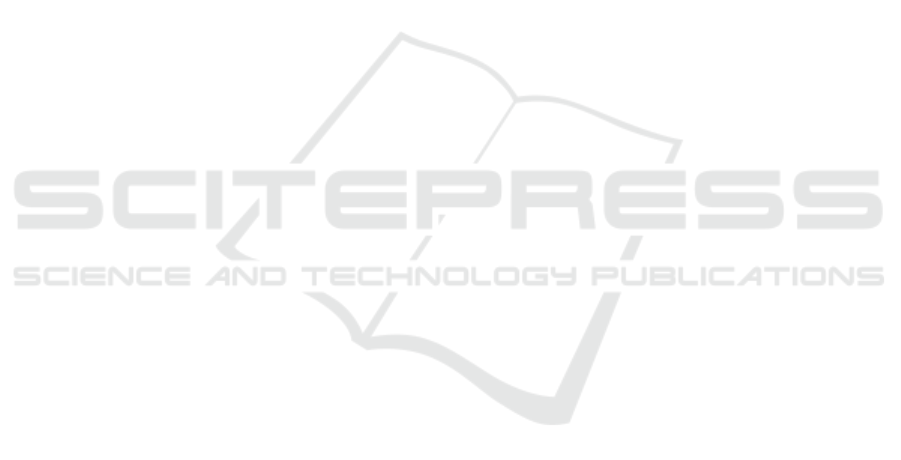
IEEE/CVF International Conference on Computer Vi-
sion (ICCV) Workshops, pages 2865–2874.
He, K., Zhang, X., Ren, S., and Sun, J. (2016). Deep resid-
ual learning for image recognition. In Proceedings of
the IEEE Conference on Computer Vision and Pattern
Recognition (CVPR).
Hidalgo, G., Raaj, Y., Idrees, H., Xiang, D., Joo, H., Simon,
T., and Sheikh, Y. (2019). Single-network whole-body
pose estimation. In Proceedings of the IEEE/CVF
International Conference on Computer Vision, pages
6982–6991.
Ke, L., Chang, M.-C., Qi, H., and Lyu, S. (2018). Multi-
scale structure-aware network for human pose esti-
mation. In Ferrari, V., Hebert, M., Sminchisescu,
C., and Weiss, Y., editors, Computer Vision – ECCV
2018, pages 731–746, Cham. Springer International
Publishing.
Krizhevsky, A., Sutskever, I., and Hinton, G. E. (2012).
Imagenet classification with deep convolutional neu-
ral networks. In Pereira, F., Burges, C. J. C., Bottou,
L., and Weinberger, K. Q., editors, Advances in Neu-
ral Information Processing Systems 25, pages 1097–
1105. Curran Associates, Inc.
Kumar, A., Alavi, A., and Chellappa, R. (2017). Kepler:
Keypoint and pose estimation of unconstrained faces
by learning efficient h-cnn regressors. In 2017 12th
IEEE International Conference on Automatic Face
Gesture Recognition (FG 2017), pages 258–265.
Kumar, A., Marks, T. K., Mou, W., Wang, Y., Jones, M.,
Cherian, A., Koike-Akino, T., Liu, X., and Feng, C.
(2020). Luvli face alignment: Estimating landmarks’
location, uncertainty, and visibility likelihood. In Pro-
ceedings of the IEEE/CVF Conference on Computer
Vision and Pattern Recognition, pages 8236–8246.
Li, W., Wang, Z., Yin, B., Peng, Q., Du, Y., Xiao, T., Yu,
G., Lu, H., Wei, Y., and Sun, J. (2019). Rethinking on
multi-stage networks for human pose estimation.
Lin, T.-Y., Doll
´
ar, P., Girshick, R., He, K., Hariharan, B.,
and Belongie, S. (2017). Feature pyramid networks
for object detection. In 2017 IEEE Conference on
Computer Vision and Pattern Recognition (CVPR),
pages 936–944.
Lin, T.-Y., Maire, M., Belongie, S., Bourdev, L., Girshick,
R., Hays, J., Perona, P., Ramanan, D., Zitnick, C. L.,
and Doll
´
ar, P. (2015). Microsoft coco: Common ob-
jects in context.
Newell, A., Huang, Z., and Deng, J. (2017). Associative
embedding: End-to-end learning for joint detection
and grouping. In Guyon, I., Luxburg, U. V., Ben-
gio, S., Wallach, H., Fergus, R., Vishwanathan, S., and
Garnett, R., editors, Advances in Neural Information
Processing Systems 30, pages 2277–2287. Curran As-
sociates, Inc.
Newell, A., Yang, K., and Deng, J. (2016). Stacked Hour-
glass Networks for Human Pose Estimation. In Leibe,
B., Matas, J., Sebe, N., and Welling, M., editors, Com-
puter Vision – ECCV 2016, pages 483–499, Cham.
Springer International Publishing.
Nie, X., Feng, J., Xing, J., and Yan, S. (2018). Pose partition
networks for multi-person pose estimation. In Com-
puter Vision – ECCV 2018, pages 684–699, Cham.
Springer International Publishing.
Stoffl, L., Vidal, M., and Mathis, A. (2021). End-to-end
trainable multi-instance pose estimation with trans-
formers.
Sun, K., Xiao, B., Liu, D., and Wang, J. (2019). Deep high-
resolution representation learning for human pose es-
timation. In 2019 IEEE/CVF Conference on Com-
puter Vision and Pattern Recognition (CVPR), pages
5686–5696.
Sun, X., Shang, J., Liang, S., and Wei, Y. (2017). Composi-
tional human pose regression. In 2017 IEEE Interna-
tional Conference on Computer Vision (ICCV), pages
2621–2630.
Szegedy, C., Liu, W., Jia, Y., Sermanet, P., Reed, S.,
Anguelov, D., Erhan, D., Vanhoucke, V., and Rabi-
novich, A. (2015). Going deeper with convolutions.
In Proceedings of the IEEE Conference on Computer
Vision and Pattern Recognition (CVPR).
Tan, M. and Le, Q. (2019). Efficientnet: Rethinking model
scaling for convolutional neural networks. In Interna-
tional Conference on Machine Learning, pages 6105–
6114. PMLR.
Tang, W. and Wu, Y. (2019). Does learning specific fea-
tures for related parts help human pose estimation? In
2019 IEEE/CVF Conference on Computer Vision and
Pattern Recognition (CVPR), pages 1107–1116.
Tang, W., Yu, P., and Wu, Y. (2018). Deeply learned compo-
sitional models for human pose estimation. In Ferrari,
V., Hebert, M., Sminchisescu, C., and Weiss, Y., edi-
tors, Computer Vision – ECCV 2018, pages 197–214,
Cham. Springer International Publishing.
Toshev, A. and Szegedy, C. (2014). Deeppose: Human pose
estimation via deep neural networks. In 2014 IEEE
Conference on Computer Vision and Pattern Recogni-
tion, pages 1653–1660.
Xiao, B., Wu, H., and Wei, Y. (2018). Simple baselines
for human pose estimation and tracking. In Ferrari,
V., Hebert, M., Sminchisescu, C., and Weiss, Y., edi-
tors, Computer Vision – ECCV 2018, pages 472–487,
Cham. Springer International Publishing.
Zhao, L., Xu, J., Zhang, S., Gong, C., Yang, J., and Gao,
X. (2020a). Perceiving heavily occluded human poses
by assigning unbiased score. Information Sciences,
537:284–301.
Zhao, M., Beurier, G., Wang, H., and Wang, X. (2020b).
A pipeline for creating in-vehicle posture database
for developing driver posture monitoring systems.
In DHM2020: Proceedings of the 6th International
Digital Human Modeling Symposium, August 31-
September 2, 2020, volume 11, pages 187–196. IOS
Press.
VISAPP 2022 - 17th International Conference on Computer Vision Theory and Applications
436