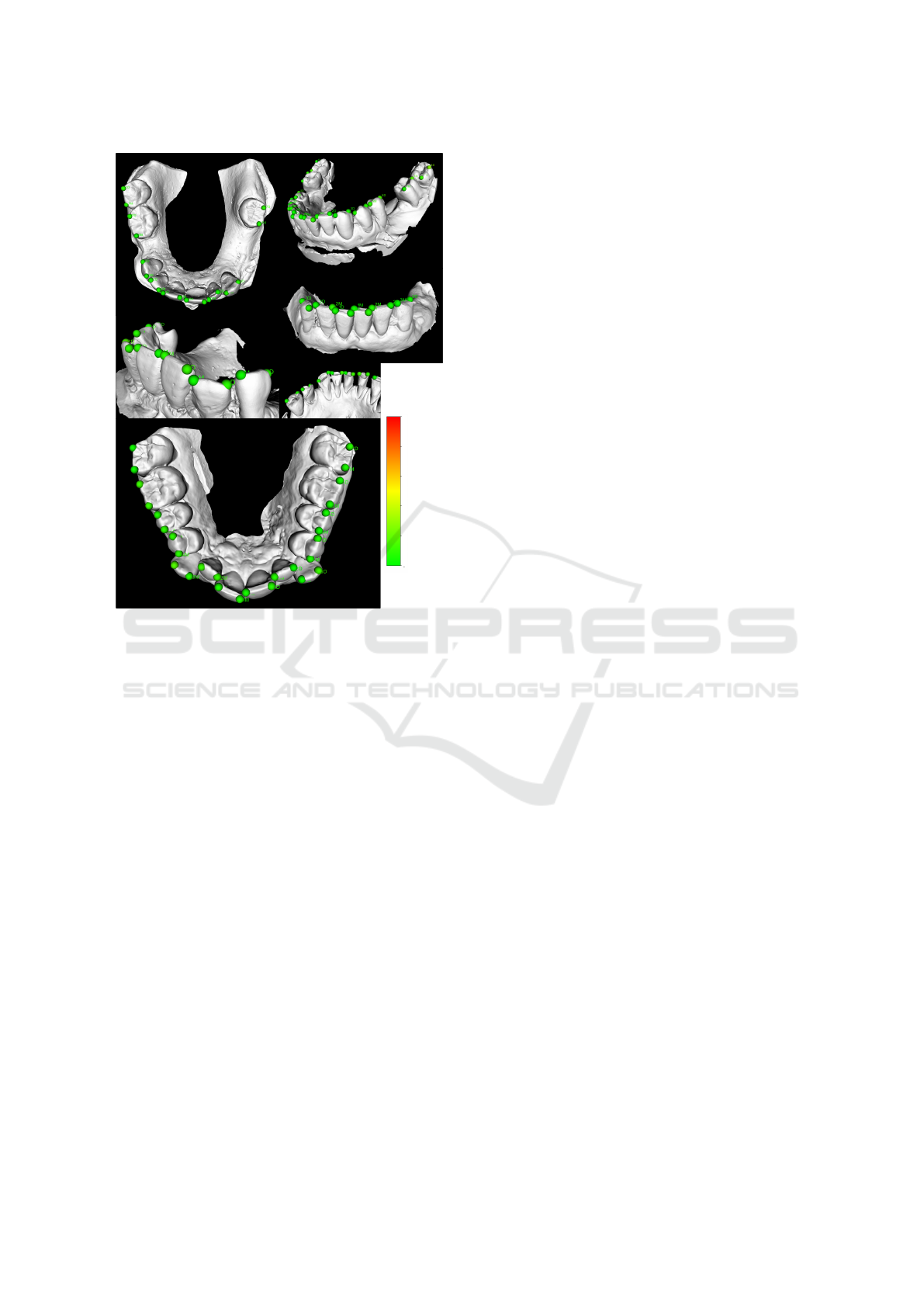
mm
2.0 mm
4.0 mm
6.0 mm
8.0 mm
10.0+ m
Figure 9: Examples of automatically detected landmarks
with our method. Majority of predictions have the landmark
localization error less than 2 mm. Our method correctly de-
tects if a tooth is missing and does not produce predictions
of corresponding landmarks.
6 CONCLUSIONS
The present findings confirm that the multi-view
approach combined with the RANSAC consensus
method brings promising results in the automation of
landmark detection. Evaluated on a dataset of real or-
thodontics dental casts with significant diversity, the
method performs the best with Attention U-Net archi-
tecture and with two-channeled input of depth maps
and geometry renders. This method setup achieves
a landmarking accuracy of 0.75 ± 0.96 mm.
Importantly, we have also shown that the uncer-
tainty measures based on the analysis of the max-
imum values of regressed heatmap predictions in
combination with multi-view uncertainty yield con-
venient information in the process of landmark pres-
ence detection. Combining these uncertainty mea-
sures, our method correctly detects landmark pres-
ence in 97.68% of cases. This means that the method
is suitable to be applied to data where landmarks’
presence is not granted. In addition, the method meets
the needs of clinical applications, as the inference at
the user’s side takes seconds to be calculated, even on
less powerful CPUs.
Even though the accuracies are satisfying, the size
of the dataset could not cover every bit of a maloc-
clusion case and teeth shifting. Future research could
examine the method on a larger dataset of dentition
with even more complex cases. Furthermore, future
studies should focus on the improvements in the in-
variance of rotation. The association between the ro-
tation from the aligned position and the landmarking
accuracy was investigated in this work, and it is the
main shortcoming of the proposed method.
ACKNOWLEDGEMENTS
This work was supported by TESCAN 3DIM, s.r.o.,
which provided us with the dataset used in this work
as well as with its funding.
REFERENCES
Cui, Y., Jia, M., Lin, T.-Y., Song, Y., and Belongie, S.
(2019). Class-balanced loss based on effective num-
ber of samples.
Donner, R., Menze, B. H., Bischof, H., and Langs, G.
(2013). Global localization of 3d anatomical struc-
tures by pre-filtered hough forests and discrete opti-
mization. Medical Image Analysis, 17(8):1304–1314.
Drevick
´
y, D. and Kodym, O. (2020). Evaluating deep learn-
ing uncertainty measures in cephalometric landmark
localization. In Proceedings of the 13th International
Joint Conference on Biomedical Engineering Systems
and Technologies - BIOIMAGING,, pages 213–220.
INSTICC, SciTePress.
Fischler, M. A. and Bolles, R. C. (1981). Random sample
consensus: A paradigm for model fitting with appli-
cations to image analysis and automated cartography.
Commun. ACM, 24(6):381–395.
Lv, J., Shao, X., Xing, J., Cheng, C., and Zhou, X.
(2017). A deep regression architecture with two-stage
re-initialization for high performance facial landmark
detection. In 2017 IEEE Conference on Computer Vi-
sion and Pattern Recognition, CVPR 2017, Honolulu,
HI, USA, July 21-26, 2017, pages 3691–3700. IEEE
Computer Society.
Normando, D. (2015). Third molars: To extract or not to ex-
tract? Dental press journal of orthodontics, 20(4):17–
18.
Paulsen, R. R., Juhl, K. A., Haspang, T. M., Hansen, T. F.,
Ganz, M., and Einarsson, G. (2018). Multi-view con-
sensus CNN for 3d facial landmark placement. In
Jawahar, C. V., Li, H., Mori, G., and Schindler, K.,
editors, Computer Vision - ACCV 2018 - 14th Asian
Conference on Computer Vision, Perth, Australia, De-
cember 2-6, 2018, Revised Selected Papers, Part I,
Robust Teeth Detection in 3D Dental Scans by Automated Multi-view Landmarking
33