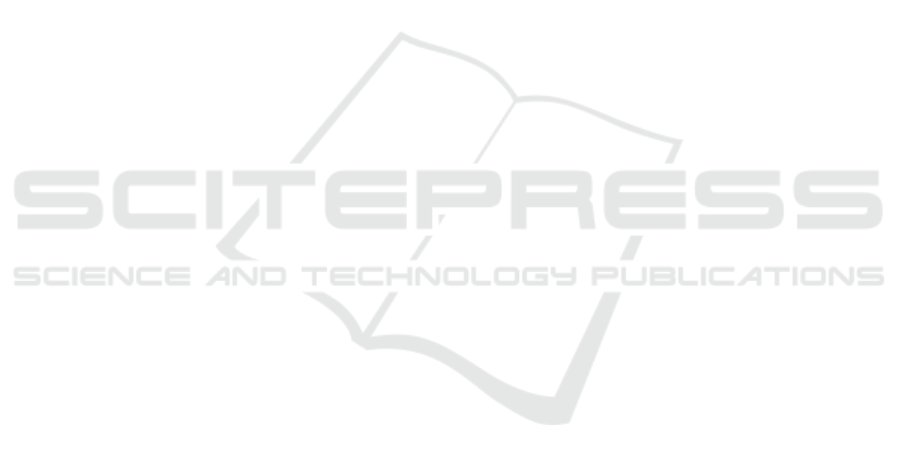
ready show a nearly 27% entailment. Although these
data records are substantially larger and more diverse,
the model seems to adapt. However, concerning our
research question, we cannot claim to have obtained
satisfactory results with a minimal amount of real-
world data. At this point, another research objec-
tive emerges, which has already been articulated by
Burgdorf et al. (Burgdorf et al., 2020). To say re-
liably whether given metadata is useful for semanti-
cally modeling tabular data requires some kind of as-
sessment or evaluation. The authors propose to use
historical data from the (potentially) established on-
tology to make some kind of prediction about how
much manual effort a given semantic model will need
with a given data set. Schauppenlehner and Muhar
(Schauppenlehner and Muhar, 2018) support this ap-
proach.
Finally, our conclusion is somewhat ambivalent.
We were able to test the present framework on an
open-domain setting and achieved valuable results,
even if not in the few-shot setting. However, many
open research questions remain: How can the genera-
tions of DTG systems be qualitatively evaluated? We
have been able to identify a method that allows us to
assess the degree of entailment, but this does not tell
us anything about factual correctness, nor whether it
is semantically relevant for use in the context of Open
Data Portals. Furthermore, our results show that the
amount of metadata is crucial for the performance of a
DTG model. If not all the information needed for the
generation can be obtained from the table, we must
rely on additional information. At this point, a vicious
circle arises because, in order to generate metadata,
we need metadata.
REFERENCES
(2013). G8 open data charter and technical annex.
Brown, T. B., Mann, B., Ryder, N., Subbiah, M., Kaplan,
J., Dhariwal, P., Neelakantan, A., Shyam, P., Sastry,
G., Askell, A., et al. (2020). Language models are
few-shot learners. arXiv preprint arXiv:2005.14165.
Burgdorf, A., Paulus, A., Pomp, A., and Meisen, T. (2022).
Vc-slam—a handcrafted data corpus for the construc-
tion of semantic models. Data, 7(2):17.
Burgdorf, A., Pomp, A., and Meisen, T. (2020). Towards
nlp-supported semantic data management. arXiv
preprint arXiv:2005.06916.
Chandola, T. and Booker, C. (2022). Archival and Sec-
ondary Data. SAGE.
Chen, D. L. and Mooney, R. J. (2008). Learning to
sportscast: a test of grounded language acquisition. In
Proceedings of the 25th international conference on
Machine learning, pages 128–135.
Chen, Z., Eavani, H., Chen, W., Liu, Y., and Wang,
W. Y. (2019). Few-shot nlg with pre-trained language
model. arXiv preprint arXiv:1904.09521.
Devlin, J., Chang, M.-W., Lee, K., and Toutanova, K.
(2018). Bert: Pre-training of deep bidirectional trans-
formers for language understanding. arXiv preprint
arXiv:1810.04805.
Dhingra, B., Faruqui, M., Parikh, A., Chang, M.-W., Das,
D., and Cohen, W. W. (2019). Handling divergent ref-
erence texts when evaluating table-to-text generation.
arXiv preprint arXiv:1906.01081.
Filippova, K. (2020). Controlled hallucinations: Learning
to generate faithfully from noisy data. arXiv preprint
arXiv:2010.05873.
Kingma, D. P. and Ba, J. (2014). Adam: A
method for stochastic optimization. arXiv preprint
arXiv:1412.6980.
Lebret, R., Grangier, D., and Auli, M. (2016). Neural text
generation from structured data with application to the
biography domain. arXiv preprint arXiv:1603.07771.
Lin, C.-Y. (2004). Rouge: A package for automatic evalu-
ation of summaries. In Text summarization branches
out, pages 74–81.
Nan, L., Radev, D., Zhang, R., Rau, A., Sivaprasad,
A., Hsieh, C., Tang, X., Vyas, A., Verma, N., Kr-
ishna, P., et al. (2020). Dart: Open-domain struc-
tured data record to text generation. arXiv preprint
arXiv:2007.02871.
Papineni, K., Roukos, S., Ward, T., and Zhu, W.-J. (2002).
Bleu: a method for automatic evaluation of machine
translation. In Proceedings of the 40th annual meet-
ing of the Association for Computational Linguistics,
pages 311–318.
Parikh, A. P., Wang, X., Gehrmann, S., Faruqui, M., Dhin-
gra, B., Yang, D., and Das, D. (2020). Totto: A con-
trolled table-to-text generation dataset. arXiv preprint
arXiv:2004.14373.
Portet, F., Reiter, E., Gatt, A., Hunter, J., Sripada, S., Freer,
Y., and Sykes, C. (2009). Automatic generation of
textual summaries from neonatal intensive care data.
Artificial Intelligence, 173(7-8):789–816.
Radford, A., Narasimhan, K., Salimans, T., and Sutskever,
I. (2018). Improving language understanding by gen-
erative pre-training.
Radford, A., Wu, J., Child, R., Luan, D., Amodei, D.,
Sutskever, I., et al. (2019). Language models are un-
supervised multitask learners. OpenAI blog, 1(8):9.
Rebuffel, C., Roberti, M., Soulier, L., Scoutheeten, G., Can-
celliere, R., and Gallinari, P. (2021). Controlling hal-
lucinations at word level in data-to-text generation.
arXiv preprint arXiv:2102.02810.
Schauppenlehner, T. and Muhar, A. (2018). Theoretical
availability versus practical accessibility: The criti-
cal role of metadata management in open data portals.
Sustainability, 10(2):545.
Tygel, A., Auer, S., Debattista, J., Orlandi, F., and Cam-
pos, M. L. M. (2016). Towards cleaning-up open
data portals: A metadata reconciliation approach. In
2016 IEEE Tenth International Conference on Seman-
tic Computing (ICSC), pages 71–78. IEEE.
Wang, H. (2020). Revisiting challenges in data-to-text
generation with fact grounding. arXiv preprint
arXiv:2001.03830.
DATA 2022 - 11th International Conference on Data Science, Technology and Applications
106