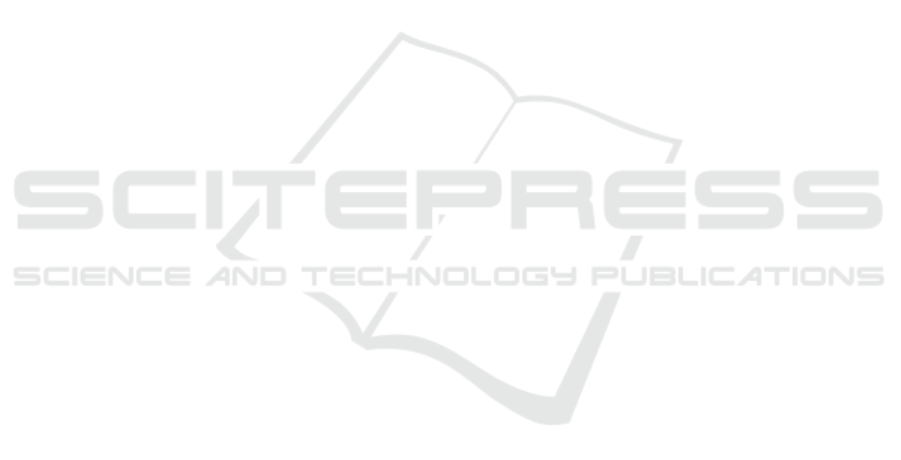
Fourure, D., Emonet, R., Fromont, E., et al. (2017). Resid-
ual conv-deconv grid network for semantic segmenta-
tion. In British Machine Vision Conf. (BMVC).
Gui, S., Wang, C., Chen, Q., and Tao, D. (2020). Fea-
tureflow: Robust video interpolation via structure-
to-texture generation. In IEEE. Int. Conf. on Com-
puter Vision and Pattern Recognition (CVPR), pages
14004–14013.
Hui, T., Tang, X., and Loy, C. C. (2018). Liteflownet: A
lightweight convolutional neural network for optical
flow estimation. In IEEE. Int. Conf. on Computer Vi-
sion and Pattern Recognition (CVPR), pages 8981–
8989.
Ilg, E., Mayer, N., Brox, T., et al. (2017). Flownet 2.0: Evo-
lution of optical flow estimation with deep networks.
In IEEE. Int. Conf. on Computer Vision and Pattern
Recognition (CVPR), pages 2462–2470.
Ilg, E., Saikia, T., Keuper, M., and Brox, T. (2018). Oc-
clusions, motion and depth boundaries with a generic
network for disparity, optical flow or scene flow esti-
mation. In Eu. Conf. on Computer Vision (ECCV).
Jaderberg, M., Simonyan, K., Zisserman, A., et al. (2015).
Spatial transformer networks. In Advances in Neural
Information Processing Systems (NIPS), pages 2017–
2025.
Jiang, H., Sun, D., Jampani, V., Kautz, J., et al. (2018).
Super slomo: High quality estimation of multiple in-
termediate frames for video interpolation. In IEEE.
Int. Conf. on Computer Vision and Pattern Recogni-
tion (CVPR), pages 9000–9008.
Liu, Z., A, R. Y., Tang, X., Liu, Y., and Agarwala, A.
(2017). Video frame synthesis using deep voxel flow.
In IEEE Int. Conf. on Computer Vision (ICCV), pages
4463–4471.
Meyer, S., Djelouah, A., McWilliams, B., Schroers, C.,
et al. (2018). Phasenet for video frame interpolation.
In IEEE. Int. Conf. on Computer Vision and Pattern
Recognition (CVPR), pages 498–507.
Meyer, S., Wang, O., Zimmer, H., Sorkine-Hornung, A.,
et al. (2015). Phase-based frame interpolation for
video. In IEEE. Int. Conf. on Computer Vision and
Pattern Recognition (CVPR), pages 1410–1418.
Niklaus, S. and Liu, F. (2018). Context-aware synthesis for
video frame interpolation. In IEEE. Int. Conf. on Com-
puter Vision and Pattern Recognition (CVPR), pages
1701–1710.
Niklaus, S. and Liu, F. (2020). Softmax splatting for video
frame interpolation. In IEEE. Int. Conf. on Computer
Vision and Pattern Recognition (CVPR), pages 5437–
5446.
Niklaus, S., Mai, L., and Liu, F. (2017a). Video frame inter-
polation via adaptive convolution. In IEEE. Int. Conf.
on Computer Vision and Pattern Recognition (CVPR),
pages 670–679.
Niklaus, S., Mai, L., and Liu, F. (2017b). Video frame
interpolation via adaptive separable convolution. In
IEEE Int. Conf. on Computer Vision (ICCV), pages
261–270.
Reda, F., Sun, D., Dundar, A., Shoeybi, M., Liu, G., Shih,
K., Tao, A., Kautz, J., and Catanzaro, B. (2019).
Unsupervised video interpolation using cycle consis-
tency. In IEEE. Int. Conf. on Computer Vision and
Pattern Recognition (CVPR), pages 892–900.
Shi, J., Jiang, X., and Guillemot, C. (2020). Learning fused
pixel and feature-based view reconstructions for light
fields. In IEEE. Int. Conf. on Computer Vision and
Pattern Recognition (CVPR), pages 2555–2564.
Soomro, K., Zamir, A. R., and Shah, M. (2012). Ucf101:
A dataset of 101 human actions classes from videos in
the wild. arXiv preprint arXiv:1212.0402.
Sun, D., Yang, X., Liu, M., and Kautz, J. (2018). Pwc-
net: Cnns for optical flow using pyramid, warping,
and cost volume. In IEEE. Int. Conf. on Computer
Vision and Pattern Recognition (CVPR), pages 8934–
8943.
Xu, X., Siyao, L., Sun, W., Yin, Q., and Yang, M. (2019).
Quadratic video interpolation. Advances in Neural In-
formation Processing Systems (NIPS).
Xue, J., Chen, B., T, W., et al. (2019). Video enhance-
ment with task-oriented flow. Int. J. Computer Vision
(IJCV), 127(8):1106–1125.
Deep Video Frame Rate Up-conversion Network using Feature-based Progressive Residue Refinement
339