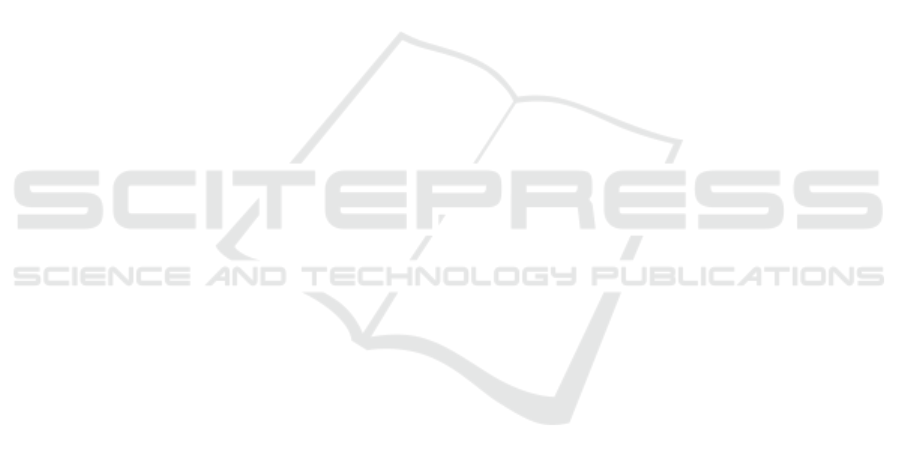
Liu, H. (2021). Biosignal Processing and Activity Model-
ing for Multimodal Human Activity Recognition. PhD
thesis, University of Bremen.
Liu, H., Hartmann, Y., and Schultz, T. (2021a). CSL-
SHARE: A multimodal wearable sensor-based human
activity dataset. Frontiers in Computer Science, 3:90.
Liu, H., Hartmann, Y., and Schultz, T. (2021b). Mo-
tion Units: Generalized sequence modeling of hu-
man activities for sensor-based activity recognition. In
EUSIPCO 2021 — 29th European Signal Processing
Conference, pages 1506–1510.
Liu, H. and Schultz, T. (2018). ASK: A framework for data
acquisition and activity recognition. In Proceedings of
the 11th International Joint Conference on Biomedi-
cal Engineering Systems and Technologies - Volume 3:
BIOSIGNALS, pages 262–268. INSTICC, SciTePress.
Liu, H. and Schultz, T. (2019). A wearable real-time hu-
man activity recognition system using biosensors in-
tegrated into a knee bandage. In Proceedings of the
12th International Joint Conference on Biomedical
Engineering Systems and Technologies - Volume 1:
BIODEVICES, pages 47–55. INSTICC, SciTePress.
Liu, H. and Schultz, T. (2022). How long are various
types of daily activities? statistical analysis of a multi-
modal wearable sensor-based human activity dataset.
In HEALTHINF 2022 — 15th International Confer-
ence on Health Informatics. INSTICC, SciTePress.
forthcoming.
Long, J., Sun, W., Yang, Z., and Raymond, O. I. (2019).
Asymmetric residual neural network for accurate hu-
man activity recognition. Information, 10(6):203.
Loose, H., Tetzlaff, L., and Bolmgren, J. L. (2020). A public
dataset of overground and treadmill walking in healthy
individuals captured by wearable imu and semg sen-
sors. In Biosignals 2020 - 13th International Confer-
ence on Bio-Inspired Systems and Signal Processing,
pages 164–171. INSTICC, SciTePress.
Lukowicz, P., Ward, J. A., Junker, H., St
¨
ager, M., Tr
¨
oster,
G., Atrash, A., and Starner, T. (2004). Recognizing
workshop activity using body worn microphones and
accelerometers. In In Pervasive Computing, pages 18–
32.
Micucci, D., Mobilio, M., and Napoletano, P. (2017).
UniMiB SHAR: A dataset for human activity recog-
nition using acceleration data from smartphones. Ap-
plied Sciences, 7(10):1101.
Peng, H., Long, F., and Ding, C. (2005). Feature se-
lection based on mutual information criteria of max-
dependency, max-relevance, and min-redundancy.
IEEE Transactions on pattern analysis and machine
intelligence, 27(8):1226–1238.
Rabiner, L. R. (1989). A tutorial on hidden markov mod-
els and selected applications in speech recognition. In
Proceedings of the IEEE, volume 77(2), pages 257–
286.
Rebelo, D., Amma, C., Gamboa, H., and Schultz, T. (2013).
Human activity recognition for an intelligent knee or-
thosis. In BIOSIGNALS 2013 - 6th International Con-
ference on Bio-inspired Systems and Signal Process-
ing, pages 368–371.
Reiss, A. and Stricker, D. (2012a). Creating and bench-
marking a new dataset for physical activity monitor-
ing. In Proceedings of the 5th International Confer-
ence on Pervasive Technologies Related to Assistive
Environments, pages 1–8.
Reiss, A. and Stricker, D. (2012b). Introducing a new
benchmarked dataset for activity monitoring. In ISWC
2012 - 16th International Symposium on Wearable
Computers, pages 108–109. IEEE.
Roggen, D., Calatroni, A., Rossi, M., Holleczek, T., F
¨
orster,
K., Tr
¨
oster, G., Lukowicz, P., Bannach, D., Pirkl, G.,
Ferscha, A., et al. (2010). Collecting complex activ-
ity datasets in highly rich networked sensor environ-
ments. In INSS 2010 - 7th International Conference
on Networked Sensing Systems, pages 233–240. IEEE.
Ronaoo, C. A. and Cho, S.-B. (2015). Evaluation of deep
convolutional neural network architectures for human
activity recognition with smartphone sensors. Jour-
nal of the Korean Information Science Society, pages
858–860.
Santos, S., Folgado, D., and Gamboa, H. (2020). Upper-
body movements: Precise tracking of human motion
using inertial sensors.
Singh, D., Merdivan, E., Psychoula, I., Kropf, J., Hanke,
S., Geist, M., and Holzinger, A. (2017). Human ac-
tivity recognition using recurrent neural networks. In
CD-MAKE 2017 - International Cross-Domain Con-
ference for Machine Learning and Knowledge Extrac-
tion, pages 267–274. Springer.
St, L., Wold, S., et al. (1989). Analysis of variance
(anova). Chemometrics and intelligent laboratory sys-
tems, 6(4):259–272.
Sztyler, T. and Stuckenschmidt, H. (2016). On-body lo-
calization of wearable devices: An investigation of
position-aware activity recognition. In PerCom 2016
- 14th IEEE International Conference on Pervasive
Computing and Communications, pages 1–9. IEEE
Computer Society.
Telaar, D., Wand, M., Gehrig, D., Putze, F., Amma, C.,
Heger, D., Vu, N. T., Erhardt, M., Schlippe, T.,
Janke, M., et al. (2014). Biokit—real-time decoder
for biosignal processing. In INTERSPEECH 2014 -
15th Annual Conference of the International Speech
Communication Association.
Tuncer, T., Ertam, F., Dogan, S., Aydemir, E., and Pławiak,
P. (2020). Ensemble residual network-based gender
and activity recognition method with signals. The
Journal of Supercomputing, 76(3):2119–2138.
Weinland, D., Ronfard, R., and Boyer, E. (2011). A sur-
vey of vision-based methods for action representation,
segmentation and recognition. Computer vision and
image understanding, 115(2):224–241.
Xue, T. and Liu, H. (2021). Hidden Markov Model and its
application in human activity recognition and fall de-
tection: A review. In CSPS 2021 - 10th International
Conference on Communications, Signal Processing,
and Systems. forthcoming.
WHC 2022 - Special Session on Wearable HealthCare
856