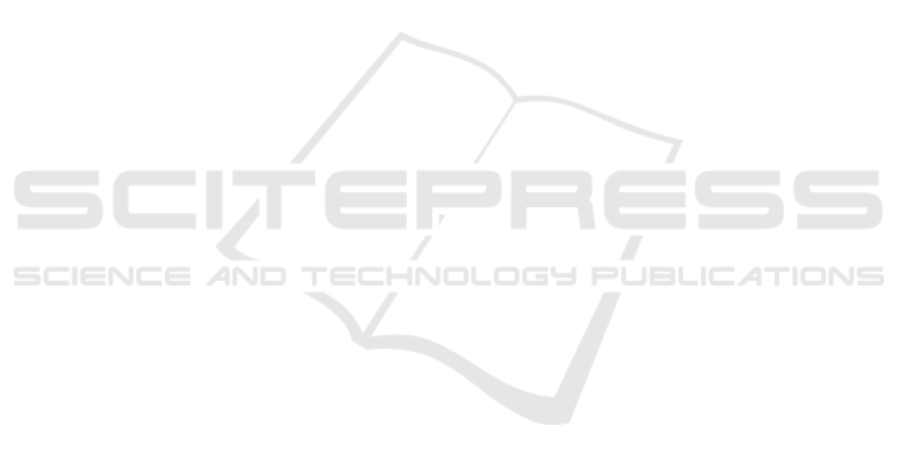
International Symposium on Neural Networks, pages
388–401. Springer.
Chen, L.-C., Papandreou, G., Kokkinos, I., Murphy, K., and
Yuille, A. L. (2017). Deeplab: Semantic image seg-
mentation with deep convolutional nets, atrous convo-
lution, and fully connected crfs. IEEE transactions on
pattern analysis and machine intelligence, 40(4):834–
848.
Chen, L.-C., Zhu, Y., Papandreou, G., Schroff, F., and
Adam, H. (2018). Encoder-decoder with atrous sepa-
rable convolution for semantic image segmentation. In
Proceedings of the European conference on computer
vision (ECCV), pages 801–818.
Chen, W., Jiang, Z., Wang, Z., Cui, K., and Qian,
X. (2019). Collaborative global-local networks for
memory-efficient segmentation of ultra-high resolu-
tion images. In Proceedings of the IEEE/CVF Con-
ference on Computer Vision and Pattern Recognition,
pages 8924–8933.
Cheng, H., Gu, C., and Wu, K. (2020). Weakly-supervised
semantic segmentation via self-training. In Journal
of Physics: Conference Series, volume 1487, page
012001. IOP Publishing.
Csurka, G., Larlus, D., Perronnin, F., and Meylan, F. (2013).
What is a good evaluation measure for semantic seg-
mentation?. In BMVC, volume 27, pages 10–5244.
Delany, M. and Bazley, E. (1970). Acoustical properties
of fibrous absorbent materials. Applied Acoustics,
3(2):105–116.
Deng, J., Dong, W., Socher, R., Li, L.-J., Li, K., and Fei-
Fei, L. (2009). Imagenet: A large-scale hierarchical
image database. In 2009 IEEE conference on com-
puter vision and pattern recognition, pages 248–255.
Ieee.
Everingham, M., Eslami, S. A., Van Gool, L., Williams,
C. K., Winn, J., and Zisserman, A. (2015). The pascal
visual object classes challenge: A retrospective. Inter-
national journal of computer vision, 111(1):98–136.
Farhadi, A., Endres, I., Hoiem, D., and Forsyth, D. (2009).
Describing objects by their attributes. In 2009 IEEE
Conference on Computer Vision and Pattern Recogni-
tion, pages 1778–1785. IEEE.
Goodfellow, I., Bengio, Y., and Courville, A. (2016). Deep
learning. MIT press.
He, K., Zhang, X., Ren, S., and Sun, J. (2016). Deep resid-
ual learning for image recognition. In Proceedings of
the IEEE conference on computer vision and pattern
recognition, pages 770–778.
Hu, D., Bo, L., and Ren, X. (2011). Toward robust material
recognition for everyday objects. In BMVC, volume 2,
page 6. Citeseer.
Iodice, S. and Mikolajczyk, K. (2020). Text attribute ag-
gregation and visual feature decomposition for person
search. In BMVC.
Kang, J., Fernandez-Beltran, R., Sun, X., Ni, J., and Plaza,
A. (2021). Deep learning-based building footprint ex-
traction with missing annotations. IEEE Geoscience
and Remote Sensing Letters.
Kim, H., Remaggi, L., Jackson, P. J., and Hilton, A. (2019).
Immersive spatial audio reproduction for vr/ar using
room acoustic modelling from 360 images. In 2019
IEEE Conference on Virtual Reality and 3D User In-
terfaces (VR), pages 120–126. IEEE.
Kr
¨
ahenb
¨
uhl, P. and Koltun, V. (2013). Parameter learn-
ing and convergent inference for dense random fields.
In International Conference on Machine Learning,
pages 513–521. PMLR.
Le, T. H. N., Luu, K., and Savvides, M. (2015). Fast and ro-
bust self-training beard/moustache detection and seg-
mentation. In 2015 international conference on bio-
metrics (ICB), pages 507–512. IEEE.
Lin, T.-Y., Doll
´
ar, P., Girshick, R., He, K., Hariharan, B.,
and Belongie, S. (2017a). Feature pyramid networks
for object detection. In Proceedings of the IEEE con-
ference on computer vision and pattern recognition,
pages 2117–2125.
Lin, T.-Y., Goyal, P., Girshick, R., He, K., and Doll
´
ar, P.
(2017b). Focal loss for dense object detection. In
Proceedings of the IEEE international conference on
computer vision, pages 2980–2988.
Long, J., Shelhamer, E., and Darrell, T. (2015). Fully con-
volutional networks for semantic segmentation. In
Proceedings of the IEEE conference on computer vi-
sion and pattern recognition, pages 3431–3440.
McDonagh, A., Lemley, J., Cassidy, R., and Corcoran, P.
(2018). Synthesizing game audio using deep neural
networks. In 2018 IEEE Games, Entertainment, Me-
dia Conference (GEM), pages 1–9. IEEE.
Schwartz, G. (2018). Visual Material Recognition. Drexel
University.
Schwartz, G. and Nishino, K. (2016). Material recognition
from local appearance in global context. In Biol. and
Artificial Vision (Workshop held in conjunction with
ECCV 2016).
Schwartz, G. and Nishino, K. (2020). Recognizing material
properties from images. IEEE Transactions on Pattern
Analysis and Machine Intelligence, 42(8):1981–1995.
Sharan, L., Liu, C., Rosenholtz, R., and Adelson, E. H.
(2013). Recognizing materials using perceptually in-
spired features. International journal of computer vi-
sion, 103(3):348–371.
Shrivatsav, N., Nair, L., and Chernova, S. (2019). Tool sub-
stitution with shape and material reasoning using dual
neural networks. arXiv preprint arXiv:1911.04521.
Silberman, N., Hoiem, D., Kohli, P., and Fergus, R. (2012).
Indoor segmentation and support inference from rgbd
images. In European conference on computer vision,
pages 746–760. Springer.
Souly, N., Spampinato, C., and Shah, M. (2017). Semi su-
pervised semantic segmentation using generative ad-
versarial network. In Proceedings of the IEEE inter-
national conference on computer vision, pages 5688–
5696.
Su, H., Jampani, V., Sun, D., Gallo, O., Learned-Miller,
E., and Kautz, J. (2019). Pixel-adaptive convolutional
neural networks. In Proceedings of the IEEE/CVF
Conference on Computer Vision and Pattern Recog-
nition, pages 11166–11175.
Tang, Z., Bryan, N. J., Li, D., Langlois, T. R., and Manocha,
D. (2020). Scene-aware audio rendering via deep
VISAPP 2022 - 17th International Conference on Computer Vision Theory and Applications
198