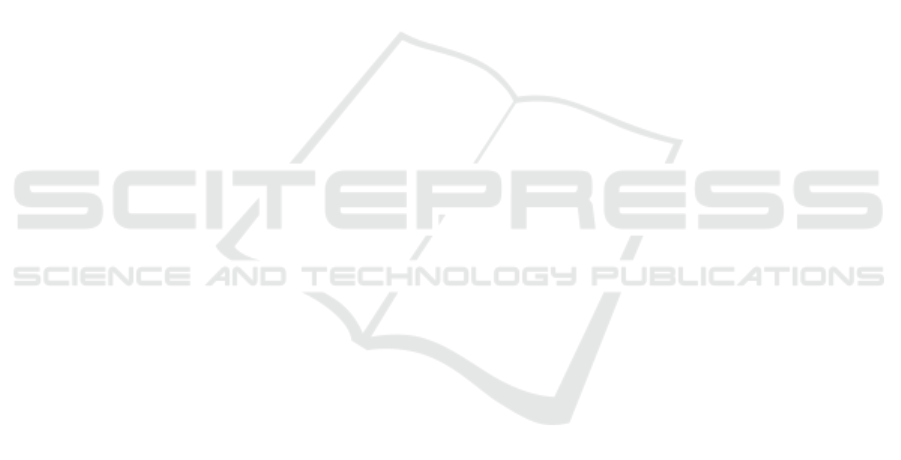
REFERENCES
Aamir, S. A., Stradmann, Y., M
¨
uller, P., Pehle, C., Hartel,
A., Gr
¨
ubl, A., Schemmel, J., and Meier, K. (2018).
An accelerated lif neuronal network array for a large-
scale mixed-signal neuromorphic architecture. IEEE
Transactions on Circuits and Systems I: Regular Pa-
pers, 65(12):4299–4312.
Akopyan, F., Sawada, J., Cassidy, A., Alvarez-Icaza, R.,
Arthur, J., Merolla, P., Imam, N., Nakamura, Y., Datta,
P., Nam, G.-J., Taba, B., Beakes, M., Brezzo, B.,
Kuang, J. B., Manohar, R., Risk, W. P., Jackson,
B., and Modha, D. S. (2015). TrueNorth: Design
and Tool Flow of a 65 mW 1 Million Neuron Pro-
grammable Neurosynaptic Chip. IEEE Transactions
on Computer-Aided Design of Integrated Circuits and
Systems, 34(10):1537–1557. Conference Name: IEEE
Transactions on Computer-Aided Design of Integrated
Circuits and Systems.
Asghar, M. S., Arslan, S., and Kim, H. (2021). A low-power
spiking neural network chip based on a compact lif
neuron and binary exponential charge injector synapse
circuits. Sensors, 21(13):4462.
Bi, G.-q. and Poo, M.-m. (1998). Synaptic Modifications
in Cultured Hippocampal Neurons: Dependence on
Spike Timing, Synaptic Strength, and Postsynaptic
Cell Type. Journal of Neuroscience, 18(24):10464–
10472. Publisher: Society for Neuroscience Section:
ARTICLE.
Bichler, O., Querlioz, D., Thorpe, S. J., Bourgoin, J.-P.,
and Gamrat, C. (2012). Extraction of temporally
correlated features from dynamic vision sensors with
spike-timing-dependent plasticity. Neural Networks,
32:339–348.
Brandli, C., Berner, R., Yang, M., Liu, S.-C., and Delbruck,
T. (2014). A 240 × 180 130 db 3 µs latency global
shutter spatiotemporal vision sensor. IEEE Journal of
Solid-State Circuits, 49(10):2333–2341.
Chauhan, T., Masquelier, T., Montlibert, A., and Cottereau,
B. R. (2018). Emergence of Binocular Disparity Se-
lectivity through Hebbian Learning. Journal of Neu-
roscience, 38(44):9563–9578. Publisher: Society for
Neuroscience Section: Research Articles.
Dan, Y. and Poo, M.-m. (2004). Spike Timing-Dependent
Plasticity of Neural Circuits. Neuron, 44(1):23–30.
Davies, M., Srinivasa, N., Lin, T.-H., Chinya, G., Cao, Y.,
Choday, S. H., Dimou, G., Joshi, P., Imam, N., Jain,
S., Liao, Y., Lin, C.-K., Lines, A., Liu, R., Math-
aikutty, D., McCoy, S., Paul, A., Tse, J., Venkatara-
manan, G., Weng, Y.-H., Wild, A., Yang, Y., and
Wang, H. (2018). Loihi: A Neuromorphic Many-
core Processor with On-Chip Learning. IEEE Micro,
38(1):82–99. Conference Name: IEEE Micro.
Debat, G., Chauhan, T., Cottereau, B. R., Masquelier, T.,
Paindavoine, M., and Baures, R. (2021). Event-based
trajectory prediction using spiking neural networks.
Frontiers in Computational Neuroscience, 15:47.
Diehl, P. U. and Cook, M. (2015). Unsupervised learning of
digit recognition using spike-timing-dependent plas-
ticity. Front. Comput. Neurosci.
Gallego, G. and Scaramuzza, D. (2017). Accurate angu-
lar velocity estimation with an event camera. IEEE
Robotics and Automation Letters, 2(2):632–639.
Gerstner, W. and Kistler, W. M. (2002). Spiking Neu-
ron Models: Single Neurons, Populations, Plasticity.
Cambridge University Press, Cambridge.
Indiveri, G., Chicca, E., and Douglas, R. (2006). A
vlsi array of low-power spiking neurons and bistable
synapses with spike-timing dependent plasticity.
IEEE Transactions on Neural Networks, 17(1):211–
221.
Lakshmi, A., Chakraborty, A., and Thakur, C. S. (2019).
Neuromorphic vision: From sensors to event-based
algorithms. WIREs Data Mining and Knowledge Dis-
covery, 9(4):e1310.
Lee, C., Kosta, A. K., Zhu, A. Z., Chaney, K., Daniilidis, K.,
and Roy, K. (2020). Spike-flownet: event-based opti-
cal flow estimation with energy-efficient hybrid neural
networks. In European Conference on Computer Vi-
sion, pages 366–382. Springer.
Markram, H., L
¨
ubke, J., Frotscher, M., and Sakmann, B.
(1997). Regulation of synaptic efficacy by coinci-
dence of postsynaptic APs and EPSPs. Science (New
York, N.Y.), 275(5297):213–215.
Mink, J. W., Blumenschine, R. J., and Adams, D. B. (1981).
Ratio of central nervous system to body metabolism
in vertebrates: its constancy and functional basis. The
American Journal of Physiology, 241(3):R203–212.
Mueggler, E., Rebecq, H., Gallego, G., Delbruck, T., and
Scaramuzza, D. (2017). The event-camera dataset and
simulator: Event-based data for pose estimation, vi-
sual odometry, and SLAM. The International Jour-
nal of Robotics Research, 36(2):142–149. Publisher:
SAGE Publications Ltd STM.
Neftci, E. O., Mostafa, H., and Zenke, F. (2019). Surro-
gate Gradient Learning in Spiking Neural Networks:
Bringing the Power of Gradient-Based Optimization
to Spiking Neural Networks. IEEE Signal Process-
ing Magazine, 36(6):51–63. Conference Name: IEEE
Signal Processing Magazine.
Neil, D. and Liu, S.-C. (2016). Effective sensor fusion with
event-based sensors and deep network architectures.
In 2016 IEEE International Symposium on Circuits
and Systems (ISCAS), pages 2282–2285. ISSN: 2379-
447X.
Nguyen, A., Do, T.-T., Caldwell, D. G., and Tsagarakis,
N. G. (2019). Real-time 6dof pose relocalization for
event cameras with stacked spatial lstm networks. In
Proceedings of the IEEE/CVF Conference on Com-
puter Vision and Pattern Recognition (CVPR) Work-
shops.
Oudjail., V. and Martinet., J. (2019). Bio-inspired event-
based motion analysis with spiking neural networks.
In Proceedings of the 14th International Joint Con-
ference on Computer Vision, Imaging and Computer
Graphics Theory and Applications - Volume 4: VIS-
APP,, pages 389–394. INSTICC, SciTePress.
Oudjail., V. and Martinet., J. (2020). Meta-parameters ex-
ploration for unsupervised event-based motion anal-
ysis. In Proceedings of the 15th International Joint
Event-based Extraction of Navigation Features from Unsupervised Learning of Optic Flow Patterns
709