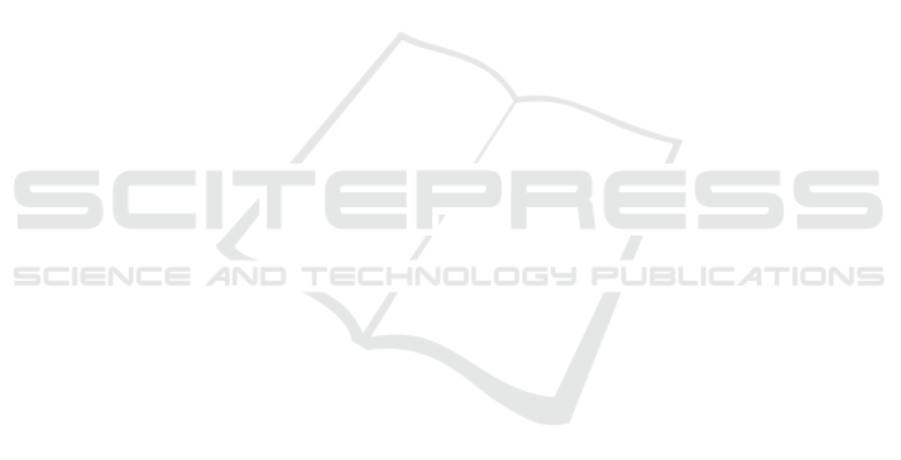
ral networks we obtain a mean error of −0.10 l and
0.09 l respectively. The method is so far equivalent to
the use of a global scaling factor.
In the future, we would like to further reduce the
error by using a larger data set and additional signal
features. The volume-time diagram can as well be
used to determine other respiration parameters. An
automatic selection and tracking of the region of in-
terest shall be implemented for a real world applica-
tion.
ACKNOWLEDGEMENTS
We would like to thank Dr. med. Sohrab for testing all
of our patients for health suitability and for advising
us from a medical perspective.
REFERENCES
Bernal, E. A., Mestha, L. K., and Shilla, E. (2014). Non
contact monitoring of respiratory function via depth
sensing. In 2014 IEEE-EMBS International Con-
ference on Biomedical and Health Informatics (BHI
2014), Piscataway, NJ. IEEE.
Cri
´
ee, C. P., Sorichter, S., Smith, H. J., Kardos, P., Mer-
get, R., Heise, D., Berdel, D., K
¨
ohler, D., Magnussen,
H., Marek, W., Mitfessel, H., Rasche, K., Rolke, M.,
Worth, H., and J
¨
orres, R. A. (2011). Body plethys-
mography – its principles and clinical use. Respira-
tory Medicine, 105(7):959–971.
de Boer, W., Lasenby, J., Cameron, J., Wareham, R., Ah-
mad, S., Roach, C., Hills, W., and Iles, R. (2010). Slp:
A zero-contact non-invasive method for pulmonary
function testing. In Labrosse, F., Zwiggelaar, R.,
Liu, Y., and Tiddeman, B., editors, Procedings of the
British Machine Vision Conference 2010, pages 85.1–
85.12. British Machine Vision Association.
ERS White Book (2012). The burden of lung disease.
Imano, W., Kameyama, K., Hollingdal, M., Refsgaard, J.,
Larsen, K., Topp, C., Kronborg, S. H., Gade, J. D., and
Dinesen, B. (2020). Non-contact respiratory measure-
ment using a depth camera for elderly people. Sensors,
20(23):6901.
Lim, S. H., Golkar, E., and Abd. Rahni, A. A. (2014).
Respiratory motion tracking using the kinect camera.
In 2014 IEEE Conference on Biomedical Engineering
and Sciences (IECBES), pages 797–800. IEEE.
Moore, V. C. (2012). Spirometry: step by step. Breathe,
8(3):232–240.
Oh, K., Shin, C. S., Kim, J., and Yoo, S. K. (2019). Level-
set segmentation-based respiratory volume estimation
using a depth camera. Ieee Journal of Biomedical and
Health Informatics, 23(4):1674–1682.
Ostadabbas, S., Sebkhi, N., Zhang, M., Rahim, S., Ander-
son, L. J., Lee, F. E.-H., and Ghovanloo, M. (2016).
A vision-based respiration monitoring system for pas-
sive airway resistance estimation. IEEE Transactions
on Biomedical Engineering, 63(9):1904–1913.
Sharp, C., Soleimani, V., Hannuna, S., Camplani, M.,
Damen, D., Viner, J., Mirmehdi, M., and Dodd, J. W.
(2017). Toward respiratory assessment using depth
measurements from a time-of-flight sensor. Frontiers
in Physiology, 8:65.
Soleimani, V., Mirmehdi, M., Damen, D., Dodd, J., Han-
nuna, S., Sharp, C., Camplani, M., and Viner, J.
(2017). Remote, depth-based lung function assess-
ment. IEEE Transactions on Biomedical Engineering,
64(8):1943–1958.
Soleimani, V., Mirmehdi, M., Damen, D., Hannuna, S.,
Camplani, M., Viner, J., and Dodd, J. (2015). Remote
pulmonary function testing using a depth sensor. In
2015 IEEE Biomedical Circuits and Systems Confer-
ence (BioCAS 2015), Piscataway, NJ. IEEE.
Wuerich, C., Wichum, F., Wiede, C., and Grabmaier, A.
(2021). Contactless optical respiration rate measure-
ment for a fast triage of sars-cov-2 patients in hospi-
tals. In Proceedings of the International Conference
on Image Processing and Vision Engineering, pages
29–35. SCITEPRESS - Science and Technology Pub-
lications.
BIOSIGNALS 2022 - 15th International Conference on Bio-inspired Systems and Signal Processing
158