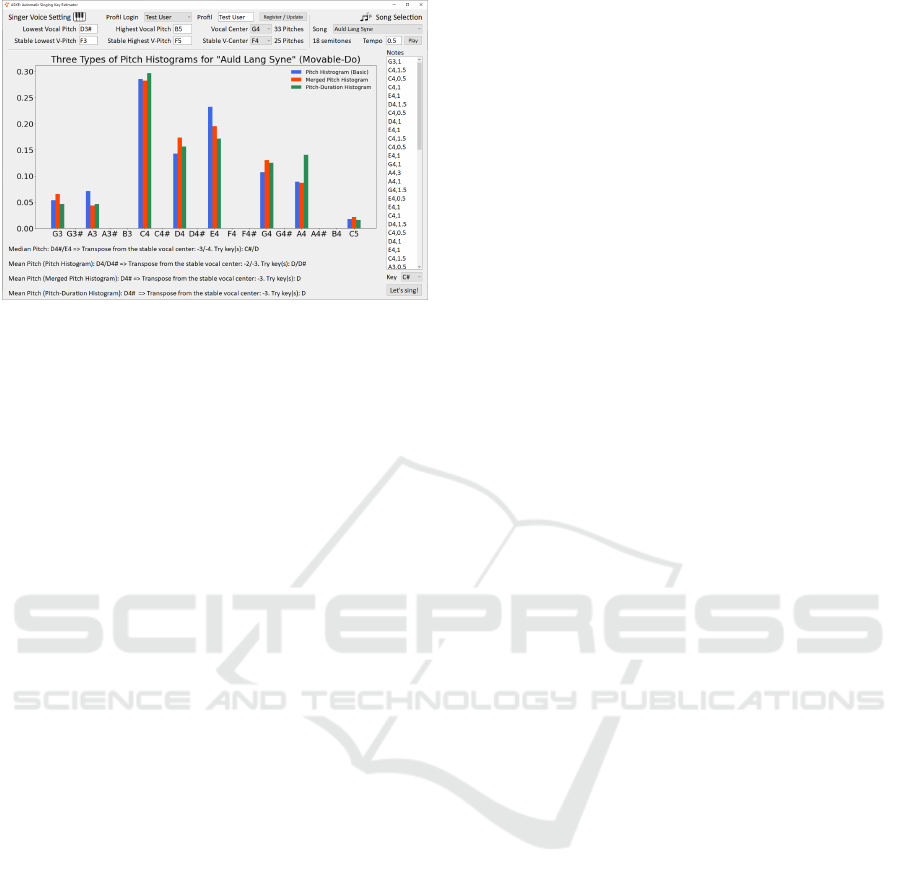
Figure 10: Screenshot of the software Automatic Singing
Key Estimator (ASKE).
et al., 2022b). For Auld Lang Syne, only one key was
automatically calculated for each amateur singer us-
ing a merged pitch histogram or a pitch-duration his-
togram, and the resulted key was scored satisfactorily
by the subjects in the singing experiment.
5 CONCLUSION AND OUTLOOK
This paper introduces two novel variants of the basic
pitch histogram, the merged pitch histogram and the
pitch-duration histogram, which consider each pitch’s
temporal information in the entire melody, the for-
mer in some measure (from the perspective of con-
tinuity) and the latter in full measure (from the per-
spective of weighting). Complemented by the anal-
ysis of song examples and the comparison between
histograms, the characteristics of the proposed his-
tograms are visually expounded. Furthermore, the
proposed histograms’ computational algorithms, ad-
vantages, limitations, and use cases in the latest re-
search works are exhibited in detail.
We believe that the merged pitch histogram and
the pitch-duration histogram are meaningful and help-
ful for music research, especially for the informa-
tion retrieval of songs’ main melody, as exemplified
in Sections 4.2 and 4.3. We expect the two intro-
duced histograms to be extensively applied in vari-
ous research aspects. Future work includes investi-
gating abundant new features extracted from the two
novel pitch histograms and applying these features to
various machine learning-based music research fields.
Besides, diverse transformation approaches of the ba-
sic pitch histogram, such as pitch class, folded fifths,
and melodic interval, can be practiced on the two
novel variants to generate more varied histograms
and new features, like the kurtosis of the pitch-class-
duration histogram.
Moving from overall pitch statistics to segment-
based features, windowing/framing the song’s
(pair, duration) sequence to generate time series
of merged pitch histograms and pitch-duration
histograms deserves further investigating, e.g., uti-
lizing the Time Series Subsequence Search Library
(TSSEARCH) (Folgado et al., 2022) to conduct a
subsequence similarity analysis on the melody.
REFERENCES
Adams, N. H., Bartsch, M. A., Shifrin, J., and Wakefield,
G. H. (2004). Time series alignment for music infor-
mation retrieval. In ISMIR 2004 – 5th International
Conference on Music Information Retrieval.
Barandas, M., Folgado, D., Fernandes, L., Santos, S.,
Abreu, M., Bota, P., Liu, H., Schultz, T., and Gam-
boa, H. (2020). Tsfel: Time series feature extraction
library. SoftwareX, 11:100456.
Brown, J. C. (1993). Determination of the meter of musical
scores by autocorrelation. The Journal of the Acousti-
cal Society of America, 94(4):1953–1957.
Folgado, D., Barandas, M., Antunes, M., Nunes, M. L.,
Liu, H., Hartmann, Y., Schultz, T., and Gamboa, H.
(2022). Tssearch: Time series subsequence search li-
brary. SoftwareX, 18:101049.
Gedik, A. C. and Bozkurt, B. (2010). Pitch-frequency
histogram-based music information retrieval for turk-
ish music. Signal Processing, 90(4):1049–1063.
Karydis, I. (2006). Symbolic music genre classification
based on note pitch and duration. In ADBIS 2006
– 10th East European Conference on Advances in
Databases and Information Systems, pages 329–338.
Springer.
Liu, H., Jiang, K., Gamboa, H., Xue, T., and Schultz, T.
(2022a). Bell shapes: Exploring the pitch distribution
of traditional han anhemitonic pentatonic folk songs.
(submitted).
Liu, H., Xue, T., Gamboa, H., Hertenstein, V., Xu, P., and
Schultz, T. (2022b). Automatic singing key estimation
for amateur singers using novel pitch-related features:
A singer-song-feature model. (submitted).
Lykartsis, A. and Lerch, A. (2015). Beat histogram features
for rhythm-based musical genre classification using
multiple novelty functions. In DAFx15 – 18th Inter-
national Conference on Digital Audio Effects.
McKay, C. (2010). Automatic music classification with
jMIR. PhD thesis, McGill University.
Tolonen, T. and Karjalainen, M. (2000). A computationally
efficient multipitch analysis model. IEEE transactions
on speech and audio processing, 8(6):708–716.
Tzanetakis, G. (2002). Manipulation, analysis and retrieval
systems for audio signals. Princeton University.
Tzanetakis, G. and Cook, P. (2002). Musical genre classifi-
cation of audio signals. IEEE Transactions on speech
and audio processing, 10(5):293–302.
SIGMAP 2022 - 19th International Conference on Signal Processing and Multimedia Applications
38