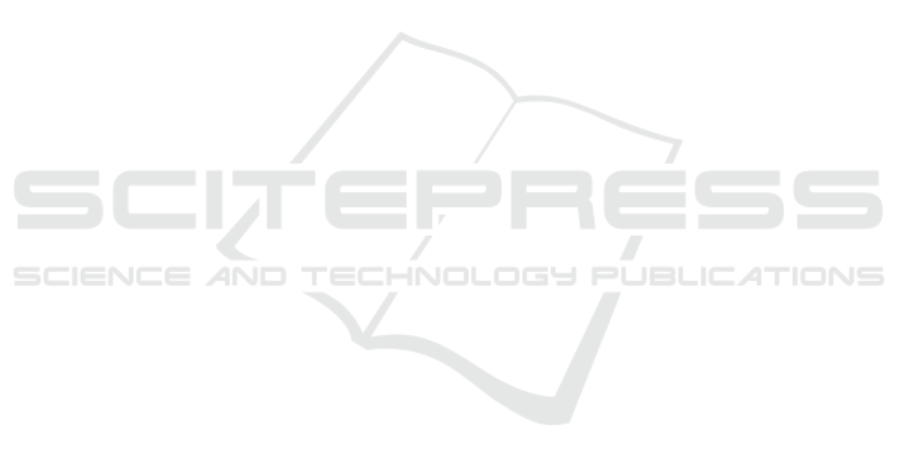
REFERENCES
Abel, M.-H., Benayache, A., Lenne, D., and Moulin, C.
(2007). E-memorae: a content-oriented environment
for e-learning. In E-Learning Networked Environ-
ments and Architectures, pages 186–205. Springer.
Akour, A., Ala’a, B., Barakat, M., Kanj, R., Fakhouri,
H. N., Malkawi, A., and Musleh, G. (2020). The
impact of the covid-19 pandemic and emergency dis-
tance teaching on the psychological status of univer-
sity teachers: a cross-sectional study in jordan. The
American journal of tropical medicine and hygiene,
103(6):2391.
Andres, F., Blancan, B., Foutouhi, F., Ghinea, G., and Leite,
M. C. (2021). Overview of the 4th decor workshop
2021. In 37th IEEE International Conference on Data
Engineering.
Bishay, A. (1996). Teacher motivation and job satisfaction:
A study employing the experience sampling method.
Journal of undergraduate Sciences, 3(3):147–155.
Cobos, C., Rodriguez, O., Rivera, J., Betancourt, J., Men-
doza, M., Le
´
on, E., and Herrera-Viedma, E. (2013).
A hybrid system of pedagogical pattern recommenda-
tions based on singular value decomposition and vari-
able data attributes. Information Processing & Man-
agement, 49(3):607–625.
Cooper, K. and Olson, M. R. (2020). The multiple ‘i’s’ of
teacher identity. In Changing research and practice,
pages 78–89. Routledge.
De Meo, P., Messina, F., Rosaci, D., and Sarn
´
e, G. M.
(2017). Combining trust and skills evaluation to form
e-learning classes in online social networks. Informa-
tion Sciences, 405:107–122.
Flores, M. A. and Day, C. (2006). Contexts which shape and
reshape new teachers’ identities: A multi-perspective
study. Teaching and teacher education, 22(2):219–
232.
Frenzel, A. C. (2014). Teacher emotions.
Friedman, I. A. (2000). Burnout in teachers: Shat-
tered dreams of impeccable professional performance.
Journal of clinical psychology, 56(5):595–606.
Herman, K. C., Hickmon-Rosa, J., and Reinke, W. M.
(2018). Empirically derived profiles of teacher stress,
burnout, self-efficacy, and coping and associated stu-
dent outcomes. Journal of Positive Behavior Interven-
tions, 20(2):90–100.
Heutte, J., Fenouillet, F., Kaplan, J., Martin-Krumm, C.,
and Bachelet, R. (2016). The eduflow model: A con-
tribution toward the study of optimal learning environ-
ments. In Flow experience, pages 127–143. Springer.
Imran, A. S., Muhammad, K., Fayyaz, N., Sajjad, M., et al.
(2021). A systematic mapping review on mooc rec-
ommender systems. IEEE Access.
Jain, S., Lall, M., and Singh, A. (2021). Teachers’ voices
on the impact of covid-19 on school education: Are
ed-tech companies really the panacea? Contemporary
Education Dialogue, 18(1):58–89.
Jenaro, C., Flores, N., and Arias, B. (2007). Burnout and
coping in human service practitioners. Professional
psychology: research and practice, 38(1):80.
Jiang, J. J. and Conrath, D. W. (1997). Semantic similarity
based on corpus statistics and lexical taxonomy. arXiv
preprint cmp-lg/9709008.
Kla
ˇ
snja-Mili
´
cevi
´
c, A., Vesin, B., and Ivanovi
´
c, M. (2018).
Social tagging strategy for enhancing e-learning expe-
rience. Computers & Education, 118:166–181.
Lachowska, B. H., Szpringer, M., Lachowski, S., and Pory-
dzaj, K. (2018). Conflict and facilitation between fam-
ily and occupational roles versus work-related stress
in the teachers’ group. Medical Studies/Studia Medy-
czne, 34(1):41–56.
Lizana, P. A., Vega-Fernadez, G., Gomez-Bruton, A., Ley-
ton, B., and Lera, L. (2021). Impact of the covid-19
pandemic on teacher quality of life: A longitudinal
study from before and during the health crisis. Inter-
national Journal of Environmental Research and Pub-
lic Health, 18(7):3764.
Marini, F. and Walczak, B. (2015). Particle swarm opti-
mization (pso). a tutorial. Chemometrics and Intelli-
gent Laboratory Systems, 149:153–165.
McIntyre, T. M., McIntyre, S. E., Barr, C. D., Francis, D. J.,
and Durand, A. C. (2017). Towards a dynamic inte-
grative theory of educator stress. In Educator Stress,
pages 261–289. Springer.
M
´
erida-L
´
opez, S. and Extremera, N. (2017). Emotional
intelligence and teacher burnout: A systematic re-
view. International Journal of Educational Research,
85:121–130.
Moore, P., Zhao, Z., and Pham, H. V. (2019). Towards
cloud-based personalised student-centric context-
aware e-learning pedagogic systems. In Conference
on Complex, Intelligent, and Software Intensive Sys-
tems, pages 331–342. Springer.
Mukhtar, K., Javed, K., Arooj, M., and Sethi, A. (2020).
Advantages, limitations and recommendations for on-
line learning during covid-19 pandemic era. Pakistan
journal of medical sciences, 36(COVID19-S4):S27.
Nashed, N. N., Lahoud, C., and Abel, M.-H. (2022). Edu-
cational resources recommender system for teachers:
Why and how? In Advances in Deep Learning, Artifi-
cial Intelligence and Robotics, pages 71–80. Springer.
Nashed, N. N., Lahoud, C., Abel, M.-H., Andr
`
es, F., and
Blancan, B. (2021). Mood detection ontology integra-
tion with teacher context. In 2021 20th IEEE Interna-
tional Conference on Machine Learning and Applica-
tions (ICMLA), pages 1710–1715. IEEE.
Nilashi, M., Asadi, S., Abumalloh, R. A., Samad, S., and
Ibrahim, O. (2020). Intelligent recommender systems
in the covid-19 outbreak: the case of wearable health-
care devices. Journal of Soft Computing and Decision
Support Systems, 7(4):8–12.
Oberle, E., Gist, A., Cooray, M. S., and Pinto, J. B.
(2020). Do students notice stress in teachers? asso-
ciations between classroom teacher burnout and stu-
dents’ perceptions of teacher social–emotional com-
petence. Psychology in the Schools, 57(11):1741–
1756.
Peimani, N. and Kamalipour, H. (2021). Online educa-
tion and the covid-19 outbreak: A case study of on-
Teacher Educational Resources Recommendation in the COVID-19 Context
597