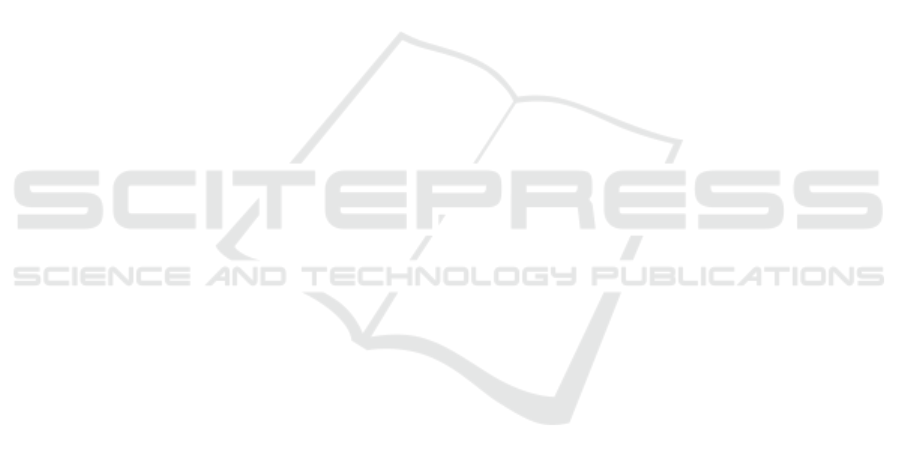
REFERENCES
Ahmed, N. K., Rossi, R. A., Lee, J. B., Willke, T. L., Zhou,
R., Kong, X., and Eldardiry, H. (2019). role2vec:
Role-based network embeddings. In Proc. DLG KDD,
pages 1–7.
Aiello, L. M., Deplano, M., Schifanella, R., and Ruffo, G.
(2012). People are strange when you’re a stranger:
Impact and influence of bots on social networks. In
Sixth International AAAI Conference on Weblogs and
Social Media.
Antenore, M., Camacho Rodriguez, J. M., and Panizzi,
E. (2022). A comparative study of bot detection
techniques with an application in twitter covid-19
discourse. Social Science Computer Review, page
08944393211073733.
Bail, C. A., Guay, B., Maloney, E., Combs, A., Hilly-
gus, D. S., Merhout, F., Freelon, D., and Volfovsky,
A. (2020). Assessing the russian internet research
agency’s impact on the political attitudes and behav-
iors of american twitter users in late 2017. Proceed-
ings of the national academy of sciences, 117(1):243–
250.
Brown, T. B., Mann, B., Ryder, N., Subbiah, M., Kaplan,
J., Dhariwal, P., Neelakantan, A., Shyam, P., Sastry,
G., Askell, A., et al. (2020). Language models are
few-shot learners. arXiv preprint arXiv:2005.14165.
Cai, H., Zheng, V. W., and Chang, K. C.-C. (2018). A com-
prehensive survey of graph embedding: Problems,
techniques, and applications. IEEE Transactions on
Knowledge and Data Engineering, 30(9):1616–1637.
Carter, D. (2016). Hustle and brand: The sociotechni-
cal shaping of influence. Social Media+ Society,
2(3):2056305116666305.
Cha, M., Haddadi, H., Benevenuto, F., and Gummadi, K.
(2010). Measuring user influence in twitter: The mil-
lion follower fallacy. In Proceedings of the Interna-
tional AAAI Conference on Web and Social Media,
volume 4.
Chavoshi, N., Hamooni, H., and Mueen, A. (2016). Debot:
Twitter bot detection via warped correlation. In Icdm,
pages 817–822.
De Domenico, M. and Altmann, E. G. (2020). Unraveling
the origin of social bursts in collective attention. Sci-
entific reports, 10(1):1–9.
Dong, G. and Liu, H. (2018). Feature engineering for ma-
chine learning and data analytics. CRC Press.
Donnat, C., Zitnik, M., Hallac, D., and Leskovec, J. (2018).
Learning structural node embeddings via diffusion
wavelets. In Proceedings of the 24th ACM SIGKDD
International Conference on Knowledge Discovery &
Data Mining, pages 1320–1329.
Feng, S., Wan, H., Wang, N., Li, J., and Luo, M. (2021).
Twibot-20: A comprehensive twitter bot detection
benchmark. In Proceedings of the 30th ACM Interna-
tional Conference on Information & Knowledge Man-
agement, pages 4485–4494.
Freelon, D., Bossetta, M., Wells, C., Lukito, J., Xia, Y.,
and Adams, K. (2020). Black trolls matter: Racial
and ideological asymmetries in social media disin-
formation. Social Science Computer Review, page
0894439320914853.
Freitas, C., Benevenuto, F., Ghosh, S., and Veloso, A.
(2015). Reverse engineering socialbot infiltration
strategies in twitter. In 2015 IEEE/ACM International
Conference on Advances in Social Networks Analysis
and Mining (ASONAM), pages 25–32. IEEE.
Gonz
´
alez-Bail
´
on, S. and De Domenico, M. (2021). Bots are
less central than verified accounts during contentious
political events. Proceedings of the National Academy
of Sciences, 118(11).
Goyal, P. and Ferrara, E. (2018). Graph embedding tech-
niques, applications, and performance: A survey.
Knowledge-Based Systems, 151:78–94.
Grover, A. and Leskovec, J. (2016). node2vec: Scal-
able feature learning for networks. In Proceedings
of the 22nd ACM SIGKDD international conference
on Knowledge discovery and data mining, pages 855–
864.
Henderson, K., Gallagher, B., Eliassi-Rad, T., Tong, H.,
Basu, S., Akoglu, L., Koutra, D., Faloutsos, C., and
Li, L. (2012). Rolx: structural role extraction & min-
ing in large graphs. In Proceedings of the 18th ACM
SIGKDD international conference on Knowledge dis-
covery and data mining, pages 1231–1239.
Hwang, T., Pearce, I., and Nanis, M. (2012). Socialbots:
Voices from the fronts. interactions, 19(2):38–45.
Kami
´
nski, B., Prałat, P., and Th
´
eberge, F. (2021). Mining
Complex Networks. CRC Press.
Lehmann, J., Gonc¸alves, B., Ramasco, J. J., and Cattuto,
C. (2012). Dynamical classes of collective attention
in twitter. In Proceedings of the 21st international
conference on World Wide Web, pages 251–260.
Matwin, S., Milios, A., Prałat, P., Soares, A., and Th
´
eberge,
F. (2021). Survey of generative methods for social
media analysis. arXiv preprint arXiv:2112.07041.
Mikolov, T., Sutskever, I., Chen, K., Corrado, G. S., and
Dean, J. (2013). Distributed representations of words
and phrases and their compositionality. In Advances in
neural information processing systems, pages 3111–
3119.
Monti, F., Frasca, F., Eynard, D., Mannion, D., and Bron-
stein, M. M. (2019). Fake news detection on social
media using geometric deep learning. arXiv preprint
arXiv:1902.06673.
Perozzi, B., Al-Rfou, R., and Skiena, S. (2014). Deepwalk:
Online learning of social representations. In Proceed-
ings of the 20th ACM SIGKDD international confer-
ence on Knowledge discovery and data mining, pages
701–710.
Ribeiro, L. F., Saverese, P. H., and Figueiredo, D. R.
(2017). struc2vec: Learning node representations
from structural identity. In Proceedings of the 23rd
ACM SIGKDD international conference on knowl-
edge discovery and data mining, pages 385–394.
Rossi, R. A. and Ahmed, N. K. (2014). Role discovery in
networks. IEEE Transactions on Knowledge and Data
Engineering, 27(4):1112–1131.
DATA 2022 - 11th International Conference on Data Science, Technology and Applications
60