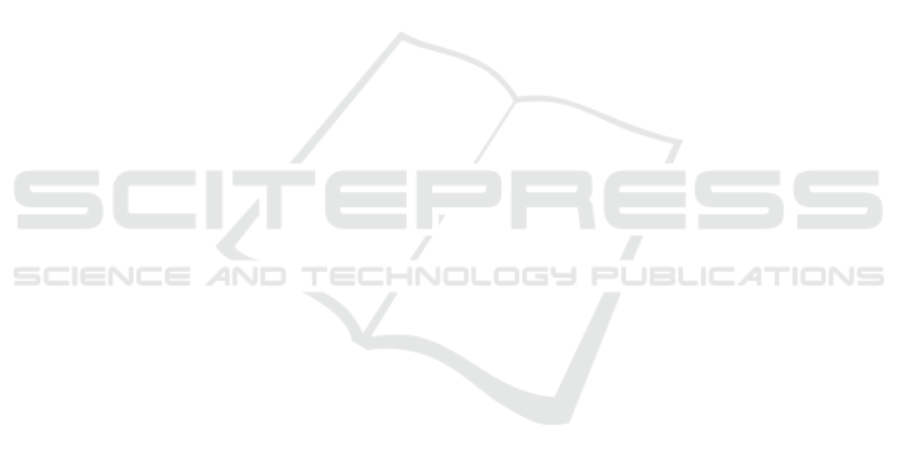
ity criterion (srqc): a super-resolution image quality
assessment metric. Multimedia Tools and Applica-
tions, 79(47):35125–35146.
Haris, M., Shakhnarovich, G., and Ukita, N. (2019).
Recurrent back-projection network for video super-
resolution. In Proceedings of the IEEE/CVF Con-
ference on Computer Vision and Pattern Recognition,
pages 3897–3906.
Isobe, T., Zhu, F., Jia, X., and Wang, S. (2020). Revisiting
temporal modeling for video super-resolution. arXiv
preprint arXiv:2008.05765.
Ji, X., Cao, Y., Tai, Y., Wang, C., Li, J., and Huang, F.
(2020). Real-world super-resolution via kernel esti-
mation and noise injection. In Proceedings of the
IEEE/CVF Conference on Computer Vision and Pat-
tern Recognition Workshops, pages 466–467.
K
¨
ohler, T., B
¨
atz, M., Naderi, F., Kaup, A., Maier, A., and
Riess, C. (2019). Toward bridging the simulated-to-
real gap: Benchmarking super-resolution on real data.
IEEE transactions on pattern analysis and machine
intelligence, 42(11):2944–2959.
Liu, S., Peng, X., and Liu, Z. (2019). Image quality as-
sessment through contour detection. In 2019 IEEE
28th International Symposium on Industrial Electron-
ics (ISIE), pages 1413–1417. IEEE.
Ma, C., Yang, C.-Y., Yang, X., and Yang, M.-H. (2017).
Learning a no-reference quality metric for single-
image super-resolution. Computer Vision and Image
Understanding, 158:1–16.
Ni, Z., Ma, L., Zeng, H., Chen, J., Cai, C., and Ma, K.-K.
(2017). Esim: Edge similarity for screen content im-
age quality assessment. IEEE Transactions on Image
Processing, 26(10):4818–4831.
Prewitt, J. M. (1970). Object enhancement and extraction.
Picture processing and Psychopictorics, 10(1):15–19.
Roberts, L. G. (1963). Machine perception of three-
dimensional solids. PhD thesis, Massachusetts Insti-
tute of Technology.
Shi, G., Wan, W., Wu, J., Xie, X., Dong, W., and Wu, H. R.
(2019). Sisrset: Single image super-resolution subjec-
tive evaluation test and objective quality assessment.
Neurocomputing, 360:37–51.
Tian, Y., Zhang, Y., Fu, Y., and Xu, C. (2020). Tdan:
Temporally-deformable alignment network for video
super-resolution. In Proceedings of the IEEE/CVF
Conference on Computer Vision and Pattern Recog-
nition, pages 3360–3369.
Wan, W., Wu, J., Shi, G., Li, Y., and Dong, W. (2018).
Super-resolution quality assessment: Subjective eval-
uation database and quality index based on perceptual
structure measurement. In 2018 IEEE International
Conference on Multimedia and Expo (ICME), pages
1–6. IEEE.
Wang, G., Li, L., Li, Q., Gu, K., Lu, Z., and Qian, J.
(2017). Perceptual evaluation of single-image super-
resolution reconstruction. In 2017 IEEE International
Conference on Image Processing (ICIP), pages 3145–
3149. IEEE.
Wang, G., Zhu, F., Lu, Z., Yuan, X., and Li, L.
(2018). No-reference quality assessment of super-
resolution reconstructed images by incorporating do-
main knowledge. J. Inf. Hiding Multim. Signal Pro-
cess., 9(2):496–505.
Wang, X., Xie, L., Dong, C., and Shan, Y. (2021).
Real-esrgan: Training real-world blind super-
resolution with pure synthetic data. arXiv preprint
arXiv:2107.10833.
Wang, Z., Bovik, A. C., Sheikh, H. R., and Simoncelli, E. P.
(2004). Image quality assessment: from error visi-
bility to structural similarity. IEEE transactions on
image processing, 13(4):600–612.
Wang, Z., Simoncelli, E. P., and Bovik, A. C. (2003). Mul-
tiscale structural similarity for image quality assess-
ment. In The Thrity-Seventh Asilomar Conference on
Signals, Systems & Computers, 2003, volume 2, pages
1398–1402. Ieee.
Xue, W. and Mou, X. (2011). An image quality assessment
metric based on non-shift edge. In 2011 18th IEEE
International Conference on Image Processing, pages
3309–3312. IEEE.
Yang, C.-Y., Ma, C., and Yang, M.-H. (2014). Single-image
super-resolution: A benchmark. In European confer-
ence on computer vision, pages 372–386. Springer.
Yang, L., Sheng, Y., and Chai, L. (2019). A machine
learning based reduced-reference image quality as-
sessment method for single-image super-resolution.
In 2019 Chinese Control Conference (CCC), pages
3571–3576. IEEE.
Yeganeh, H., Rostami, M., and Wang, Z. (2015). Ob-
jective quality assessment of interpolated natural im-
ages. IEEE Transactions on Image Processing,
24(11):4651–4663.
Zhang, K., Zhu, D., Li, J., Gao, X., Gao, F., and Lu, J.
(2021). Learning stacking regression for no-reference
super-resolution image quality assessment. Signal
Processing, 178:107771.
Zhang, R., Isola, P., Efros, A. A., Shechtman, E., and Wang,
O. (2018). The unreasonable effectiveness of deep
features as a perceptual metric. In Proceedings of
the IEEE conference on computer vision and pattern
recognition, pages 586–595.
Zhou, F., Yao, R., Liu, B., and Qiu, G. (2019). Visual qual-
ity assessment for super-resolved images: Database
and method. IEEE Transactions on Image Process-
ing, 28(7):3528–3541.
Zhou, W., Wang, Z., and Chen, Z. (2021). Image
super-resolution quality assessment: Structural fi-
delity versus statistical naturalness. arXiv preprint
arXiv:2105.07139.
VISAPP 2022 - 17th International Conference on Computer Vision Theory and Applications
322