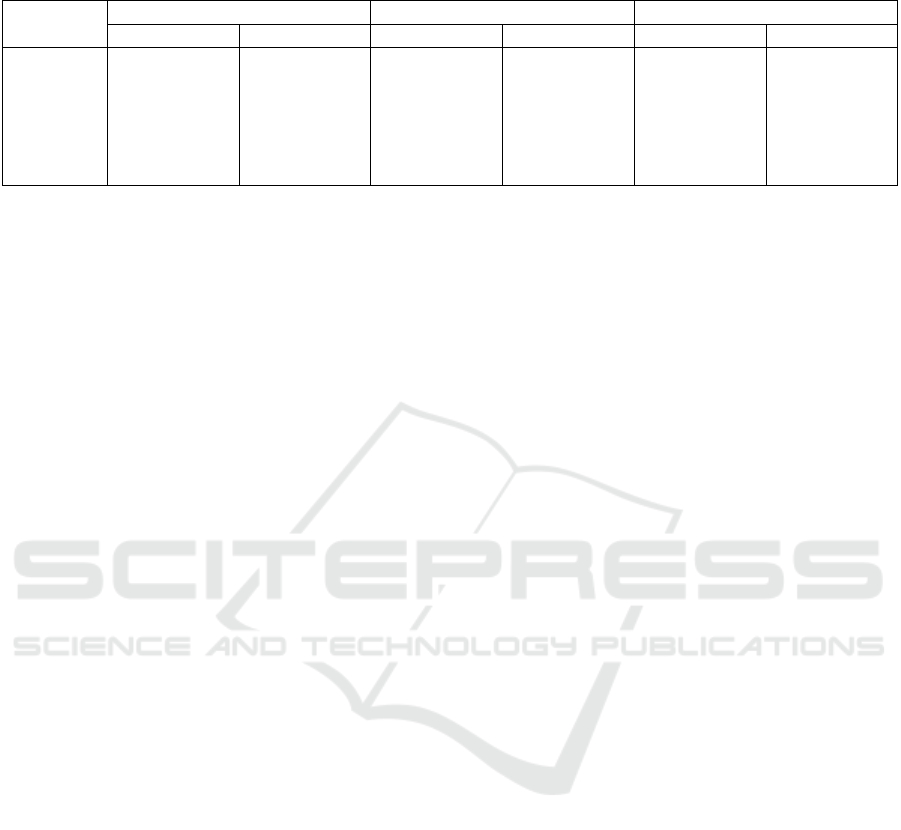
Table 3: Effect of the amount of training data in the short-term training protocol with triplet loss and augmentation on CMC
performance metric at rank-1 and rank-3.
# tracks
Same day, same hour Diff day, same hour Diff day, any hour
Rank 1 Rank 3 Rank 1 Rank 3 Rank 1 Rank 3
181 0.183 0.397 0.112 0.322 0.106 0.305
362 0.315 0.578 0.239 0.463 0.186 0.408
724 0.392 0.676 0.249 0.534 0.251 0.520
1448 0.548 0.795 0.422 0.688 0.378 0.655
2896 0.663 0.884 0.504 0.774 0.421 0.725
4949 0.680 0.885 0.538 0.814 0.480 0.761
These results show the possibility to recognize
honeybees amongst a gallery of distractors over multi-
ple days using only images of their abdomen. Future
work will consider how the performance of such an
approach would be improved with lightweight mark-
ings such as paint, by considering full-body images
and by further increasing the scale of automatically
collected training datasets, which could yield practi-
cal ways to track larger number of individuals over
multiple hours and days without heavy marking pro-
cedures.
ACKNOWLEDGMENTS
This work is supported by grant no. 2021-67014-
34999 from the USDA National Institute of Food
and Agriculture. This material is based upon work
supported by the National Science Foundation under
grants no. 1707355 and 1633184. J. C. acknowledges
support from the PR-LSAMP Bridge to the Doctor-
ate, a program from the NSF under award number
HRD-1906130. T. G. acknowledges NSF-HRD award
#1736019 that provided funds for the purchase of
bees. This work used the High-Performance Comput-
ing facility (HPCf) of the University of Puerto Rico,
supported by National Institute of General Medical
Sciences, National Institutes of Health (NIH) award
number P20GM103475 and NSF grants number EPS-
1002410 and EPS-1010094. This work used the Ex-
treme Science and Engineering Discovery Environ-
ment (XSEDE), which is supported by National Sci-
ence Foundation grant number ACI-1548562. Specif-
ically, it used the Bridges-2 system, which is sup-
ported by NSF award number ACI-1928147, at the
Pittsburgh Supercomputing Center (PSC).
REFERENCES
Bergamini, L., Porrello, A., Dondona, A. C., Negro, E. D.,
Mattioli, M., D’alterio, N., and Calderara, S. (2018).
Multi-views Embedding for Cattle Re-identification.
In 2018 14th International Conference on Signal-
Image Technology & Internet-Based Systems (SITIS),
pages 184–191.
Boenisch, F., Rosemann, B., Wild, B., Dormagen, D.,
Wario, F., and Landgraf, T. (2018). Tracking All
Members of a Honey Bee Colony Over Their Lifetime
Using Learned Models of Correspondence. Frontiers
in Robotics and AI, 5:35.
Bozek, K., Hebert, L., Portugal, Y., and Stephens, G. J.
(2021). Markerless tracking of an entire honey bee
colony. Nature Communications, 12(1):1733.
Brust, C.-A., Burghardt, T., Groenenberg, M., Kading, C.,
Kuhl, H. S., Manguette, M. L., and Denzler, J. (2017).
Towards automated visual monitoring of individual
gorillas in the wild. In Proceedings of the IEEE Inter-
national Conference on Computer Vision Workshops,
pages 2820–2830.
Chen, T., Kornblith, S., Norouzi, M., and Hinton, G.
(2020a). A simple framework for contrastive learn-
ing of visual representations. Proceedings of the
37th International Conference on Machine Learning,
119:1597–1607.
Chen, T., Kornblith, S., Swersky, K., Norouzi, M., and Hin-
ton, G. E. (2020b). Big Self-Supervised Models are
Strong Semi-Supervised Learners. In Larochelle, H.,
Ranzato, M., Hadsell, R., Balcan, M. F., and Lin, H.,
editors, Advances in Neural Information Processing
Systems, volume 33, pages 22243–22255. Curran As-
sociates, Inc.
Deb, D., Wiper, S., Gong, S., Shi, Y., Tymoszek, C.,
Fletcher, A., and Jain, A. K. (2018). Face recogni-
tion: Primates in the wild. In 2018 IEEE 9th Interna-
tional Conference on Biometrics Theory, Applications
and Systems (BTAS), pages 1–10. IEEE.
Gao, J., Burghardt, T., Andrew, W., Dowsey, A. W.,
and Campbell, N. W. (2021). Towards Self-
Supervision for Video Identification of Individual
Holstein-Friesian Cattle: The Cows2021 Dataset.
arXiv preprint arXiv:2105.01938.
He, K., Zhang, X., Ren, S., and Sun, J. (2016). Iden-
tity Mappings in Deep Residual Networks BT - Com-
puter Vision – ECCV 2016. pages 630–645, Cham.
Springer International Publishing.
K
¨
orschens, M., Barz, B., and Denzler, J. (2018). Towards
automatic identification of elephants in the wild. arXiv
preprint arXiv:1812.04418.
Li, S., Li, J., Lin, W., and Tang, H. (2019). Amur
tiger re-identification in the wild. arXiv preprint
arXiv:1906.05586.
VISAPP 2022 - 17th International Conference on Computer Vision Theory and Applications
524