
of the National Academy of Sciences, 117(31):18412–
18423.
Karayılan, T. and Kılıc¸, . (2017). Prediction of heart disease
using neural network. In 2017 International Confer-
ence on Computer Science and Engineering (UBMK),
pages 719–723.
Lussier, F., Missirlis, D., Spatz, J. P., and Mas-
son, J.-F. (2019). Machine-learning-driven surface-
enhanced raman scattering optophysiology reveals
multiplexed metabolite gradients near cells. ACS
Nano, 13(2):1403–1411. PMID: 30724079.
Miller, K. D., Siegel, R. L., Lin, C. C., Mariotto, A. B.,
Kramer, J. L., and Rowland, J. H. (2016). Cancer
treatment and survivorship statistics, 2016. CA Can-
cer J Clin, 66:271–289.
Mulvaney, S. P. and Keating, C. D. (2000). Raman spec-
troscopy. Analytical Chemistry, 72(12):145–158.
Neal, R. D., Tharmanathan, P., France, B., Din, N. U.,
Cotton, S. J., Fallon-Ferguson, J., Hamilton, W. T.,
Hendry, A., Hendry, M., Lewis, R., Macleod, U.,
Mitchell, E. D., Pickett, M., Rai, T. K., Shaw, K., Stu-
art, N. S., Tørring, M. L., Wilkinson, C., Williams,
B., Williams, N., and Emery, J. D. (2015). Is increased
Using Machine Learning for Classification of Cancer Cells from Raman Spectroscopy
23