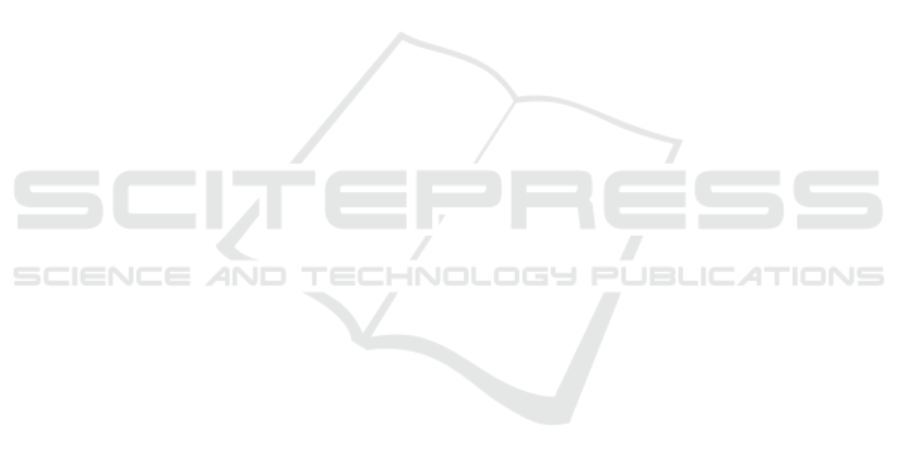
ground truth annotations for all of them.
We performed an initial benchmark of the few ex-
isting methods showing that there is significant room
for improvement. In particular, the accuracy of the
evaluated methods is insufficient for them to be used
in real-world industrial applications. We are convinced
that suitable datasets are a key factor in the develop-
ment of new techniques and expect our dataset to spark
the design of better methods in the future.
REFERENCES
Armeni, I., Sener, O., Zamir, A. R., Jiang, H., Brilakis, I.,
Fischer, M., and Savarese, S. (2016). 3D Semantic
Parsing of Large-Scale Indoor Spaces. In IEEE Con-
ference on Computer Vision and Pattern Recognition
(CVPR), pages 1534–1543.
Baid, U. et al. (2021). The RSNA-ASNR-MICCAI
BraTS 2021 Benchmark on Brain Tumor Segmen-
tation and Radiogenomic Classification. Preprint:
arXiv:2107.02314.
Bakas, S., Akbari, H., Sotiras, A., Bilello, M., Rozycki,
M., Kirby, J. S., et al. (2017). Advancing the cancer
genome atlas glioma MRI collections with expert seg-
mentation labels and radiomic features. Scientific Data,
4(1).
Bengs, M., Behrendt, F., Krüger, J., Opfer, R., and Schlaefer,
A. (2021). Three-dimensional deep learning with spa-
tial erasing for unsupervised anomaly segmentation in
brain MRI. International Journal of Computer Assisted
Radiology and Surgery, 16.
Bergmann, P., Batzner, K., Fauser, M., Sattlegger, D., and
Steger, C. (2021). The MVTec Anomaly Detection
Dataset: A Comprehensive Real-World Dataset for Un-
supervised Anomaly Detection. International Journal
of Computer Vision, 129(4):1038–1059.
Bergmann, P., Fauser, M., Sattlegger, D., and Steger, C.
(2019a). MVTec AD — A Comprehensive Real-World
Dataset for Unsupervised Anomaly Detection. In IEEE
Conference on Computer Vision and Pattern Recogni-
tion (CVPR), pages 9584–9592.
Bergmann, P., Fauser, M., Sattlegger, D., and Steger,
C. (2020). Uninformed Students: Student-Teacher
Anomaly Detection With Discriminative Latent Em-
beddings. In IEEE/CVF Conference on Computer Vi-
sion and Pattern Recognition (CVPR), pages 4182–
4191.
Bergmann, P., Löwe, S., Fauser, M., Sattlegger, D., and
Steger., C. (2019b). Improving Unsupervised Defect
Segmentation by Applying Structural Similarity to Au-
toencoders. In 14th International Joint Conference
on Computer Vision, Imaging and Computer Graphics
Theory and Applications - Volume 5: VISAPP, pages
372–380. INSTICC, SciTePress.
Blum, H., Sarlin, P.-E., Nieto, J., Siegwart, R., and Ca-
dena, C. (2019). Fishyscapes: A Benchmark for Safe
Semantic Segmentation in Autonomous Driving. In
IEEE/CVF International Conference on Computer Vi-
sion Workshops (ICCVW), pages 2403–2412.
Carrera, D., Manganini, F., Boracchi, G., and Lanzarone, E.
(2017). Defect Detection in SEM Images of Nanofi-
brous Materials. IEEE Transactions on Industrial In-
formatics, 13(2):551–561.
Chang, A. X., Funkhouser, T., Guibas, L., Hanrahan, P.,
Huang, Q., Li, Z., Savarese, S., Savva, M., Song, S.,
Su, H., Xiao, J., Yi, L., and Yu, F. (2015). ShapeNet:
An Information-Rich 3D Model Repository. Preprint:
arXiv:1512.03012.
Cohen, N. and Hoshen, Y. (2021). Sub-Image Anomaly De-
tection with Deep Pyramid Correspondences. Preprint:
arXiv:2005.02357.
Dai, A., Chang, A. X., Savva, M., Halber, M., Funkhouser,
T., and Nießner, M. (2017). ScanNet: Richly-
Annotated 3D Reconstructions of Indoor Scenes. In
IEEE Conference on Computer Vision and Pattern
Recognition (CVPR), pages 2432–2443.
Drost, B., Ulrich, M., Bergmann, P., Härtinger, P., and Steger,
C. (2017). Introducing MVTec ITODD — A Dataset
for 3D Object Recognition in Industry. In IEEE Inter-
national Conference on Computer Vision Workshops
(ICCVW), pages 2200–2208.
Goodfellow, I., Pouget-Abadie, J., Mirza, M., Xu, B., Warde-
Farley, D., Ozair, S., Courville, A., and Bengio, Y.
(2014). Generative Adversarial Nets. In Advances in
Neural Information Processing Systems, pages 2672–
2680.
Hoda
ˇ
n, T., Sundermeyer, M., Drost, B., Labbé, Y., Brach-
mann, E., Michel, F., Rother, C., and Matas, J. (2020).
BOP Challenge 2020 on 6D Object Localization. Eu-
ropean Conference on Computer Vision Workshops
(ECCVW).
Huang, Y., Qiu, C., Guo, Y., Wang, X., and Yuan, K. (2018).
Surface Defect Saliency of Magnetic Tile. In 2018
IEEE 14th International Conference on Automation
Science and Engineering (CASE), pages 612–617.
Kingma, D. P. and Ba, J. (2015). Adam: A Method for
Stochastic Optimization. In 3rd International Confer-
ence on Learning Representations (ICLR).
Krizhevsky, A., Sutskever, I., and Hinton, G. E. (2012). Ima-
geNet Classification with Deep Convolutional Neural
Networks. In 25th International Conference on Neu-
ral Information Processing Systems - Volume 1, pages
1097–1105.
Li, W.-X., Mahadevan, V., and Vasconcelos, N. (2013).
Anomaly Detection and Localization in Crowded
Scenes. IEEE Transactions on Pattern Analysis and
Machine Intelligence (TPAMI), 36(1):18–32.
Liew, S.-L., Anglin, J. M., Banks, N. W., et al. (2018). A
large, open source dataset of stroke anatomical brain
images and manual lesion segmentations. Scientific
data, 5:180011.
Lu, C., Shi, J., and Jia, J. (2013). Abnormal Event Detec-
tion at 150 FPS in MATLAB. In IEEE International
Conference on Computer Vision, pages 2720–2727.
Masci, J., Meier, U., Cire¸san, D., and Schmidhuber, J. (2011).
Stacked Convolutional Auto-Encoders for Hierarchi-
cal Feature Extraction. In Artificial Neural Networks
VISAPP 2022 - 17th International Conference on Computer Vision Theory and Applications
210