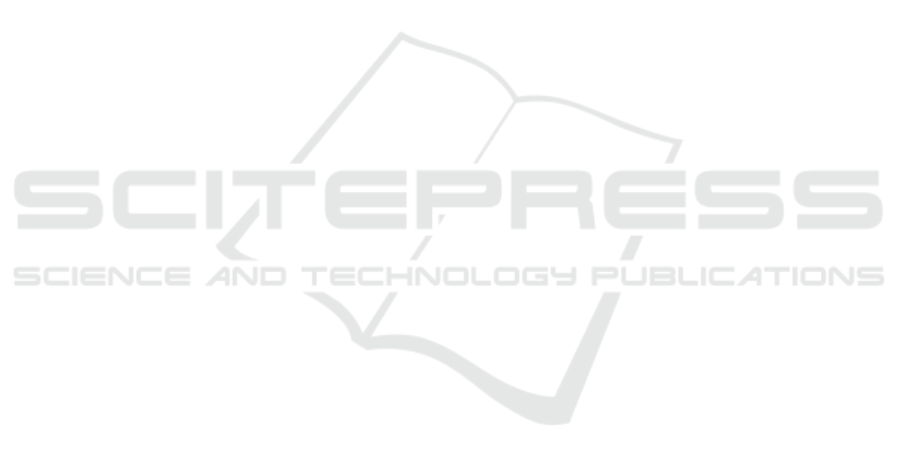
half of the AddNeuroMed consortium, S. L., Fr
¨
ohlich,
H., Hofmann-Apitius, M., the Japanese Alzheimer’s
Disease Neuroimaging Initiative, and the Alzheimer’s
Disease Neuroimaging Initiative (2020). Evaluating
the Alzheimer’s disease data landscape. Alzheimer’s
& Dementia: Translational Research & Clinical In-
terventions, 6(1):e12102.
Birkenbihl, C., Westwood, S., Shi, L., Nevado-Holgado,
A., Westman, E., Lovestone, S., Consortium, A., and
Hofmann-Apitius, M. (2021). ANMerge: A compre-
hensive and accessible Alzheimer’s disease patient-
level dataset. J Alzheimers Dis., 79:423–431.
Cheng, D. and Liu, M. (2017). CNNs based multi-modality
classification for AD diagnosis. In 2017 10th In-
ternational Congress on Image and Signal Process-
ing, BioMedical Engineering and Informatics (CISP-
BMEI), pages 1–5.
Gorgolewski, K., Auer, T., Calhoun, V., Craddock, R.,
Das, S., Duff, E., Flandin, G., Ghosh, S., Glatard, T.,
Halchenko, Y., Handwerker, D., Hanke, M., Keator,
D., Li, X., Michael, Z., Maumet, C., Nichols, B.,
Nichols, T., Pellman, J., Poline, J., Rokem, A., Schae-
fer, G., Sochat, V., Triplett, W., Turner, J., Varoquaux,
G., and Poldrack, R. (2016). The brain imaging data
structure, a format for organizing and describing out-
puts of neuroimaging experiments. Scientific data, 3.
Copyright: Copyright 2016 Elsevier B.V., All rights
reserved.
Gupta, Y., Lama, R. K., Kwon, G.-R., et al. (2019). Predic-
tion and classification of Alzheimer’s disease based
on combined features from apolipoprotein-e geno-
type, cerebrospinal fluid, MR, and FDG-PET imag-
ing biomarkers. Frontiers in Computational Neuro-
science, 13:72.
Joliot, M., Jobard, G., Naveau, M., Delcroix, N., Petit, L.,
Zago, L., Crivello, F., Mellet, E., Mazoyer, B., and
Tzourio-Mazoyer, N. (2015). AICHA: An atlas of in-
trinsic connectivity of homotopic areas. Journal of
Neuroscience Methods, 254:46–59.
Lawrence, E., Vegvari, C., Ower, A., Hadjichrysanthou, C.,
De Wolf, F., and RM, A. (2017). A systematic review
of longitudinal studies which measure Alzheimer’s
disease biomarkers. J Alzheimers Dis., 59(4):1359–
1379.
Lee, T. and Lee, H. (2020). Prediction of Alzheimer’s
disease using blood gene expression data. Sci Rep,
10(1):3485.
Li, F. and Liu, M. (2018). Alzheimer’s disease diagno-
sis based on multiple cluster dense convolutional net-
works. Computerized Medical Imaging and Graphics,
70:101–110.
Li, X., Wang, H., Long, J., et al. (2018). Systematic analysis
and biomarker study for Alzheimer’s disease. Sci Rep,
8:17394.
Lovestone, S., Francis, P., Kloszewska, I., Mecocci, P., Sim-
mons, A., Soininen, H., Spenger, C., Tsolaki, M., Vel-
las, B., Wahlund, L., Ward, M., and Consortium, A.
(2009). AddNeuroMed–the European collaboration
for the discovery of novel biomarkers for Alzheimer’s
disease. Ann N Y Acad Sci, pages 36–46.
Maddalena, L., Granata, I., Manipur, I., Manzo, M., and
Guarracino, M. (2020). Glioma grade classification
via omics imaging. In Proceedings of the 13th Inter-
national Joint Conference on Biomedical Engineering
Systems and Technologies - Volume 2: BIOIMAGING,
pages 82–92. INSTICC, SciTePress.
Maddalena, L., Granata, I., Manipur, I., Manzo, M., and
Guarracino, M. R. (2021). A framework based on
metabolic networks and biomedical images data to
discriminate glioma grades. In Ye, X., Soares, F.,
De Maria, E., G
´
omez Vilda, P., Cabitza, F., Fred,
A., and Gamboa, H., editors, Biomedical Engineer-
ing Systems and Technologies, pages 165–189, Cham.
Springer International Publishing.
Mart
´
ı-Juan, G., Sanroma-Guell, G., and Piella, G. (2020).
A survey on machine and statistical learning for longi-
tudinal analysis of neuroimaging data in Alzheimer’s
disease. Comput. Methods Programs Biomed.,
189:105348.
Matthews, B. (1975). Comparison of the predicted and
observed secondary structure of T4 phage lysozyme.
Biochimica et Biophysica Acta (BBA) - Protein Struc-
ture, 405(2):442–451.
Mueller, S., Weiner, M., Thal, L., Petersen, R., Jack, C.,
Jagust, W., Trojanowski, J., Toga, A., and Beck-
ett, L. (2005). Ways toward an early diagnosis in
Alzheimer’s disease: the Alzheimer’s Disease Neu-
roimaging initiative (ADNI). J Alzheimers Dement.,
1(1):55–66.
Nho, K., ADNI, et al. (2016). Integration of bioinformatics
and imaging informatics for identifying rare PSEN1
variants in Alzheimer’s disease. BMC Medical Ge-
nomics, 9(Suppl 1).
Peng, J., An, L., Zhu, X., Jin, Y., and Shen, D. (2016).
Structured sparse kernel learning for imaging genet-
ics based Alzheimer’s disease diagnosis. In Inter-
national Conference on Medical Image Computing
and Computer-Assisted Intervention, pages 70–78.
Springer.
Routier, A., Burgos, N., D
´
ıaz, M., Bacci, M., Bottani, S., El-
Rifai, O., Fontanella, S., Gori, P., Guillon, J., Guyot,
A., Hassanaly, R., Jacquemont, T., Lu, P., Marcoux,
A., Moreau, T., Samper-Gonz
´
alez, J., Teichmann, M.,
Thibeau-Sutre, E., Vaillant, G., Wen, J., Wild, A.,
Habert, M.-O., Durrleman, S., and Colliot, O. (2021).
Clinica: An open-source software platform for re-
producible clinical neuroscience studies. Frontiers in
Neuroinformatics, 15:39.
Samper-Gonz
´
alez, J., Burgos, N., Bottani, S., Fontanella,
S., Lu, P., Marcoux, A., Routier, A., Guillon, J., Bacci,
M., Wen, J., Bertrand, A., Bertin, H., Habert, M. O.,
Durrleman, S., Evgeniou, T., and Colliot, O. (2018).
Reproducible evaluation of classification methods in
Alzheimer’s disease: Framework and application to
MRI and PET data. NeuroImage, 183:504–521.
Senanayake, U., Sowmya, A., and Dawes, L. (2018). Deep
fusion pipeline for mild cognitive impairment diagno-
sis. In 2018 IEEE 15th International Symposium on
Biomedical Imaging (ISBI 2018), pages 1394–1997.
Shi, J., Zheng, X., Li, Y., Zhang, Q., and Ying, S. (2018).
Multimodal neuroimaging feature learning with mul-
BIOIMAGING 2022 - 9th International Conference on Bioimaging
78