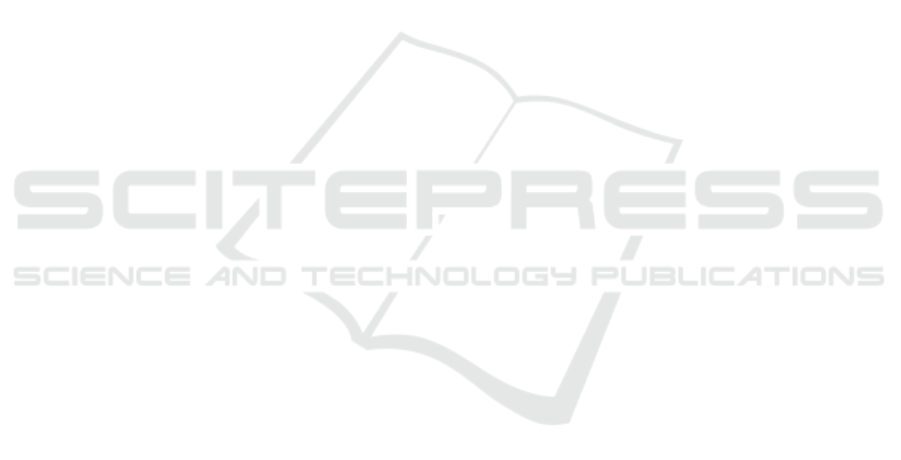
5 CONCLUSION
In this study, skeleton-based online sign language
recognition using monotonic attention was investi-
gated. A total of three monotonic attention techniques
were applied to continuous sign language word recog-
nition based on the STGCN-RNNA model. The ef-
fectiveness of the monotonic attention for online con-
tinuous sign language word recognition was demon-
strated through the results of the evaluation using the
JSL video dataset.
Seq2Seq-based online recognition has been well
researched within the speech recognition and nat-
ural language processing domains. Recently,
Transformer-based online recognition methods have
also been proposed (Tsunoo et al., 2019; Inaguma
et al., 2020; Miao et al., 2020; Li et al., 2021) in the
field. Future studies will include investigations on the
applicability of these methods to online sign language
recognition.
Furthermore, the authors have considered online
sign language translation as an interesting research
topic for future studies. The techniques for simulta-
neous translation in natural language processing (Gu
et al., 2017; Dalvi et al., 2018; Ma et al., 2019) can be
expected to contribute in this direction.
ACKNOWLEDGEMENTS
This research is supported by SoftBank Corp.
REFERENCES
Arivazhagan, N., Cherry, C., Macherey, W., Chiu, C.-C.,
Yavuz, S., Pang, R., Li, W., and Raffel, C. (2019).
Monotonic infinite lookback attention for simultane-
ous machine translation. In Proceedings of the 57th
Annual Meeting of the Association for Computational
Linguistics, pages 1313–1323.
Bahdanau, D., Cho, K., and Bengio, Y. (2015). Neural ma-
chine translation by jointly learning to align and trans-
late. In Proceedings of the Third International Con-
ference on Learning Representations, pages 1–15.
Camgoz, N. C., Hadfield, S., Koller, O., Ney, H., and Bow-
den, R. (2018). Neural sign language translation. In
Proceedings of the IEEE Conference on Computer Vi-
sion and Pattern Recognition, pages 7784–7793.
Camgoz, N. C., Koller, O., Hadfield, S., and Bowden, R.
(2020). Sign language transformers: Joint end-to-end
sign language recognition and translation. In Proceed-
ings of the IEEE Conference on Computer Vision and
Pattern Recognition, pages 10023–10033.
Cao, Z., Hidalgo, G., Simon, T., Wei, S.-E., and Sheikh,
Y. (2021). Openpose: Realtime multi-person 2d pose
estimation using part affinity fields. IEEE Transac-
tions on Pattern Analysis and Machine Intelligence,
43(1):172–186.
Chiu, C.-C. and Raffel, C. (2018). Monotonic chunkwise
attention. In Proceedings of the Sixth International
Conference on Learning Representations, pages 1–16.
Cho, K., van Merri
¨
enboer, B., Gulcehre, C., Bahdanau, D.,
Bougares, F., Schwenk, H., and Bengio, Y. (2014).
Learning phrase representations using RNN encoder–
decoder for statistical machine translation. In Pro-
ceedings of the Conference on Empirical Methods in
Natural Language Processing, pages 1724–1734.
Cooper, H., Pugeault, N., and Bowden, R. (2011). Reading
the signs: A video based sign dictionary. In Proceed-
ings of the IEEE International Conference on Com-
puter Vision Workshops, pages 914–919.
Cui, R., Liu, H., and Zhang, C. (2019). A deep neural
framework for continuous sign language recognition
by iterative training. IEEE Transactions on Multime-
dia, 21(7):1880–1891.
Dalvi, F., Durrani, N., Sajjad, H., and Vogel, S. (2018). In-
cremental decoding and training methods for simul-
taneous translation in neural machine translation. In
Proceedings of the Annual Conference of the North
American Chapter of the Association for Computa-
tional Linguistics: Human Language Technologies,
pages 493–499.
De Coster, M., Van Herreweghe, M., and Dambre, J. (2020).
Sign language recognition with transformer networks.
In Proceedings of the Twelveth Language Resources
and Evaluation Conference, pages 6018–6024.
Forster, J., Koller, O., Oberd
¨
orfer, C., Gweth, Y., and
Ney, H. (2013). Improving continuous sign language
recognition: Speech recognition techniques and sys-
tem design. In Proceedings of the Fourth Workshop on
Speech and Language Processing for Assistive Tech-
nologies, pages 41–46.
Gu, J., Neubig, G., Cho, K., and Li, V. O. (2017). Learning
to translate in real-time with neural machine transla-
tion. In Proceedings of the 15th Conference of the Eu-
ropean Chapter of the Association for Computational
Linguistics, pages 1053–1062.
Guo, D., Zhou, W., Li, A., Li, H., and Wang, M. (2020).
Hierarchical recurrent deep fusion using adaptive clip
summarization for sign language translation. IEEE
Transactions on Image Processing, 29:1575–1590.
Huang, J., Zhou, W., Zhang, Q., Li, H., and Li, W. (2018).
Video-based sign language recognition without tem-
poral segmentation. In Proceedings of the 32nd AAAI
Conference on Artificial Intelligence, pages 2257–
2264.
Inaguma, H., Mimura, M., and Kawahara, T. (2020). En-
hancing monotonic multihead attention for streaming
asr. In Proceedings of the 21st INTERSPEECH, pages
2137–2141.
Jiang, S., Wang, L., Bai, Y., Li, K., and Fu, Y. (2021). Skele-
ton aware multi-modal sign language recognition. In
Proceedings of the IEEE/CVF Conference on Com-
puter Vision and Pattern Recognition Workshops.
Skeleton-based Online Sign Language Recognition using Monotonic Attention
607