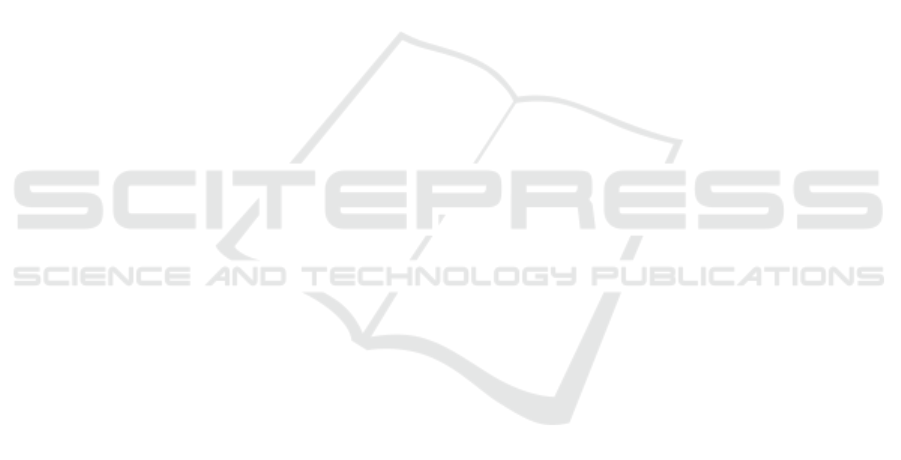
fields. In Proceedings of the IEEE Conference
on Computer Vision and Pattern Recognition, pages
8827–8836.
Qi, C. R., Su, H., Mo, K., and Guibas, L. J. (2017a). Point-
net: Deep learning on point sets for 3d classification
and segmentation. In Proceedings of the IEEE con-
ference on computer vision and pattern recognition,
pages 652–660.
Qi, C. R., Yi, L., Su, H., and Guibas, L. J. (2017b). Point-
net++: Deep hierarchical feature learning on point sets
in a metric space. arXiv preprint arXiv:1706.02413.
Russell, C., Kohli, P., Torr, P. H., et al. (2009). Associa-
tive hierarchical crfs for object class image segmen-
tation. In Computer Vision, 2009 IEEE 12th Interna-
tional Conference on, pages 739–746. IEEE.
Song, S., Yu, F., Zeng, A., Chang, A. X., Savva, M., and
Funkhouser, T. (2017). Semantic scene completion
from a single depth image. Proceedings of 30th IEEE
Conference on Computer Vision and Pattern Recogni-
tion.
Straub, J., Whelan, T., Ma, L., Chen, Y., Wijmans, E.,
Green, S., Engel, J. J., Mur-Artal, R., Ren, C., Verma,
S., Clarkson, A., Yan, M., Budge, B., Yan, Y., Pan,
X., Yon, J., Zou, Y., Leon, K., Carter, N., Briales, J.,
Gillingham, T., Mueggler, E., Pesqueira, L., Savva,
M., Batra, D., Strasdat, H. M., Nardi, R. D., Goesele,
M., Lovegrove, S., and Newcombe, R. (2019). The
Replica dataset: A digital replica of indoor spaces.
arXiv preprint arXiv:1906.05797.
Sun, C.-Y., Zou, Q.-F., Tong, X., and Liu, Y. (2019). Learn-
ing adaptive hierarchical cuboid abstractions of 3d
shape collections. ACM Transactions on Graphics
(TOG), 38(6):1–13.
Uy, M. A., Pham, Q.-H., Hua, B.-S., Nguyen, T., and
Yeung, S.-K. (2019). Revisiting point cloud clas-
sification: A new benchmark dataset and classifica-
tion model on real-world data. In Proceedings of the
IEEE/CVF International Conference on Computer Vi-
sion, pages 1588–1597.
Wang, P.-S., Liu, Y., Guo, Y.-X., Sun, C.-Y., and Tong,
X. (2017). O-cnn: Octree-based convolutional neu-
ral networks for 3d shape analysis. ACM Transactions
on Graphics (TOG), 36(4):1–11.
Wang, W., Yu, R., Huang, Q., and Neumann, U. (2018).
Sgpn: Similarity group proposal network for 3d point
cloud instance segmentation. In Proceedings of the
IEEE Conference on Computer Vision and Pattern
Recognition, pages 2569–2578.
Wang, X., Liu, S., Shen, X., Shen, C., and Jia, J. (2019a).
Associatively segmenting instances and semantics in
point clouds. In Proceedings of the IEEE Conference
on Computer Vision and Pattern Recognition, pages
4096–4105.
Wang, Y., Sun, Y., Liu, Z., Sarma, S. E., Bronstein, M. M.,
and Solomon, J. M. (2019b). Dynamic graph cnn
for learning on point clouds. ACM Transactions on
Graphics (TOG), 38(5):1–12.
Wu, R., Zhuang, Y., Xu, K., Zhang, H., and Chen, B.
(2019a). Pq-net: A generative part seq2seq network
for 3d shapes. arXiv preprint arXiv:1911.10949.
Wu, Z., Wang, X., Lin, D., Lischinski, D., Cohen-Or, D.,
and Huang, H. (2019b). Sagnet: Structure-aware gen-
erative network for 3d-shape modeling. ACM Trans-
actions on Graphics (TOG), 38(4):1–14.
Xu, D., Zhu, Y., Choy, C. B., and Fei-Fei, L. (2017). Scene
graph generation by iterative message passing. In
The IEEE Conference on Computer Vision and Pat-
tern Recognition (CVPR).
Yang, B., Wang, J., Clark, R., Hu, Q., Wang, S., Markham,
A., and Trigoni, N. (2019). Learning object bounding
boxes for 3d instance segmentation on point clouds. In
Advances in Neural Information Processing Systems,
pages 6737–6746.
Yi, L., Guibas, L., Hertzmann, A., Kim, V. G., Su, H., and
Yumer, E. (2017). Learning hierarchical shape seg-
mentation and labeling from online repositories. arXiv
preprint arXiv:1705.01661.
Yi, L., Kim, V. G., Ceylan, D., Shen, I.-C., Yan, M., Su, H.,
Lu, C., Huang, Q., Sheffer, A., and Guibas, L. (2016).
A scalable active framework for region annotation in
3d shape collections. ACM Transactions on Graphics
(TOG), 35(6):1–12.
Yi, L., Zhao, W., Wang, H., Sung, M., and Guibas, L. J.
(2019). Gspn: Generative shape proposal network for
3d instance segmentation in point cloud. In Proceed-
ings of the IEEE Conference on Computer Vision and
Pattern Recognition, pages 3947–3956.
Yildirim, I., Kulkarni, T. D., Freiwald, W. A., and Tenen-
baum, J. B. (2015). Efficient and robust analysis-by-
synthesis in vision: A computational framework, be-
havioral tests, and modeling neuronal representations.
In Annual conference of the cognitive science society,
volume 1.
Yuille, A. and Kersten, D. (2006). Vision as bayesian in-
ference: analysis by synthesis? Trends in cognitive
sciences, 10(7):301–308.
Zacharov, I., Arslanov, R., Gunin, M., Stefonishin,
D., Bykov, A., Pavlov, S., Panarin, O., Mal-
iutin, A., Rykovanov, S., and Fedorov, M. (2019).
“zhores”—petaflops supercomputer for data-driven
modeling, machine learning and artificial intelligence
installed in skolkovo institute of science and technol-
ogy. Open Engineering, 9(1):512–520.
Zhang, B. and Wonka, P. (2019). Point cloud instance
segmentation using probabilistic embeddings. arXiv
preprint arXiv:1912.00145.
Zhang, Z. (2012). Microsoft kinect sensor and its effect.
IEEE multimedia, 19(2):4–10.
Zhu, C., Xu, K., Chaudhuri, S., Yi, L., Guibas, L., and
Zhang, H. (2019). Cosegnet: Deep co-segmentation
of 3d shapes with group consistency loss. arXiv
preprint arXiv:1903.10297.
Zhu, S.-C. and Mumford, D. (2006). A stochastic gram-
mar of images. Foundations and Trends® in Com-
puter Graphics and Vision, 2(4):259–362.
VISAPP 2022 - 17th International Conference on Computer Vision Theory and Applications
722