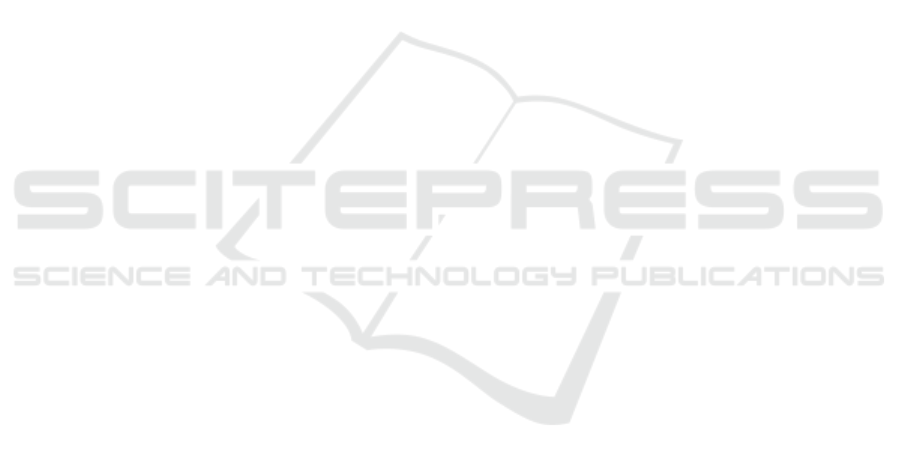
the network to let them behave in an unexpected and
erroneous way, while monitoring if an autonomous
vehicle trained with a customized DRL algorithm was
able to minimize the number of road accidents (colli-
sions) over time.
The experimental analysis results showed that
only 1 DRL vehicle can help to optimize traffic flows
in order to mitigate the number of collisions that re-
alistically would happen if no autonomous vehicles
were inserted in the network. We showed that the col-
lision frequency decreases while the number of steps
increases: this means that the DRL agent is able to
learn from the environment despite the presence of
bad behaviours.
As future work, we plan to add an increasing num-
ber of DRL vehicles in the network with the aim to
create more complex scenarios to evaluate the perfor-
mances of the proposed DRL model based on a reward
function that penalizes strong accelerations and lane-
changes, while rewards velocities under a target ve-
locity and near to the mean velocity of all the vehicles
in the network. Also, we would like to execute a no-
DRL scenario with the same network configuration in
order to compare DRL performance with no-DRL per-
formance.
ACKNOWLEDGEMENTS
This work has been funded by Spike Reply and par-
tially supported by MIUR - SecureOpenNets, EU
SPARTA, CyberSANE and E-CORRIDOR projects.
REFERENCES
BOMEY, N. (2018). U.S. vehicle deaths topped 40.000
in 2017, National Safety Council estimates. https:
//eu.usatoday.com/story/money/cars/2018/02/15/
national-safety-council-traffic-deaths/340012002/.
Accessed: Nov-2021.
Drozhzhin, A. (2015). Black hat usa 2015: The
full story of how that jeep was hacked.
https://www.kaspersky.com/blog/blackhat-jeep-
cherokee-hack-explained/9493/. Accessed: Nov-
2021.
Khatoun, R. and Zeadally, S. (2017). Cybersecurity and pri-
vacy solutions in smart cities. IEEE Communications
Magazine, 55(3):51–59.
Martinelli, F., Marulli, F., Mercaldo, F., and Santone, A.
(2021a). Neural networks for driver behavior analysis.
Electronics, 10(3):342.
Martinelli, F., Mercaldo, F., Nardone, V., Orlando, A., and
Santone, A. (2018a). Cluster analysis for driver ag-
gressiveness identification. In ICISSP, pages 562–
569.
Martinelli, F., Mercaldo, F., Nardone, V., Orlando, A., and
Santone, A. (2018b). Who’s driving my car? a ma-
chine learning based approach to driver identification.
In ICISSP, pages 367–372.
Martinelli, F., Mercaldo, F., Nardone, V., and Santone, A.
(2021b). Driver identification through formal meth-
ods. IEEE Transactions on Intelligent Transportation
Systems.
Miller, C. and Valasek, C. (2015). Remote exploitation of an
unaltered passenger vehicle. Black Hat USA, 2015(S
91).
Saiprasert, C. and Pattara-Atikom, W. (2013). Smartphone
enabled dangerous driving report system. In 2013 46th
Hawaii International Conference on System Sciences,
pages 1231–1237. IEEE.
Sikander, G. and Anwar, S. (2018). Driver fatigue detection
systems: A review. IEEE Transactions on Intelligent
Transportation Systems, 20(6):2339–2352.
Statista.com (2021). Projected size of the global
autonomous car market from 2019 to 2023.
https://www.statista.com/statistics/428692/projected-
size-of-global-autonomous-vehicle-market-by-
vehicle-type/. Accessed: Nov-2021.
Sutton, R. S. and Barto, A. G. (2018). Reinforcement learn-
ing: An introduction. MIT press.
Treiber, M. and Kesting, A. (2013). Traffic flow dynamics.
Traffic Flow Dynamics: Data, Models and Simulation,
Springer-Verlag Berlin Heidelberg.
Wu, C., Kreidieh, A., Parvate, K., Vinitsky, E., and
Bayen, A. M. (2017a). Flow: A modular learning
framework for autonomy in traffic. arXiv preprint
arXiv:1710.05465.
Wu, C., Kreidieh, A., Parvate, K., Vinitsky, E., and Bayen,
A. M. (2017b). Flow: Architecture and benchmark-
ing for reinforcement learning in traffic control. arXiv
preprint arXiv:1710.05465, page 10.
Yu, J., Chen, Z., Zhu, Y., Chen, Y., Kong, L., and Li,
M. (2016). Fine-grained abnormal driving behaviors
detection and identification with smartphones. IEEE
transactions on mobile computing, 16(8):2198–2212.
SECRYPT 2022 - 19th International Conference on Security and Cryptography
518