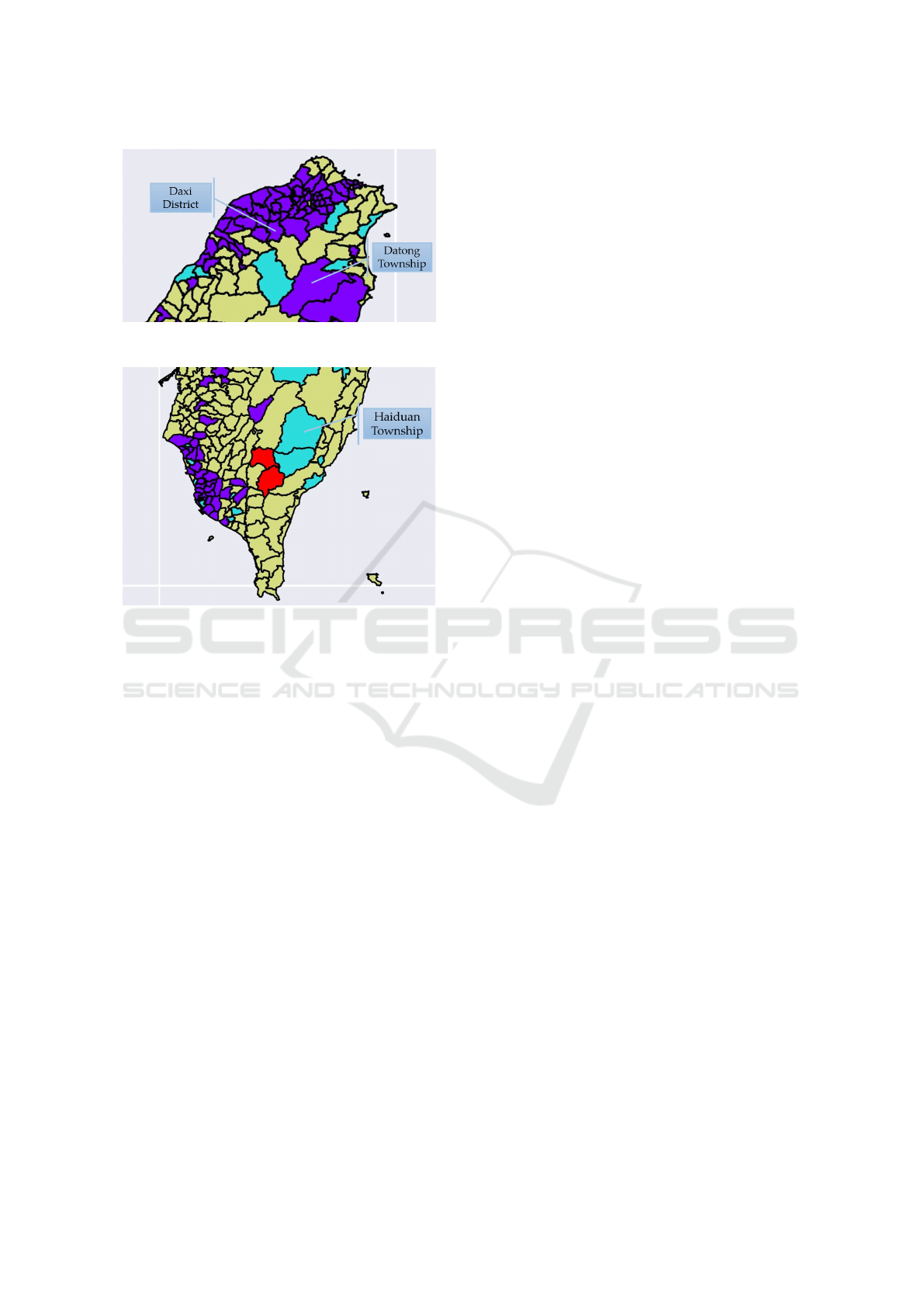
Figure 4: The regions affected by the North Link Highway.
Figure 5: The regions affected by the South Link Highway.
7 CONCLUSION
We used clustering methods to cluster the samples
generated by the simulation system of infectious dis-
eases to cluster administrative regions with similar
conditions of epidemic transmission. We also iden-
tified urban and non-urban areas by clustering meth-
ods. The result of clustering was then used to label
the samples to build decision trees. From the deci-
sion trees we built, we found age distributions are the
important features distinguishing the rural and urban
areas. In addition, by further analyzing the result, we
also found that road infrastructure may be important
to epidemic transmission.
ACKNOWLEDGMENT
This study was supported in part by MOST, Taiwan
by Grants 108-2221-E-001-011-MY3 and 110-2222-
E-033-005-.
REFERENCES
Balcan, D., Colizza, V., Gonc¸alves, B., Hu, H., Ramasco,
J. J., and Vespignani, A. (2009). Multiscale mobility
networks and the spatial spreading of infectious dis-
eases. Proceedings of the National Academy of Sci-
ences, 106(51):21484–21489.
Bargain, O. and Aminjonov, U. (2020). Trust and com-
pliance to public health policies in times of covid-19.
Journal of Public Economics, 192:104316.
Bijkerk, P., van Lier, E., van Vliet, J., and Kretzschmar,
M. Effecten van vergrijzing op infectieziekten. Ned
Tijdschr Geneeskd 2010;154:A1613.
Breiman, L., Friedman, J. H., Olshen, R. A., and Stone,
C. J. (1984). Classification and Regression Trees.
Wadsworth and Brooks, Monterey, CA.
Chang, H., Chuang, J., Chern, T., Stein, M., Coker, R.,
Wang, D., and Hsu, T. (2014). A comparison be-
tween a deterministic, compartmental model and an
individual based-stochastic model for simulating the
transmission dynamics of pandemic influenza. In 4th
International Conference On Simulation And Model-
ing Methodologies, Technologies And Applications,
SIMULTECH 2014, Vienna, Austria, August 28-30,
2014, pages 586–594. IEEE.
Heald-Sargent, T., Muller, W. J., Zheng, X., Rippe, J., Patel,
A. B., and Kociolek, L. K. (2020). Age-Related Dif-
ferences in Nasopharyngeal Severe Acute Respiratory
Syndrome Coronavirus 2 (SARS-CoV-2) Levels in
Patients With Mild to Moderate Coronavirus Disease
2019 (COVID-19). JAMA Pediatrics, 174(9):902–
903.
Lenzen, M., Li, M., Malik, A., Pomponi, F., Sun, Y.-
Y., Wiedmann, T., Faturay, F., Fry, J., Gallego, B.,
Geschke, A., et al. (2020). Global socio-economic
losses and environmental gains from the coronavirus
pandemic. PloS one, 15(7):e0235654.
Opendata platform (2022). Opendata platform. https://data.
gov.tw/en. Online; access 22 March, 2022.
Prothero, R. M. (1977). Disease and mobility: a neglected
factor in epidemiology. International journal of epi-
demiology, 6(3):259–267.
Quinlan, J. R. (1986). Induction of decision trees. Machine
Learning, 1:81–106.
Quinlan, J. R. (1993). C4.5: Programs for Machine Learn-
ing. Morgan Kaufmann Publishers Inc., San Fran-
cisco, CA, USA.
Ward, J. H. (1963). Hierarchical grouping to optimize an
objective function. Journal of the American Statistical
Association, 58(301):236–244.
World Health Organization (2010). Influenza a (h1n1)
pandemic 2009 - 2010. https://www.who.int/
emergencies/situations/influenza-a-(h1n1)-outbreak.
Online; access 22 March, 2022.
World Health Organization (2022). Coronavirus dis-
ease (covid-19) pandemic. https://www.who.int/
emergencies/diseases/novel-coronavirus-2019. On-
line; access 22 March, 2022.
DATA 2022 - 11th International Conference on Data Science, Technology and Applications
422