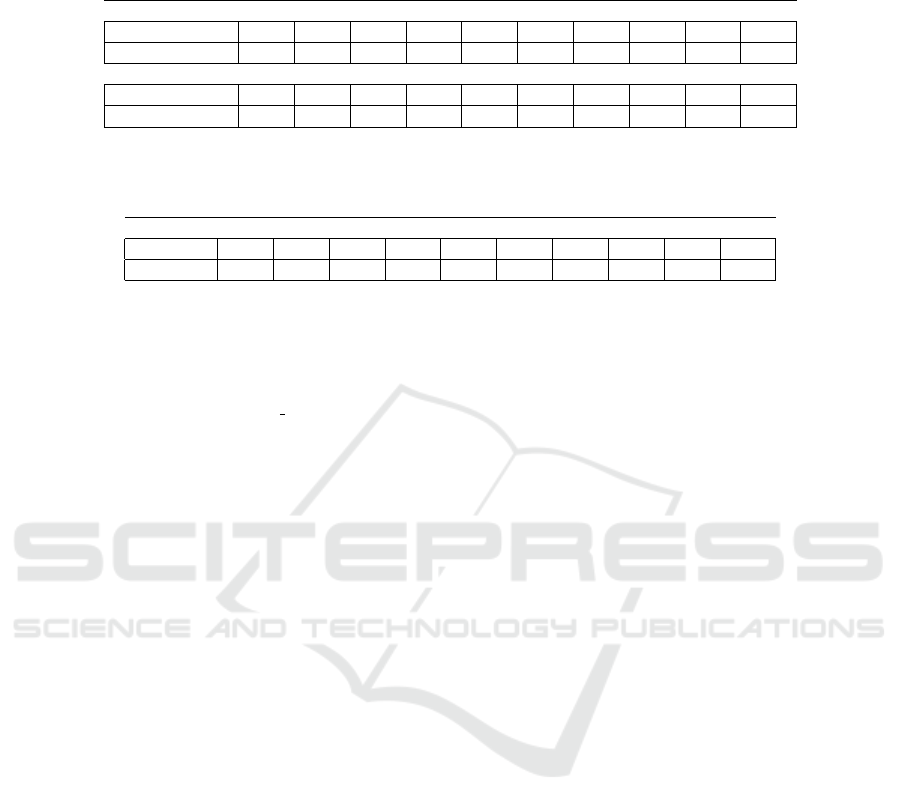
Table 3: Results on the estimation of rotation and translation. Each estimation was evaluated and compared against a set of
thresholds. Results given on the validation dataset from Apolloscape.
Total: 2245 images in validation set
Rotation - 18.0
o
average error
Error (degrees) 5
o
10
o
15
o
20
o
25
o
30
o
35
o
40
o
45
o
50
o
% of cars 73.3 80.7 82.8 85.5 86.2 86.1 86.7 87.0 87.2 87.5
Translation - 4.8m average error
Error (meters) 0.1 0.4 0.7 1.0 1.3 1.6 1.9 2.2 2.5 2.8
% of cars 0.09 2.6 8.0 14.4 21.7 27.1 33.2 38.4 43.5 48.0
Table 4: Results on the quality of the binary mask, where the score of each mask was compared with a set of thresholds.
Results given on the validation dataset from Apolloscape.
Total: 2403 images in validation set
Average IoU (Decoder) - 88%
IoU 0.95 0.9 0.85 0.8 0.75 0.7 0.65 0.60 0.55 0.5
% of cars 14.9 57.8 80.8 90.4 94.3 96.4 97.8 98.7 99.3 99.5
REFERENCES
Apolloscape (2021). Apolloscape, car instance - metric for-
mula. http://apolloscape.auto/car instance.html.
Chabot, F., Chaouch, M., Rabarisoa, J., Teuli
`
ere, C., and
Chateau, T. (2017). Deep manta: A coarse-to-fine
many-task network for joint 2d and 3d vehicle analy-
sis from monocular image. In 2017 IEEE Conference
on Computer Vision and Pattern Recognition (CVPR),
pages 1827–1836.
Chen, X., Kundu, K., Zhang, Z., Ma, H., Fidler, S., and
Urtasun, R. (2016). Monocular 3d object detection
for autonomous driving. In 2016 IEEE Conference
on Computer Vision and Pattern Recognition (CVPR),
pages 2147–2156.
Chen, X., Kundu, K., Zhu, Y., Berneshawi, A. G., Ma, H.,
Fidler, S., and Urtasun, R. (2015). 3d object propos-
als for accurate object class detection. In Cortes, C.,
Lawrence, N., Lee, D., Sugiyama, M., and Garnett,
R., editors, Advances in Neural Information Process-
ing Systems, volume 28, pages 424–432.
Fang, H., Gupta, S., Iandola, F., Srivastava, R., Deng, L.,
Dollar, P., Gao, J., He, X., Mitchell, M., Platt, J., Zit-
nick, L., and Zweig, G. (2015). From captions to vi-
sual concepts and back. In The proceedings of CVPR.
IEEE - Institute of Electrical and Electronics Engi-
neers.
Grenzd
¨
orffer, T., G
¨
unther, M., and Hertzberg, J. (2020).
Ycb-m: A multi-camera rgb-d dataset for object
recognition and 6dof pose estimation. In 2020 IEEE
International Conference on Robotics and Automation
(ICRA), pages 3650–3656.
Krizhevsky, A., Sutskever, I., and Hinton, G. (2012). Im-
agenet classification with deep convolutional neural
networks. In NIPS’12: Proceedings of the 25th Inter-
national Conference on Neural Information Process-
ing Systems, pages 1097–1105.
Kundu, A., Li, Y., and Rehg, J. M. (2018). 3d-rcnn:
Instance-level 3d object reconstruction via render-
and-compare. In 2018 IEEE/CVF Conference on
Computer Vision and Pattern Recognition, pages
3559–3568.
Li, Z., Yu, T., Pan, C., Zheng, Z., and Liu, Y. (2020). Robust
3d self-portraits in seconds. In 2020 IEEE/CVF Con-
ference on Computer Vision and Pattern Recognition
(CVPR), pages 1344–1353.
Liu, Z., Wu, Z., and T
´
oth, R. (2020). Smoke: Single-stage
monocular 3d object detection via keypoint estima-
tion. In 2020 IEEE/CVF Conference on Computer
Vision and Pattern Recognition Workshops (CVPRW),
pages 4289–4298.
Mousavian, A., Anguelov, D., Flynn, J., and Ko
ˇ
seck
´
a, J.
(2017). 3d bounding box estimation using deep learn-
ing and geometry. In 2017 IEEE Conference on Com-
puter Vision and Pattern Recognition (CVPR), pages
5632–5640.
Song, X., Wang, P., Zhou, D., Zhu, R., Guan, C., Dai, Y.,
Su, H., Li, H., and Yang, R. (2019). Apollocar3d:
A large 3d car instance understanding benchmark for
autonomous driving. In 2019 IEEE/CVF Conference
on Computer Vision and Pattern Recognition (CVPR),
pages 5447–5457.
Stegmann, M. B. and Gomez, D. D. (2002). A brief intro-
duction to statistical shape analysis.
Tan, V., Budvytis, I., and Cipolla, R. (2017). Indirect deep
structured learning for 3d human body shape and pose
prediction. In 2017 British Machine Vision Confer-
ence (BMVC).
Tatarchenko, M., Dosovitskiy, A., and Brox, T. (2016).
Multi-view 3d models from single images with a con-
volutional network. In European Conference on Com-
puter Vision, ECCV 2016, pages 322–337.
Wu, Y., Kirillov, A., Massa, F., Lo, W.-Y.,
and Girshick, R. (2019). Detectron2.
https://github.com/facebookresearch/detectron2.
Zou, W., Wu, D., Tian, S., Xiang, C., Li, X., and Zhang, L.
(2021). End-to-end 6dof pose estimation from monoc-
ular rgb images. IEEE Transactions on Consumer
Electronics, 67(1):87–96.
Zuffi, S., Kanazawa, A., Jacobs, D. W., and Black, M. J.
(2017). 3d menagerie: Modeling the 3d shape and
pose of animals. In 2017 IEEE Conference on Com-
puter Vision and Pattern Recognition (CVPR), pages
5524–5532.
VISAPP 2022 - 17th International Conference on Computer Vision Theory and Applications
396