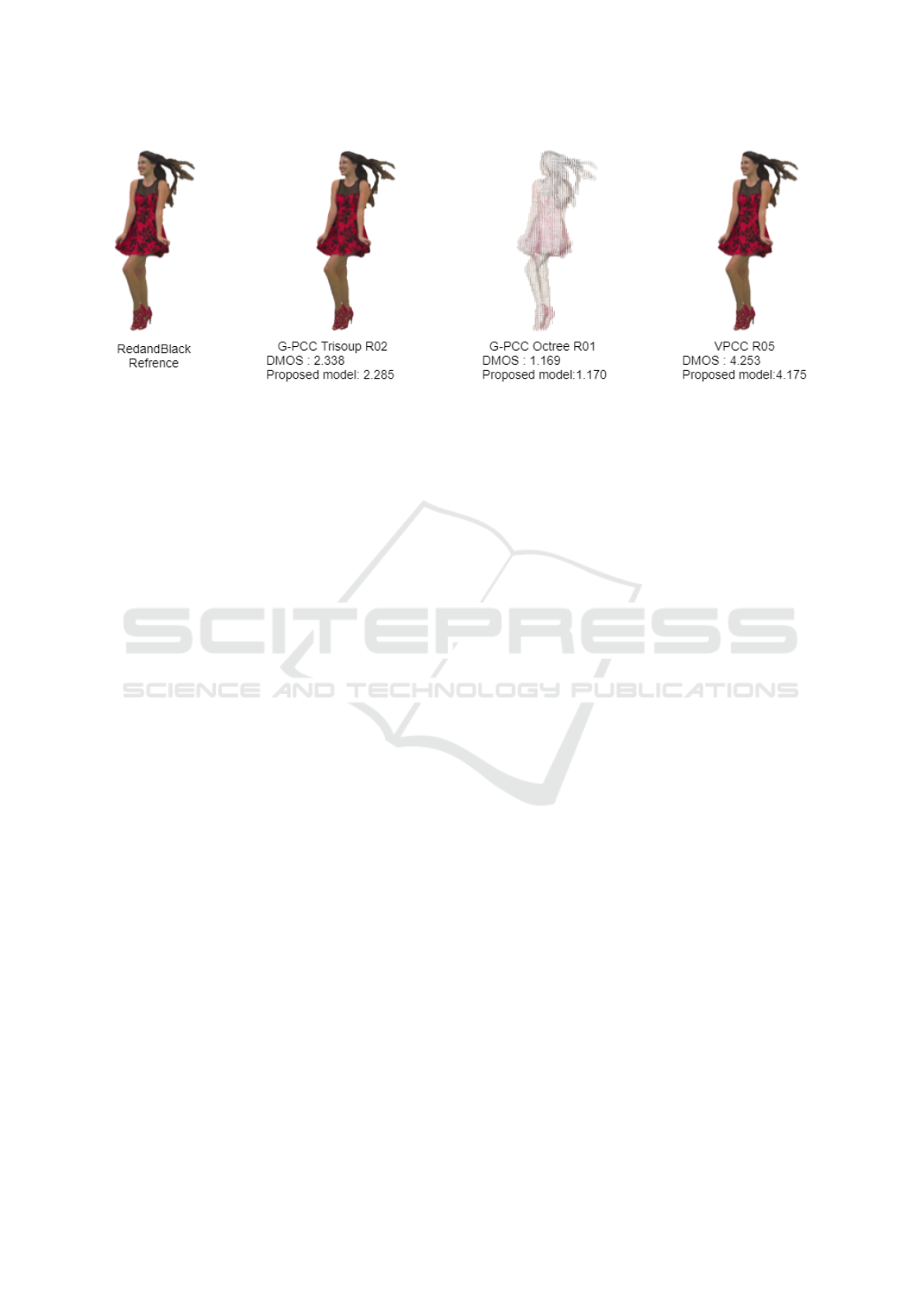
Figure 5: Perceptual quality score comparison between the proposed model and the subjective quality score (MOS) on
RedandBlack object from ICIP2020 dataset.
REFERENCES
Abouelaziz, I., Chetouani, A., Hassouni, M. E., Latecki,
L. J., and Cherifi, H. (2018). Convolutional neural
network for blind mesh visual quality assessment us-
ing 3d visual saliency. In 2018 25th IEEE Interna-
tional Conference on Image Processing (ICIP), pages
3533–3537.
Abouelaziz, I., Chetouani, A., Hassouni, M. E., Latecki,
L. J., and Cherifi, H. (2020a). 3d visual saliency and
convolutional neural network for blind mesh quality
assessment. Neural Comput. Appl., 32(21):16589–
16603.
Abouelaziz, I., Chetouani, A., Hassouni, M. E., Latecki,
L. J., and Cherifi, H. (2020b). No-reference mesh
visual quality assessment via ensemble of convolu-
tional neural networks and compact multi-linear pool-
ing. Pattern Recognit., 100:107174.
Alexiou, E. and Ebrahimi, T. (2018). Point cloud qual-
ity assessment metric based on angular similarity. In
2018 IEEE International Conference on Multimedia
and Expo (ICME), pages 1–6.
Alexiou, E. and Ebrahimi, T. (2020). Towards a point
cloud structural similarity metric. In 2020 IEEE Inter-
national Conference on Multimedia Expo Workshops
(ICMEW), pages 1–6.
Bourbia, S., Karine, A., Chetouani, A., and El Hassouni,
M. (2021). A Multi-task convolutional neural net-
work for blind stereoscopic image quality assessment
using naturalness analysis. In The 28th IEEE Interna-
tional Conference on Image Processing (IEEE - ICIP),
Anchorage-Alaska, United States.
Chen, T., Long, C., Su, H., Chen, L., Chi, J., Pan, Z.,
Yang, H., and Liu, Y. (2021). Layered projection-
based quality assessment of 3d point clouds. IEEE
Access, 9:88108–88120.
Chetouani, A. (2014). Full reference image quality metric
for stereo images based on cyclopean image compu-
tation and neural fusion. In 2014 IEEE Visual Com-
munications and Image Processing Conference, pages
109–112.
Chetouani, A. (2018). Convolutional neural network and
saliency selection for blind image quality assessment.
In 2018 25th IEEE International Conference on Image
Processing (ICIP), pages 2835–2839.
Chetouani, A. (2019). Blind utility and quality assessment
using a convolutional neural network and a patch se-
lection. In 2019 IEEE International Conference on
Image Processing (ICIP), pages 459–463.
Deng, J., Dong, W., Socher, R., Li, L.-J., Li, K., and Fei-
Fei, L. (2009). Imagenet: A large-scale hierarchical
image database. In 2009 IEEE Conference on Com-
puter Vision and Pattern Recognition, pages 248–255.
Diniz, R., Farias, M. Q., and Garcia-Freitas, P. (2021).
Color and geometry texture descriptors for point-
cloud quality assessment. IEEE Signal Processing
Letters.
Fourati, E., Elloumi, W., and Chetouani, A. (2020). Anti-
spoofing in face recognition-based biometric authenti-
cation using image quality assessment. Multim. Tools
Appl., 79(1-2):865–889.
Gao, L., He, C., Luo, W., Cui, Y., Fan, Q., Peng, Q., Zhao,
G., Hao, X., Xia, Y., and Zhang, P. (2017). Blind im-
age quality assessment model based on deep convolu-
tional neural network. In 2017 International Confer-
ence on Computer Technology, Electronics and Com-
munication (ICCTEC), pages 336–339.
He, K., Zhang, X., Ren, S., and Sun, J. (2015). Deep
residual learning for image recognition. CoRR,
abs/1512.03385.
Javaheri, A., Brites, C., Pereira, F., and Ascenso, J. (2020a).
A generalized hausdorff distance based quality metric
for point cloud geometry. In 2020 Twelfth Interna-
tional Conference on Quality of Multimedia Experi-
ence (QoMEX), pages 1–6. IEEE.
Javaheri, A., Brites, C., Pereira, F., and Ascenso, J. (2020b).
Mahalanobis based point to distribution metric for
point cloud geometry quality evaluation. IEEE Sig-
nal Processing Letters, 27:1350–1354.
Javaheri, A., Brites, C., Pereira, F., and Ascenso, J. (2021).
A point-to-distribution joint geometry and color met-
ric for point cloud quality assessment. arXiv preprint
arXiv:2108.00054.
VISAPP 2022 - 17th International Conference on Computer Vision Theory and Applications
524