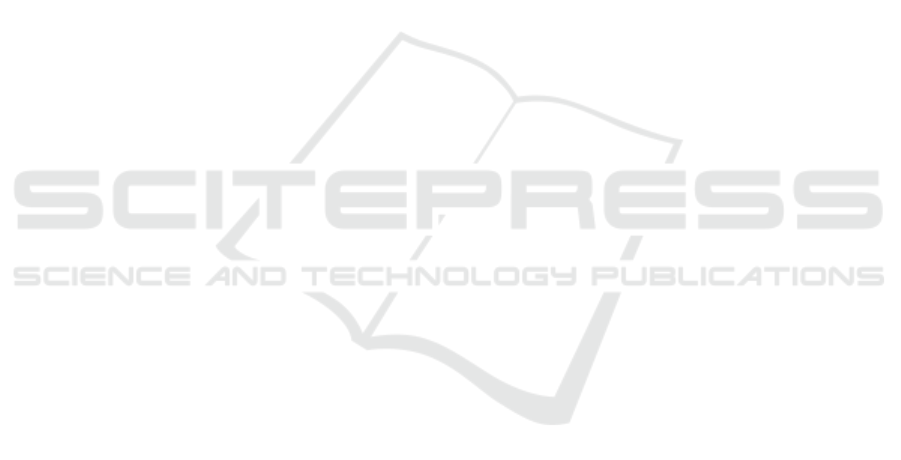
scheme does not require manual annotation, allowing
for additional few steps of fine tuning without manual
intervention for a better adaptation of the CNN model
for the sequence specificity without loss of generality
of the approach.
4 CONCLUSIONS AND FUTURE
WORKS
In this work, we propose the first deep learning
based approach for endoscopic image matching. For
this purpose, a triplet-based dataset of image patches
triplet has been firstly constructed. After that, to train
the CNN, an adaptive triplet loss has been designed to
improve the inter-class separability as well as the inter
class compactness leading to a discriminative feature
space. We assessed the robustness of the proposed ap-
proach against viewpoint changes and compared the
obtained performances to SIFT, one of the most suc-
cessful state-of-art descriptors.
Our further work will be focused on exploring
graph neural networks to integrate neighboring image
interest points in order to improve the discriminative
ability of the model. We intend also to test our ap-
proach on other endoscopic data captured for different
organs.
REFERENCES
Ait-Aoudia, S., Mahiou, R., Djebli, H., and Guerrout, E.-
H. (2012). Satellite and Aerial Image Mosaicing - A
Comparative Insight. In 2012 16th International Con-
ference on Information Visualisation, pages 652–657,
Montpellier, France. IEEE.
Ali, S., Zhou, F., Bailey, A., Braden, B., East, J. E., Lu,
X., and Rittscher, J. (2021). A deep learning frame-
work for quality assessment and restoration in video
endoscopy. Medical Image Analysis, 68:101900. Pub-
lisher: Elsevier.
Bay, H., Ess, A., Tuytelaars, T., and Van Gool, L. (2008).
Speeded-Up Robust Features (SURF). Computer Vi-
sion and Image Understanding, 110(3):346–359.
Behrens, A., Bommes, M., Stehle, T., Gross, S., Leonhardt,
S., and Aach, T. (2010). Real-time image composition
of bladder mosaics in fluorescence endoscopy. Com-
puter Science - Research and Development.
Behrens, A., Stehle, T., Gross, S., and Aach, T. (2009).
Local and global panoramic imaging for fluorescence
bladder endoscopy. In 2009 Annual International
Conference of the IEEE Engineering in Medicine and
Biology Society, pages 6990–6993. IEEE.
Ben-Hamadou, A., Daul, C., and Soussen, C. (2016). Con-
struction of extended 3d field of views of the inter-
nal bladder wall surface: A proof of concept. 3D Re-
search, 7(3):1–23.
Bergen, T., Wittenberg, T., M
¨
unzenmayer, C., Chen, C.
C. G., and Hager, G. D. (2013). A graph-based ap-
proach for local and global panorama imaging in cys-
toscopy. In Medical Imaging 2013: Image-Guided
Procedures, Robotic Interventions, and Modeling,
volume 8671, page 86711K. International Society for
Optics and Photonics.
Daul, C., Blondel, W., Ben-Hamadou, A., Miranda-Luna,
R., Soussen, C., Wolf, D., and Guillemin, F. (2010).
From 2d towards 3d cartography of hollow organs. In
2010 7th International Conference on Electrical En-
gineering Computing Science and Automatic Control,
pages 285–293. IEEE.
Du, P., Zhou, Y., Xing, Q., and Hu, X. (2011). Improved
SIFT matching algorithm for 3D reconstruction from
endoscopic images. In Proceedings of the 10th In-
ternational Conference on Virtual Reality Continuum
and Its Applications in Industry, pages 561–564.
Elibol, A., Kim, J., Gracias, N., and Garcia, R. (2017). Fast
Underwater Image Mosaicing through Submapping.
Journal of Intelligent & Robotic Systems, 85(1):167–
187.
Fischler, M. A. and Bolles, R. C. (1981). Random sample
consensus: a paradigm for model fitting with appli-
cations to image analysis and automated cartography.
Communications of the ACM, 24(6):381–395. Pub-
lisher: ACM New York, NY, USA.
Ghosh, T., Li, L., and Chakareski, J. (2018). Effective
deep learning for semantic segmentation based bleed-
ing zone detection in capsule endoscopy images. In
2018 25th IEEE International Conference on Image
Processing (ICIP), pages 3034–3038. IEEE.
Hossein-Nejad, Z. and Nasri, M. (2018). A-RANSAC:
Adaptive random sample consensus method in mul-
timodal retinal image registration. Biomedical Signal
Processing and Control, 45:325–338. Publisher: El-
sevier.
Kim, Y. J., Bae, J. P., Chung, J.-W., Park, D. K., Kim, K. G.,
and Kim, Y. J. (2021). New polyp image classifica-
tion technique using transfer learning of network-in-
network structure in endoscopic images. Scientific Re-
ports, 11(1):1–8. Publisher: Nature Publishing Group.
Kumar, P., Jain, S., Raman, B., Roy, P. P., and Iwamura,
M. (2021). End-to-end Triplet Loss based Emotion
Embedding System for Speech Emotion Recognition.
In 2020 25th International Conference on Pattern
Recognition (ICPR), pages 8766–8773. IEEE.
Lai, S.-C., Kong, M., Lam, K.-M., and Li, D. (2019).
High-resolution face recognition via deep pore-feature
matching. In 2019 IEEE International Conference on
Image Processing (ICIP), pages 3477–3481. IEEE.
Lee, M. H. and Park, I. K. (2014). Performance evaluation
of local descriptors for affine invariant region detector.
In Asian Conference on Computer Vision, pages 630–
643. Springer.
Li, Z., Sang, N., Chen, K., Gao, C., and Wang, R. (2018).
Learning deep features with adaptive triplet loss for
person reidentification. In MIPPR 2017: Pattern
Deep Features Extraction for Endoscopic Image Matching
931