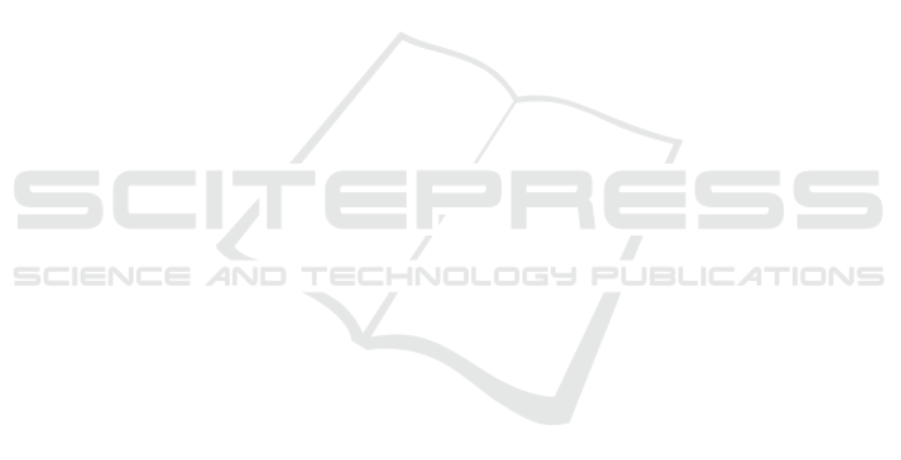
preventive measures and policy to guide and provide
safety to society.
Current tools such as web-based questionnaire
surveys or phone interviews to collect the data from
the community are time-consuming, labor-intensive,
and costly. Moreover, the long delays of data gather-
ing can make the time-critical decisions suffered. It
is important to develop an effective method to collect
data and extract the opinions of society. In this study,
we proposed to utilize social media to accomplish this
goal.
By October 2020, Twitter has more than 47 mil-
lion accounts from the US with 56 percent males and
44 percent females (updated on 10/10/2020) (Twitter,
2020). Real-time monitoring of public health based
on data from social media is promising. In addition,
thanks to the availability of APIs and services, col-
lecting data from social media platforms is straight-
forward. In this study, we analyzed the tweets posted
on Twitter to understand the opinions of social me-
dia users, and society in general, on the use of HCQ
for COVID-19 treatment. We conducted both descrip-
tive analysis and sentiment analysis to reveal the hid-
den reaction patterns and the shifting of their per-
ceptions on H4C over time. We linked the tweets
and Google keyword search frequencies to shed light
on the hidden information of the users’ opinions on
the topic in the space domain. We also evaluated
and compared the performance of the state-of-the-
art sentiment analysis tools including Google Cloud
Natural Language API (GCNL) and Valence Aware
Dictionary and Sentiment Reasoner Python library
(VADER) on the tweets as well.
There is some existing work studying online dis-
cussions on hydroxychloroquine for COVID-19 treat-
ment. The authors in (Hamamsy and Bonneau, 2020)
calculated the number of tweets mentioning this drug
per day from Feb 28 to May 22, 2020, on Twitter
to reveal the patterns. They also computed the av-
erage sentiment per day to understand the opinions of
users on the topic. They found that peaks of reac-
tions on HCQ posts appeared after the days’ Trump
promoted HCQ on social media. In another study
(Xue et al., 2020), the authors analyzed Twitter dis-
cussions and emotions using a machine learning ap-
proach. In this study, a tweet was classified into one
of the eight classes of emotions and one of the thir-
teen topics to understand the users’ opinions. Data
showed that “anticipation” was the most dominant
theme while “surprise” is the least across all 13 topics.
Furthermore, the authors in (Niburski and Niburski,
2020) studied the impact of Trump’s promotion of
HCQ for COVID-19 patients by analyzing social me-
dia content. It’s reported that the frequencies substan-
tially increased after Trump’s discussions about HCQ.
However, all of these studies limited their findings in a
very short period ((Niburski and Niburski, 2020) has
only 2 months) and that may not be sufficient to re-
veal the changing of the opinions associated with the
development of the pandemic.
Our work expands the existing frameworks by col-
lecting a more complete dataset spanning in much
longer time duration (10 months). In addition, we
conducted both descriptive analysis and sentiment
analysis of the tweets to understand the opinions of
users over time. To the best of our knowledge, we
are one of the first studies to link tweets, Google key-
word search frequencies, and data from the Centers
for Disease Control and Prevention (CDC) to reveal
the users’ reaction patterns on H4C. Finally, we con-
ducted a manual classification of 4,850 tweet senti-
ments to evaluate and compare the existing state-of-
the-art sentiment analysis tools including GCNL and
VADER. In summary, our contributions in this study
include:
1. More Complete Dataset: We collected 164,016
HCQ related tweets from February to December
of 2020 in our study. The collected data provides
a more complete picture of society’s perspectives
on the use of HCQ for COVID-19 treatment. This
is one of the most complete datasets on the topic
that has been collected so far.
2. Identifying Reactions Patterns in both Time
and Space Domains: We conducted both descrip-
tive and sentiment analysis in both time and space
domains to reveal the reaction patterns of both
online and geographically local communities on
H4C.
3. Linking Multiple Data Sources to Reveal Hid-
den Reaction Patterns: We also linked data from
Twitter, Google, and CDC to identify reaction pat-
terns and the relationship between “listening” (re-
actions on Twitter) and “doing” (search queries
on Google) and “did” (purchased drug, CDC re-
ports).
4. Conducting Manual Classifier: In this study, we
manually classified 4,850 tweets associated with
important events of the HCQ and COVID-19 de-
velopments to evaluate and compare the existing
sentiment analysis tools. To our best knowledge,
this is one of the largest US-based users datasets
of tweets regarding COVID-19 and HCQ. We plan
to share this dataset with the research community
upon completion of this project.
The remainder of the paper is as follows. In Section
II, we present our system architecture and data pro-
cessing workflow. In Section III, we describe our data
HEALTHINF 2022 - 15th International Conference on Health Informatics
632