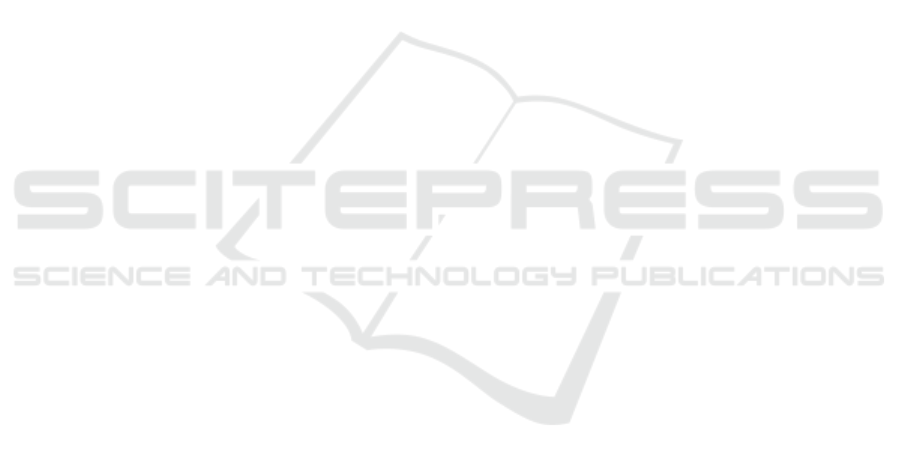
Khorshidi, S., Carter, J., Mohler, G., and Tita, G. (2021).
Explaining crime diversity with google street view.
Journal of Quantitative Criminology, 37(2):361–391.
Li, X., Zhang, C., Li, W., Ricard, R., Meng, Q., and Zhang,
W. (2015). Assessing street-level urban greenery us-
ing google street view and a modified green view in-
dex. Urban Forestry & Urban Greening, 14(3):675–
685.
Lin, T.-Y., Maire, M., Belongie, S., Hays, J., Perona, P.,
Ramanan, D., Doll
´
ar, P., and Zitnick, C. L. (2014).
Microsoft coco: Common objects in context. In Euro-
pean conference on computer vision, pages 740–755.
Springer.
Lynch, K. (1984). Reconsidering the image of the city. In
Cities of the Mind, pages 151–161. Springer.
Megler, V., Banis, D., and Chang, H. (2014). Spatial anal-
ysis of graffiti in san francisco. Applied Geography,
54:63–73.
Moreno-Vera, F., Lavi, B., and Poco, J. (2021a). Quantify-
ing urban safety perception on street view images. In
2021 IEEE/WIC/ACM International Joint Conference
on Web Intelligence and Intelligent Agent Technology
(WI-IAT). IEEE.
Moreno-Vera, F., Lavi, B., and Poco, J. (2021b). Urban per-
ception: Can we understand why a street is safe? In
Mexican International Conference on Artificial Intel-
ligence, pages 277–288. Springer.
Naik, N., Philipoom, J., Raskar, R., and Hidalgo, C. (2014).
StreetScore: predicting the perceived safety of one
million streetscapes. 2014 IEEE Conference on Com-
puter Vision and Pattern Recognition Workshops.
Naik, N., Raskar, R., and Hidalgo, C. A. (2016). Cities are
physical too: Using computer vision to measure the
quality and impact of urban appearance. American
Economic Review, 106(5):128–32.
Ordonez, V. and Berg, T. L. (2014). Learning high-level
judgments of urban perception. European Conference
on Computer Vision (ECCV).
Paszke, A., Gross, S., Massa, F., Lerer, A., Bradbury, J.,
Chanan, G., Killeen, T., Lin, Z., Gimelshein, N.,
Antiga, L., et al. (2019). Pytorch: An imperative style,
high-performance deep learning library. Advances
in neural information processing systems, 32:8026–
8037.
Redmon, J., Divvala, S., Girshick, R., and Farhadi, A.
(2016). You only look once: Unified, real-time object
detection. In Proceedings of the IEEE conference on
computer vision and pattern recognition, pages 779–
788.
Ren, S., He, K., Girshick, R., and Sun, J. (2016). Faster
r-cnn: towards real-time object detection with region
proposal networks. IEEE transactions on pattern
analysis and machine intelligence, 39(6):1137–1149.
Sampson, R. J. and Raudenbush, S. W. (2001). Disorder in
urban neighborhoods: Does it lead to crime. US De-
partment of Justice, Office of Justice Programs, Na-
tional Institute of . . . .
Schroeder, H. W. and Anderson, L. M. (1984). Perception
of personal safety in urban recreation sites. Journal of
leisure research, 16(2):178–194.
Simonyan, K. and Zisserman, A. (2014). Very deep con-
volutional networks for large-scale image recognition.
arXiv preprint arXiv:1409.1556.
Tokuda, E. K., Cesar, R. M., and Silva, C. T. (2019). Quan-
tifying the presence of graffiti in urban environments.
In 2019 IEEE International Conference on Big Data
and Smart Computing (BigComp), pages 1–4. IEEE.
Torii, A., Havlena, M., and Pajdla, T. (2009). From google
street view to 3d city models. In IEEE 12th Inter-
national Conference on Computer Vision workshops,
pages 2188–2195. IEEE.
Yoshimura, Y., He, S., Hack, G., Nagakura, T., and Ratti, C.
(2020). Quantifying memories: Mapping urban per-
ception. Mobile Networks and Applications, 25:1275–
1286.
Zhang, F., Zhou, B., Liu, L., Liu, Y., Fung, H. H., Lin, H.,
and Ratti, C. (2018). Measuring human perceptions
of a large-scale urban region using machine learning.
Landscape and Urban Planning, 180:148–160.
Zhao, Z.-Q., Zheng, P., Xu, S.-t., and Wu, X. (2019). Ob-
ject detection with deep learning: A review. IEEE
transactions on neural networks and learning systems,
30(11):3212–3232.
17K-Graffiti: Spatial and Crime Data Assessments in São Paulo City
975