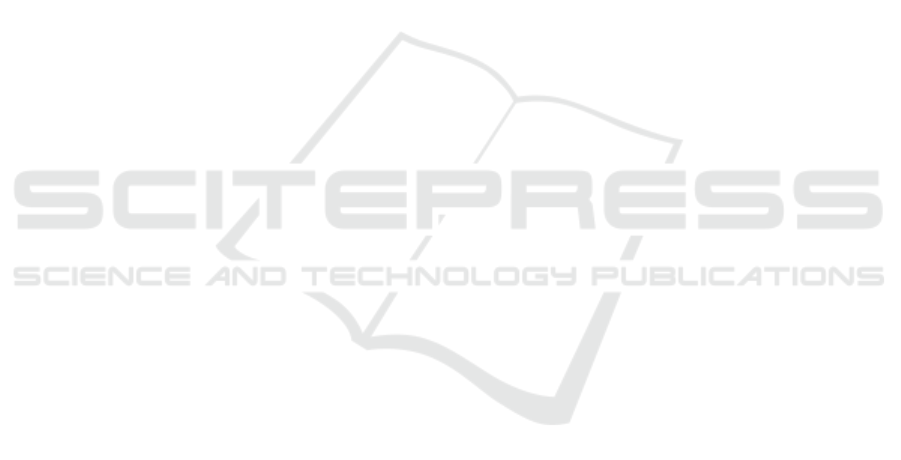
5 FUTURE WORK
The proposed wearable technology could serve for
obtaining knowledge regarding causal relationships
of electrolyte fluctuations with arrhythmia develop-
ment and sudden cardiac death. Algorithms for iden-
tification of the causal direction, coupling delay, and
causal chain relations from time series could be ap-
plied (Huang et al., 2020).
Information on the occurrence of life-threatening
conditions is valuable for developing a system for per-
sonalized decision support, for instance, implemented
as a deep recurrent neural network based on long
short-term memory, such as described in (Kwon et al.,
2018). The neural network can consist of three time
series inputs involving information on signal quality,
electrolyte fluctuations, and temporal distribution of
arrhythmia episodes. Temporal distribution that car-
ries important information about arrhythmia progres-
sion can be characterized using a model-based ap-
proach (Henriksson et al., 2021). The output of the
personalized decision support system may be a sud-
den cardiac death risk score.
The proposed framework for personalized deci-
sion support can potentially be adapted for other
groups with an increased risk of electrolyte fluctua-
tions and life-threatening arrhythmias, e.g., those with
heart failure or receiving chemotherapy treatment.
6 CONCLUSION
An unobtrusive noninvasive technology for monitor-
ing electrolyte fluctuations and detecting ventricular
tachycardia and extreme bradycardia in a home en-
vironment can be of value for identifying patients
susceptible to dangerous arrhythmias precipitated by
electrolyte imbalance.
ACKNOWLEDGMENTS
This work was supported by the European Regional
Development Fund with the Research Council of
Lithuania under the Project 01.2.2-LMT-K-718-01-
0030.
REFERENCES
Aboy, M., McNames, J., Tran Thong, Tsunami, D., El-
lenby, M. S., and Goldstein, B. (2005). An au-
tomatic beat detection algorithm for pressure sig-
nals. IEEE Transactions on Biomedical Engineering,
52(10):1662–1670.
Attia, Z. I., DeSimone, C. V., Dillon, J. J., Sapir, Y.,
Somers, V. K., Dugan, J. L., Bruce, C. J., Acker-
man, M. J., Asirvatham, S. J., Striemer, B. L., et al.
(2016). Novel bloodless potassium determination us-
ing a signal-processed single-lead ECG. Journal of
the American Heart Association, 5(1):e002746.
Bonomi, A. G., Eerik
¨
ainen, L. M., Schipper, F., Aarts,
R. M., De Morree, H. M., and Dekker, L. (2017).
Detecting episodes of brady- and tachycardia using
photo-plethysmography at the wrist in free-living con-
ditions. In 2017 Computing in Cardiology (CinC),
pages 1–4. IEEE.
Bonomi, A. G., Schipper, F., Eerik
¨
ainen, L. M., Margar-
ito, J., Van Dinther, R., Muesch, G., De Morree,
H. M., Aarts, R. M., Babaeizadeh, S., McManus,
D. D., et al. (2018). Atrial fibrillation detection using
a novel cardiac ambulatory monitor based on photo-
plethysmography at the wrist. Journal of the Ameri-
can Heart Association, 7(15):e009351.
Boriani, G., Glotzer, T. V., Santini, M., West, T. M.,
De Melis, M., Sepsi, M., Gasparini, M., Lewalter,
T., Camm, J. A., and Singer, D. E. (2014). Device-
detected atrial fibrillation and risk for stroke: an anal-
ysis of >10 000 patients from the SOS AF project
(Stroke preventiOn Strategies based on Atrial Fibrilla-
tion information from implanted devices). European
Heart Journal, 35(8):508–516.
Brunelli, S. M., Du Mond, C., Oestreicher, N., Rakov, V.,
and Spiegel, D. M. (2017). Serum potassium and
short-term clinical outcomes among hemodialysis pa-
tients: impact of the long interdialytic interval. Amer-
ican Journal of Kidney Diseases, 70(1):21–29.
Corsi, C., Cortesi, M., Callisesi, G., Bie, J. D., Napolitano,
C., Santoro, A., Mortara, D., and Severi, S. (2017).
Noninvasive quantification of blood potassium con-
centration from ECG in hemodialysis patients. Sci-
entific Reports, 7(1).
El-Sherif, N. and Turitto, G. (2011). Electrolyte disorders
and arrhythmogenesis. Cardiology Journal, 18(3):13.
Henriksson, M., Mart
´
ın-Yebra, A., Butkuvien
˙
e, M., Ras-
mussen, J. G., Marozas, V., Petr
˙
enas, A., Savelev, A.,
Platonov, P. G., and S
¨
ornmo, L. (2021). Modeling and
estimation of temporal episode patterns in paroxysmal
atrial fibrillation. IEEE Transactions on Biomedical
Engineering, 68(1):319–329.
Huang, Y., Fu, Z., and Franzke, C. L. E. (2020). Detect-
ing causality from time series in a machine learning
framework. Chaos: An Interdisciplinary Journal of
Nonlinear Science, 30(6):063116.
Kalra, P. A., Green, D., and Poulikakos, D. (2018). Arrhyth-
mia in hemodialysis patients and its relation to sudden
death. Kidney International, 93(4):781–783.
Kwon, J.-m., Lee, Y., Lee, Y., Lee, S., and Park, J. (2018).
An algorithm based on deep learning for predicting in-
hospital cardiac arrest. Journal of the American Heart
Association, 7(13):e008678.
Loewe, A., Lutz, Y., Nairn, D., Fabbri, A., Nagy, N.,
Toth, N., Ye, X., Fuertinger, D. H., Genovesi, S.,
Personalized Evaluation of Life-threatening Conditions in Chronic Kidney Disease Patients: The Concept of Wearable Technology and Case
Analysis
249