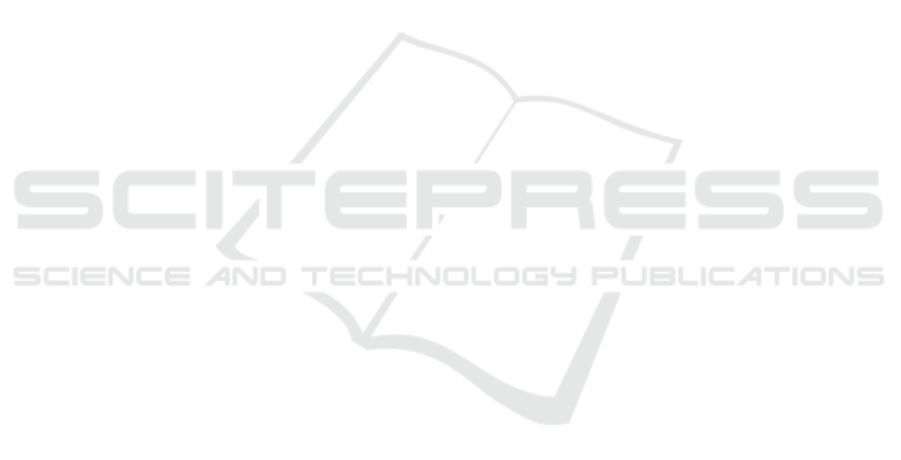
where Creative Professionals select the GT more of-
ten than AMT Workers. The examples in the two bot-
tom rows of Figure 7) suggest that AMT Workers di-
verge from GT based on consistency of color or im-
age composition. And overall, the global responses of
Creative Professionals are biased towards the Ground
Truth (38.89% versus 47, 4%). We believe these two
discrepancies can be best explained due to a differ-
ence in criterion, for particular cases: in examples
when one of the two options shows a similar cam-
era angle and content as the reference image AMT
Workers tend to select it, while Creative Professionals
usually choose the one that provides more diversity of
shots.
Further work exploring automatic viewpoint anal-
ysis should be done to clarify this possibility, and use
it to improve the next-shot prediction module. Fur-
ther work should also explore whether the combina-
tion of the shot-duration and the next-shot prediction
produces results that are more or less consistent with
subjective preferences. Further directions to explore
are to take advantage of features from the audio for
both modules, as well as to enrich the shot selection
process.
In conclusion, subjective and objective metrics
provide evidence that our next-shot prediction mod-
ule performs reasonable predictions, quite consistent
with the criteria of both AMT Workers and Creative
Professionals. We also showed that the accuracy met-
ric alone is not reliable, subjective metrics must also
be considered.
REFERENCES
Arev, I., Park, H. S., Sheikh, Y., Hodgins, J., and Shamir,
A. (2014). Automatic editing of footage from multi-
ple social cameras. ACM Transactions on Graphics
(TOG), 33(4):1–11.
Berthouzoz, F., Li, W., and Agrawala, M. (2012). Tools for
placing cuts and transitions in interview video. ACM
Transactions on Graphics (TOG), 31(4):1–8.
Chen, J., Meng, L., and Little, J. J. (2018). Camera se-
lection for broadcasting soccer games. In 2018 IEEE
Winter Conference on Applications of Computer Vi-
sion (WACV), pages 427–435. IEEE.
Chollet, F. (2017). Xception: Deep learning with depthwise
separable convolutions. In Proceedings of the IEEE
conference on computer vision and pattern recogni-
tion, pages 1251–1258.
Fried, O., Tewari, A., Zollh
¨
ofer, M., Finkelstein, A.,
Shechtman, E., Goldman, D. B., Genova, K., Jin, Z.,
Theobalt, C., and Agrawala, M. (2019). Text-based
editing of talking-head video. ACM Transactions on
Graphics (TOG), 38(4):1–14.
G
´
omez, L., Biten, A. F., Tito, R., Mafla, A., Rusi
˜
nol, M.,
Valveny, E., and Karatzas, D. (2020). Multimodal grid
features and cell pointers for scene text visual question
answering. arXiv preprint arXiv:2006.00923.
Gulcehre, C., Ahn, S., Nallapati, R., Zhou, B., and Bengio,
Y. (2016). Pointing the unknown words. In Proceed-
ings of the 54th Annual Meeting of the Association for
Computational Linguistics (Volume 1: Long Papers),
pages 140–149.
He, K., Zhang, X., Ren, S., and Sun, J. (2016). Deep resid-
ual learning for image recognition. In Proceedings of
the IEEE conference on computer vision and pattern
recognition, pages 770–778.
Laiola Guimaraes, R., Cesar, P., Bulterman, D. C., Zsom-
bori, V., and Kegel, I. (2011). Creating personalized
memories from social events: community-based sup-
port for multi-camera recordings of school concerts.
In Proceedings of the 19th ACM international confer-
ence on Multimedia, pages 303–312.
Leake, M., Davis, A., Truong, A., and Agrawala, M.
(2017). Computational video editing for dialogue-
driven scenes. ACM Trans. Graph., 36(4):130–1.
Liao, Z., Yu, Y., Gong, B., and Cheng, L. (2015). Au-
deosynth: music-driven video montage. ACM Trans-
actions on Graphics (TOG), 34(4):1–10.
See, A., Liu, P. J., and Manning, C. D. (2017). Get to
the point: Summarization with pointer-generator net-
works. In Proceedings of the 55th Annual Meeting
of the Association for Computational Linguistics (Vol-
ume 1: Long Papers), pages 1073–1083.
Shrestha, P., de With, P. H., Weda, H., Barbieri, M., and
Aarts, E. H. (2010). Automatic mashup generation
from multiple-camera concert recordings. In Pro-
ceedings of the 18th ACM international conference on
Multimedia, pages 541–550.
Singh, A., Natarajan, V., Shah, M., Jiang, Y., Chen, X.,
Batra, D., Parikh, D., and Rohrbach, M. (2019). To-
wards vqa models that can read. In Proceedings of the
IEEE/CVF Conference on Computer Vision and Pat-
tern Recognition, pages 8317–8326.
Truong, A., Berthouzoz, F., Li, W., and Agrawala, M.
(2016). Quickcut: An interactive tool for editing nar-
rated video. In Proceedings of the 29th Annual Sym-
posium on User Interface Software and Technology,
pages 497–507.
Vinyals, O., Fortunato, M., and Jaitly, N. (2015). Pointer
networks. arXiv preprint arXiv:1506.03134.
Wang, M., Yang, G.-W., Hu, S.-M., Yau, S.-T., and
Shamir, A. (2019). Write-a-video: computational
video montage from themed text. ACM Trans. Graph.,
38(6):177–1.
Wu, H.-Y. and Christie, M. (2015). Stylistic patterns for
generating cinematographic sequences. In 4th Work-
shop on Intelligent Cinematography and Editing Co-
Located w/Eurographics 2015.
Wu, H.-Y. and Jhala, A. (2018). A joint attention model for
automated editing. In INT/WICED@ AIIDE.
Zhou, B., Lapedriza, A., Khosla, A., Oliva, A., and Tor-
ralba, A. (2017). Places: A 10 million image database
for scene recognition. IEEE Transactions on Pattern
Analysis and Machine Intelligence.
VISAPP 2022 - 17th International Conference on Computer Vision Theory and Applications
948