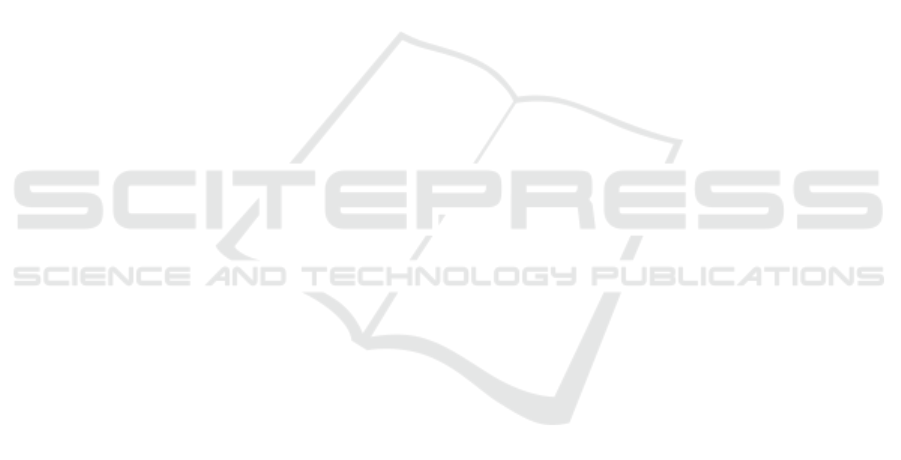
metric correlates better at higher compression than
SSIM and PSNR. One advantage of our metric is that
it depends on both image and model used for feature
extraction. The metric will change based on features
extracted from images. In the future, we will focus on
determining the quality of image patches.
ACKNOWLEDGEMENTS
This work was supported in part by Grant No.
60NANB17D178 from U.S. Dept. of Commerce, Na-
tional Institute of Standards and Technology.
REFERENCES
Aqqa, M., Mantini, P., and Shah, S. K. (2019). Understand-
ing how video quality affects object detection algo-
rithms. In VISIGRAPP (5: VISAPP), pages 96–104.
Beniwal, P., Mantini, P., and Shah, S. K. (2019). Assessing
the impact of video compression on background sub-
traction. In Asian Conference on Pattern Recognition,
pages 105–118. Springer.
Best-Rowden, L. and Jain, A. K. (2017). Automatic
face image quality prediction. arXiv preprint
arXiv:1706.09887.
Best-Rowden, L. and Jain, A. K. (2018). Learning
face image quality from human assessments. IEEE
Transactions on Information forensics and security,
13(12):3064–3077.
Chen, J., Deng, Y., Bai, G., and Su, G. (2014). Face image
quality assessment based on learning to rank. IEEE
signal processing letters, 22(1):90–94.
Everingham, M., Van Gool, L., Williams, C. K., Winn, J.,
and Zisserman, A. (2010). The pascal visual object
classes (voc) challenge. International journal of com-
puter vision, 88(2):303–338.
Gao, F., Yu, J., Zhu, S., Huang, Q., and Tian, Q. (2018).
Blind image quality prediction by exploiting multi-
level deep representations. Pattern Recognition,
81:432–442.
Guo, C., Pleiss, G., Sun, Y., and Weinberger, K. Q. (2017).
On calibration of modern neural networks. In Interna-
tional Conference on Machine Learning, pages 1321–
1330. PMLR.
Janowski, L. and Papir, Z. (2009). Modeling subjective
tests of quality of experience with a generalized linear
model. In 2009 International Workshop on Quality of
Multimedia Experience, pages 35–40. IEEE.
Kong, L., Ikusan, A., Dai, R., and Zhu, J. (2019). Blind im-
age quality prediction for object detection. In 2019
IEEE Conference on Multimedia Information Pro-
cessing and Retrieval (MIPR), pages 216–221. IEEE.
Krizhevsky, A., Sutskever, I., and Hinton, G. E. (2012). Im-
agenet classification with deep convolutional neural
networks. Advances in neural information processing
systems, 25:1097–1105.
Lin, T.-Y., Maire, M., Belongie, S., Hays, J., Perona, P.,
Ramanan, D., Doll
´
ar, P., and Zitnick, C. L. (2014).
Microsoft coco: Common objects in context. In Euro-
pean conference on computer vision, pages 740–755.
Springer.
Ma, K., Liu, W., Zhang, K., Duanmu, Z., Wang, Z., and
Zuo, W. (2017). End-to-end blind image quality as-
sessment using deep neural networks. IEEE Transac-
tions on Image Processing, 27(3):1202–1213.
Mu, M., Romaniak, P., Mauthe, A., Leszczuk, M.,
Janowski, L., and Cerqueira, E. (2012). Framework
for the integrated video quality assessment. Multime-
dia Tools and Applications, 61(3):787–817.
Ren, S., He, K., Girshick, R., and Sun, J. (2015). Faster
r-cnn: Towards real-time object detection with region
proposal networks. Advances in neural information
processing systems, 28:91–99.
Schlett, T., Rathgeb, C., Henniger, O., Galbally, J., Fier-
rez, J., and Busch, C. (2020). Face image qual-
ity assessment: A literature survey. arXiv preprint
arXiv:2009.01103.
Shi, Y. and Jain, A. K. (2019). Probabilistic face embed-
dings. In Proceedings of the IEEE/CVF International
Conference on Computer Vision, pages 6902–6911.
Terhorst, P., Kolf, J. N., Damer, N., Kirchbuchner, F., and
Kuijper, A. (2020). Ser-fiq: Unsupervised estimation
of face image quality based on stochastic embedding
robustness. In Proceedings of the IEEE/CVF Con-
ference on Computer Vision and Pattern Recognition,
pages 5651–5660.
Wallace, G. K. (1992). The jpeg still picture compression
standard. IEEE transactions on consumer electronics,
38(1):xviii–xxxiv.
Wang, Z., Lu, L., and Bovik, A. C. (2004). Video quality as-
sessment based on structural distortion measurement.
Signal processing: Image communication, 19(2):121–
132.
Wiegand, T., Sullivan, G. J., Bjontegaard, G., and Luthra,
A. (2003). Overview of the h. 264/avc video coding
standard. IEEE Transactions on circuits and systems
for video technology, 13(7):560–576.
Yang, X., Li, F., and Liu, H. (2019). A survey of dnn meth-
ods for blind image quality assessment. IEEE Access,
7:123788–123806.
Zhai, G. and Min, X. (2020). Perceptual image quality as-
sessment: a survey. Science China Information Sci-
ences, 63(11):211301.
VISAPP 2022 - 17th International Conference on Computer Vision Theory and Applications
714