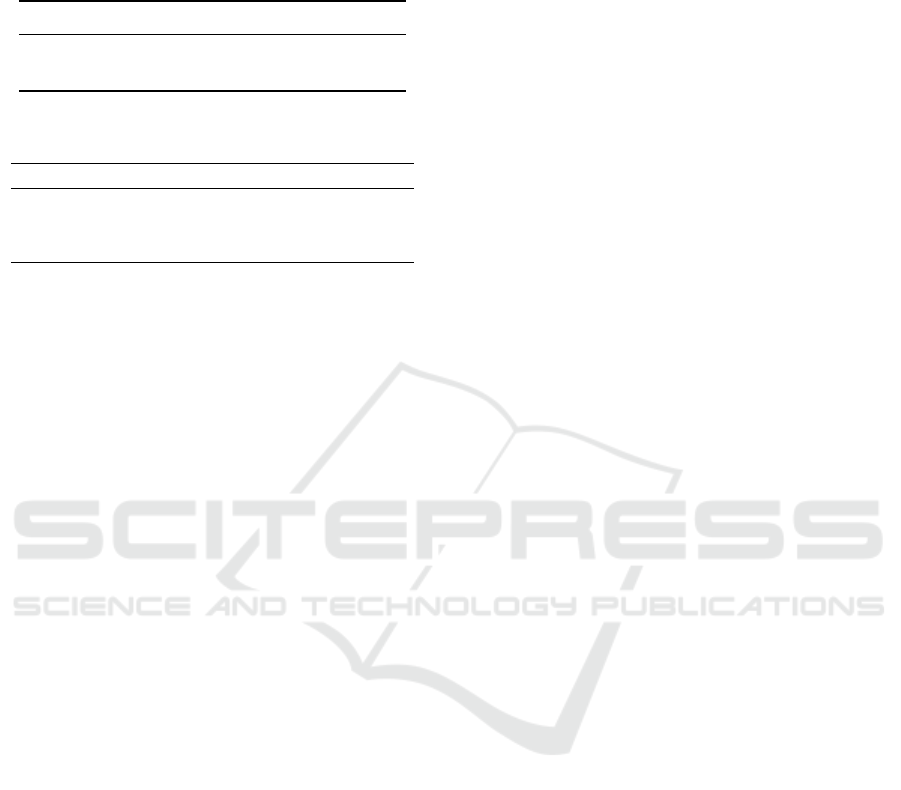
Table 5: Comparison of the best performing proposed ap-
proaches versus the considered competitor, in terms of clas-
sification accuracy (Acc), precision (Pre), recall (Rec) and
F1-score (F1).
Approach Acc Pre Rec F1
Proposed approach 0.76 0.80 0.69 0.74
Competitor 0.80 0.81 0.80 0.79
Table 6: Per-class comparison of the best performing pro-
posed approaches versus the considered competitor.
Angry Calm Happy Neutral Sad Competitor
Accuracy 0.56 0.70 0.80 0.93 0.78 0.80
Precision 0.76 0.62 0.89 0.93 0.79 0.81
Recall 0.58 0.58 0.70 0.94 0.64 0.80
F1-Score 0.58 0.60 0.78 0.94 0.71 0.79
To ensure a fair comparison, we took care performing
the same subject selection and using the very same
test set.
Table 5 reports the comparison of the best per-
forming proposed approach versus the considered
competitor. Results show that the competitor per-
forms slightly better than the proposed approach.
However, it is worth noting that while we analyse a
15 seconds long time windows, the competitor oper-
ates on the whole typing session that has, for the con-
sidered dataset, an average duration of 84 seconds.
This makes the competitor approach unsuited for the
short messages scenario (e.g., Twitter, instant messag-
ing app, etc.). To further analyse the proposed ap-
proach, in table 6 we report the same information but
organised per class. Interestingly, these results show
that our approach performs comparably or better w.r.t.
the competitor for three classes, with “Angry” and
“Calm” pushing down our average performance.
5 CONCLUSIONS
In this work we analysed the detection of users’ emo-
tional states based on the analysis of written text fo-
cusing on the case of short writing sessions (i.e., up
to a few seconds), typical of modern instant messag-
ing applications. To this aim, we leverage keystroke
dynamics, a behavioural biometric analysing habit-
ual typing patterns on a keyboard. In particular, we
introduced a time-windowing approach that allows
analysing users’ writing sessions in different batches,
re-shaping the emotion recognition task into a multi-
instance problem. The obtained results suggest that
even very short writing windows (in the order of
30”) are sufficient to recognise the subject’s emo-
tional state with the same level of accuracy as systems
based on the analysis of larger writing sessions (up to
a few minutes). Despite promising, it is worth noting
that the use of keystroke dynamics also presents some
challenges that need to be addressed, including pos-
sibly low generalisation (as the values of keystroke
parameters taken from a specific user may depend on
the type of software used) and inconsistencies in the
users’ typing rhythm due to external factors (e.g., in-
jury, fatigue, or distraction) instead of emotions.
Future works will focus on increasing the dataset
through some new data augmentation techniques, to
also balance the number of instances per class. Also,
we will investigate whether keystroke dynamics can
be combined with other biometrics or with other text-
based analyses (e.g., sentiment analysis) to further
improve the recognition performance. Finally, as in
this work we only used the Fixed Text Dataset (sec.
2), a further step will be testing the effectiveness of
the proposed approaches on the Free Text Dataset,
which is related to the subjects typing rhythm as they
wrote spontaneous sentences after watching videos.
The hope is that this type of analysis would better in-
tegrate with users’ daily activities and, of course, with
chat messages analysis, possibly providing more reli-
able, stable and precise predictions.
ACKNOWLEDGEMENTS
This work is supported by the Italian Ministry of Uni-
versity and Research (MUR) within the PRIN2017
- BullyBuster - A framework for bullying and cy-
berbullying action detection by computer vision and
artificial intelligence methods and algorithms (CUP:
F74I19000370001). The authors are also really grate-
ful to SCoPE group from the University of Naples
Federico II for the given support.
REFERENCES
Andrews, S., Tsochantaridis, I., and Hofmann, T. (2002).
Support vector machines for multiple-instance learn-
ing. Advances in neural information processing sys-
tems, 15.
Chawla, N. V., Bowyer, K. W., Hall, L. O., and Kegelmeyer,
W. P. (2002). Smote: synthetic minority over-
sampling technique. Journal of artificial intelligence
research, 16:321–357.
Chen, T., He, T., Benesty, M., Khotilovich, V., Tang, Y.,
Cho, H., Chen, K., et al. (2015). Xgboost: extreme
gradient boosting. R package version 0.4-2, 1(4):1–4.
Doran, G. and Ray, S. (2014). A theoretical and empir-
ical analysis of support vector machine methods for
multiple-instance classification. Machine learning,
97(1):79–102.
Identifying Users’ Emotional States through Keystroke Dynamics
213