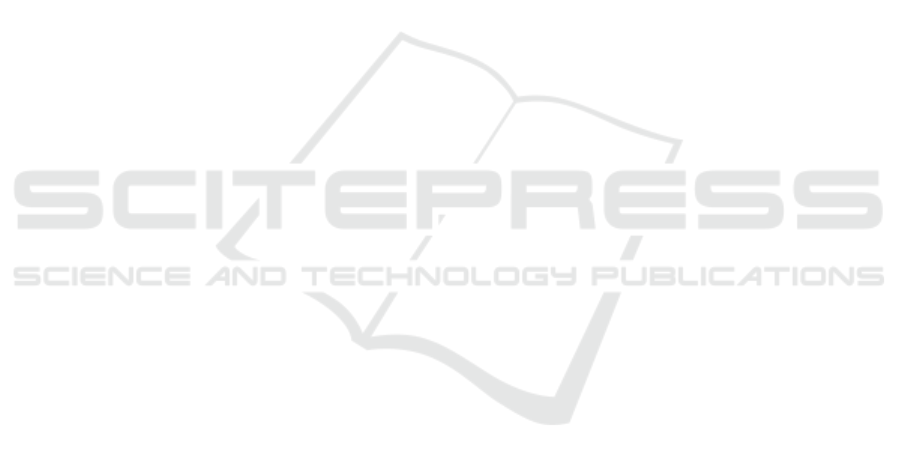
novel BCI paradigms such as inner speech classifica-
tion (van den Berg et al., 2021) could be employed
to increase the system accuracy, number of the con-
trol commands, and ease of use for the user (Hong
and Khan, 2017). For instance, the motor imagery
paradigm can be integrated as a more intuitive method
for navigation (Su et al., 2011) or the P300 paradigm
can be paired with gaze or attention tracking as an
on/off switch for active/passive control of the inter-
face (Alimardani and Hiraki, 2020). Such a multi-
modal interface will enable asynchronous communi-
cation with the BCI system whenever the user intends
to interact with the environment, which would in re-
turn reduce visual strain from the continuous flashing
of the stimuli.
In sum, robot-assisted smart home systems that
focus on the needs of disabled patients could improve
their quality of life and reduce their reliance on care-
takers, which is beneficial in both healthcare and pri-
vate home care settings. A VR simulation allows re-
searchers to fully consider all aspects of the user expe-
rience before committing to development phases and
to ensure that the potential user groups can benefit
from the system in the long term. While more user
research, prototyping, and testing are still needed, our
application demonstrates the first steps of this process
as a proof of concept.
4 CONCLUSION
In this paper, we presented a proof of concept for a
BCI-controlled robot assistant in a VR-based smart
home that enables patients with motor impairment
to conduct complex tasks such as object manipu-
lation and environment control. Our solution in-
tegrated a VR environment with a P300 BCI sys-
tem through a custom-designed controller interface;
it demonstrated that BCI commands issued via a
custom-designed household-oriented P300 interface
can be sufficient to control a combination of smart
home appliances and an assistive robot. This com-
bination serves as an affordable platform for evalua-
tion and design of real smart home environments for
disabled patients. Further developments in BCI hard-
ware/software, robotics, and VR input methods are
required to realize automated assisted living systems
efficiently.
REFERENCES
Abiri, R., Borhani, S., Sellers, E. W., Jiang, Y., and Zhao, X.
(2019). A comprehensive review of eeg-based brain-
computer interface paradigms. Journal of neural en-
gineering, 16.
Alimardani, M. and Hiraki, K. (2020). Passive brain-
computer interfaces for enhanced human-robot inter-
action. Frontiers in Robotics and AI, 7.
Alimardani, M., Nishio, S., and Ishiguro, H. (2013).
Humanlike robot hands controlled by brain activity
arouse illusion of ownership in operators. Scientific
reports, 3:2396.
Demon, R. (2020). Robot sphere. https://assetstore.unity.
com/packages/3d/characters/robots/robot-sphere-
136226.
Do, H. M., Pham, M., Sheng, W., Yang, D., and Liu,
M. (2018). Rish: A robot-integrated smart home
for elderly care. Robotics and Autonomous Systems,
101:74–92.
Edlinger, G. and Guger, C. (2011). Social environments,
mixed communication and goal-oriented control ap-
plication using a brain-computer interface.
Edlinger, G., Holzner, C., Groenegress, C., Guger, C.,
and Slater, M. (2009). Goal-oriented control with
brain-computer interface. Lecture Notes in Computer
Science (including subseries Lecture Notes in Artifi-
cial Intelligence and Lecture Notes in Bioinformatics),
5638 LNAI:732–740.
Fazel-Rezai, R., Allison, B. Z., Guger, C., Sellers, E. W.,
Kleih, S. C., and K
¨
ubler, A. (2012). P300 brain
computer interface: Current challenges and emerging
trends. Frontiers in Neuroengineering, 0:1–30.
Guger, C., Daban, S., Sellers, E., Holzner, C., Krausz,
G., Carabalona, R., Gramatica, F., and Edlinger, G.
(2009). How many people are able to control a p300-
based brain-computer interface (bci)? Neuroscience
Letters, 462:94–98.
Holzner, C., Guger, C., Edlinger, G., Gronegress, C., and
Slater, M. (2009). Virtual smart home controlled by
thoughts. 2009 18th IEEE International Workshops
on Enabling Technologies: Infrastructures for Collab-
orative Enterprises, pages 236–239.
Hong, K.-S. and Khan, M. J. (2017). Hybrid
brain–computer interface techniques for improved
classification accuracy and increased number of com-
mands: a review. Frontiers in neurorobotics, 11:35.
Huggins, J. E., Wren, P. A., and Gruis, K. L. (2011). What
would brain-computer interface users want? opinions
and priorities of potential users with amyotrophic lat-
eral sclerosis. Amyotrophic Lateral Sclerosis, 12:318–
324.
Hung, L., Gregorio, M., Mann, J., Wallsworth, C., Horne,
N., Berndt, A., Liu, C., Woldum, E., Au-Yeung, A.,
and Chaudhury, H. (2021). Exploring the perceptions
of people with dementia about the social robot paro in
a hospital setting. Dementia, 20.
Koceski, S. and Koceska, N. (2016). Evaluation of an assis-
tive telepresence robot for elderly healthcare. Journal
of Medical Systems, 40:121.
K
¨
athner, I., K
¨
ubler, A., and Halder, S. (2015). Rapid p300
brain-computer interface communication with a head-
mounted display. Frontiers in Neuroscience, 9.
BIODEVICES 2022 - 15th International Conference on Biomedical Electronics and Devices
236