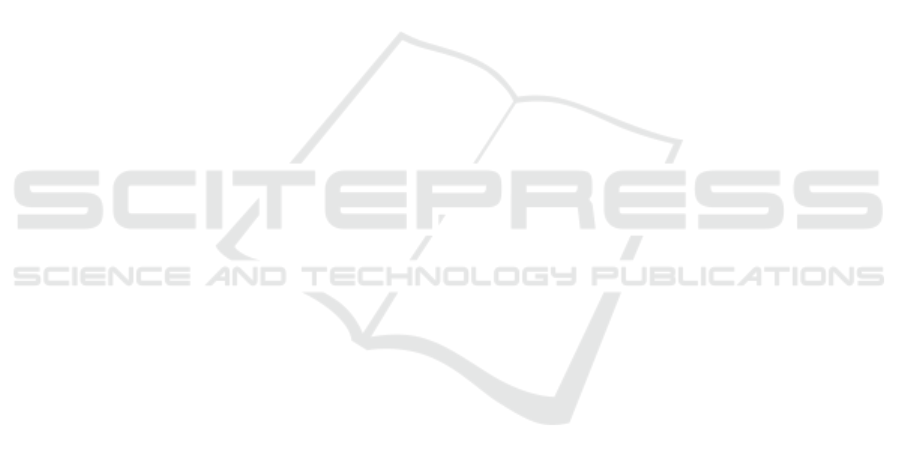
the segmentation of GGOs, whereas a larger patch
size of 32 was needed to accurately segment consol-
idations. Taken together, our findings suggest that
GAN models may be useful not only in data aug-
mentation tasks but also in image segmentation. Be-
yond COVID-19 imaging, our model can be adapted
to other medical applications, where the region of in-
terest is poorly defined and is very small compared
to other objects in the image. Future work will focus
on the experimental studies of denoising techniques
with the aim of improving the quality of the training
data sets and on the identification of most informative
training images.
ACKNOWLEDGMENT
The authors thank Cody Stevens for the assistance
with the execution of computational experiments and
the anonymous reviewers for comments that im-
proved this manuscript. The authors acknowledge
the Distributed Environment for Academic Comput-
ing (DEAC) at Wake Forest University for provid-
ing HPC resources that have contributed to the re-
search results reported within this paper. URL:
https://is.wfu.edu/deac
REFERENCES
Ai, T., Yang, Z., Hou, H., Zhan, C., Chen, C., Lv, W., Tao,
Q., Sun, Z., and Xia, L. (2020). Correlation of chest
CT and RT-PCR testing for coronavirus disease 2019
(COVID-19) in China: a report of 1014 cases. Radi-
ology, 296(2):E32–E40.
Cao, Y., Xu, Z., Feng, J., Jin, C., Han, X., Wu, H., and Shi,
H. (2020). Longitudinal assessment of COVID-19 us-
ing a deep learning–based quantitative CT pipeline:
illustration of two cases. Radiology: Cardiothoracic
Imaging, 2(2):e200082.
Drozdzal, M., Vorontsov, E., Chartrand, G., Kadoury, S.,
and Pal, C. (2016). The importance of skip connec-
tions in biomedical image segmentation. In Deep
Learning and Data Labeling for Medical Applica-
tions, pages 179–187. Springer.
Goel, T., Murugan, R., Mirjalili, S., and Chakrabartty, D. K.
(2021). Automatic screening of Covid-19 using an
optimized generative adversarial network. Cognitive
computation, pages 1–16.
Goodfellow, I., Pouget-Abadie, J., Mirza, M., Xu, B.,
Warde-Farley, D., Ozair, S., Courville, A., and Ben-
gio, Y. (2014). Generative adversarial nets. In
Advances in neural information processing systems,
pages 2672–2680.
Hesamian, M., Jia, W., He, X., and Kennedy, P. (2019).
Deep learning techniques for medical image segmen-
tation: Achievements and challenges. Journal of dig-
ital imaging, 32(4):582–596.
Huang, L., Han, R., Ai, T., Yu, P., Kang, H., Tao, Q., and
Xia, L. (2020). Serial quantitative chest CT assess-
ment of COVID-19: a deep learning approach. Radi-
ology: Cardiothoracic Imaging, 2(2):e200075.
Jiang, Y., Chen, H., Loew, M., and Ko, H. (2020). Covid-
19 CT image synthesis with a conditional generative
adversarial network. IEEE Journal of Biomedical and
Health Informatics, 25(2):441–452.
Kobayashi, Y. and Mitsudomi, T. (2013). Management
of ground-glass opacities: should all pulmonary le-
sions with ground-glass opacity be surgically re-
sected? Translational lung cancer research, 2(5):354.
Kumar Singh, V., Abdel-Nasser, M., Pandey, N., and Puig,
D. (2021). Lunginfseg: Segmenting COVID-19 in-
fected regions in lung CT images based on a receptive-
field-aware deep learning framework. Diagnostics,
11(2):158.
LeCun, Y., Bengio, Y., and Hinton, G. (2015). Deep learn-
ing. Nature, 521(7553):436–444.
Li, L., Qin, L., Xu, Z., Yin, Y., Wang, X., Kong, B., Bai, J.,
Lu, Y., Fang, Z., Song, Q., et al. (2020). Artificial in-
telligence distinguishes COVID-19 from community
acquired pneumonia on chest CT. Radiology.
Long, J., Shelhamer, E., and Darrell, T. (2015). Fully con-
volutional networks for semantic segmentation. In
Proceedings of the IEEE conference on computer vi-
sion and pattern recognition, pages 3431–3440.
Luc, P., Couprie, C., Chintala, S., and Verbeek, J. (2016).
Semantic segmentation using adversarial networks. In
NIPS Workshop on Adversarial Training.
MedSeg (2020). COVID-19 CT segmentation dataset.
https://medicalsegmentation.com/covid19/.
accessed on December 21, 2020.
Noh, H., Hong, S., and Han, B. (2015). Learning de-
convolution network for semantic segmentation. In
Proceedings of the IEEE international conference on
computer vision, pages 1520–1528.
O’Mahony, N., Campbell, S., Carvalho, A., Harapanahalli,
S., Hernandez, G. V., Krpalkova, L., Riordan, D., and
Walsh, J. (2019). Deep learning vs. traditional com-
puter vision. In Science and Information Conference,
pages 128–144. Springer.
Oulefki, A., Agaian, S., Trongtirakul, T., and Laouar, A.
(2020). Automatic COVID-19 lung infected region
segmentation and measurement using CT-scans im-
ages. Pattern Recognition, page 107747.
Rajamani, K. T., Siebert, H., and Heinrich, M. P. (2021).
Dynamic deformable attention network (DDANet) for
COVID-19 lesions semantic segmentation. Journal of
biomedical informatics, 119:103816.
Ronneberger, O., Fischer, P., and Brox, T. (2015). U-
Net: Convolutional networks for biomedical image
segmentation. In International Conference on Medi-
cal image computing and computer-assisted interven-
tion, pages 234–241. Springer.
Shan, F., Gao, Y., Wang, J., Shi, W., Shi, N., Han, M., Xue,
Z., Shen, D., and Shi, Y. (2021). Abnormal lung quan-
tification in chest CT images of COVID-19 patients
BIOINFORMATICS 2022 - 13th International Conference on Bioinformatics Models, Methods and Algorithms
36