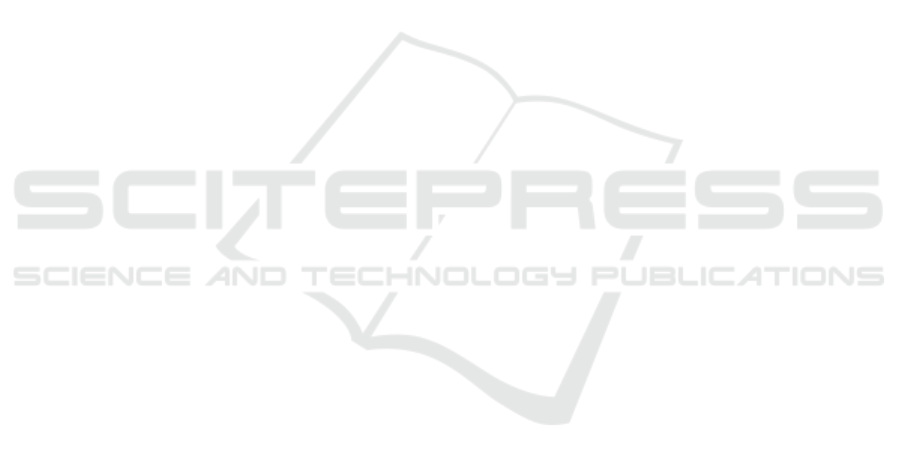
mani, Z., Welling, M., Cortes, C., Lawrence, N., and
Weinberger, K., editors, Advances in Neural Infor-
mation Processing Systems, volume 27. Curran Asso-
ciates, Inc.
H
¨
ark
¨
onen, E., Hertzmann, A., Lehtinen, J., and Paris, S.
(2020). Ganspace: Discovering interpretable gan con-
trols. In Larochelle, H., Ranzato, M., Hadsell, R.,
Balcan, M., and Lin, H., editors, Advances in Neu-
ral Information Processing Systems, volume 33, pages
9841–9850. Curran Associates, Inc.
Isola, P., Zhu, J., Zhou, T., and Efros, A. A. (2017a). Image-
to-image translation with conditional adversarial net-
works. In 2017 IEEE Conference on Computer Vision
and Pattern Recognition, CVPR 2017, Honolulu, HI,
USA, July 21-26, 2017, pages 5967–5976. IEEE Com-
puter Society.
Isola, P., Zhu, J.-Y., Zhou, T., and Efros, A. A. (2017b).
Image-to-image translation with conditional adversar-
ial networks. In 2017 IEEE Conference on Computer
Vision and Pattern Recognition (CVPR), pages 5967–
5976.
Karras, T., Aila, T., Laine, S., and Lehtinen, J. (2017). Pro-
gressive growing of gans for improved quality, stabil-
ity, and variation. CoRR, abs/1710.10196.
Karras, T., Aittala, M., Laine, S., H
¨
ark
¨
onen, E., Hellsten, J.,
Lehtinen, J., and Aila, T. (2021). Alias-free generative
adversarial networks. In Beygelzimer, A., Dauphin,
Y., Liang, P., and Vaughan, J. W., editors, Advances in
Neural Information Processing Systems.
Karras, T., Laine, S., and Aila, T. (2019). A style-based
generator architecture for generative adversarial net-
works. In Proceedings of the IEEE/CVF Conference
on Computer Vision and Pattern Recognition (CVPR).
Karras, T., Laine, S., Aittala, M., Hellsten, J., Lehtinen,
J., and Aila, T. (2020). Analyzing and improving
the image quality of stylegan. In 2020 IEEE/CVF
Conference on Computer Vision and Pattern Recog-
nition, CVPR 2020, Seattle, WA, USA, June 13-19,
2020, pages 8107–8116. Computer Vision Foundation
/ IEEE.
Kim, T., Cha, M., Kim, H., Lee, J. K., and Kim, J. (2017).
Learning to discover cross-domain relations with gen-
erative adversarial networks. In Precup, D. and Teh,
Y. W., editors, Proceedings of the 34th International
Conference on Machine Learning, ICML 2017, Syd-
ney, NSW, Australia, 6-11 August 2017, volume 70
of Proceedings of Machine Learning Research, pages
1857–1865. PMLR.
Larsen, A. B. L., Sønderby, S. K., and Winther, O. (2015).
Autoencoding beyond pixels using a learned similar-
ity metric. CoRR, abs/1512.09300.
Ledig, C., Theis, L., Huszar, F., Caballero, J., Cunning-
ham, A., Acosta, A., Aitken, A. P., Tejani, A., Totz,
J., Wang, Z., and Shi, W. (2017). Photo-realistic sin-
gle image super-resolution using a generative adver-
sarial network. In 2017 IEEE Conference on Com-
puter Vision and Pattern Recognition, CVPR 2017,
Honolulu, HI, USA, July 21-26, 2017, pages 105–114.
IEEE Computer Society.
Lee, H.-Y., Tseng, H.-Y., Mao, Q., Huang, J.-B., Lu, Y.-D.,
Singh, M. K., and Yang, M.-H. (2020). Drit++: Di-
verse image-to-image translation viadisentangled rep-
resentations. International Journal of Computer Vi-
sion, pages 1–16.
Liu, M., Breuel, T. M., and Kautz, J. (2017). Unsuper-
vised image-to-image translation networks. CoRR,
abs/1703.00848.
Liu, Z., Luo, P., Wang, X., and Tang, X. (2015). Deep learn-
ing face attributes in the wild. 2015 IEEE Interna-
tional Conference on Computer Vision (ICCV), pages
3730–3738.
Matyas, J. et al. (1965). Random optimization. Automation
and Remote control, 26(2):246–253.
Mirza, M. and Osindero, S. (2014). Conditional generative
adversarial nets. CoRR, abs/1411.1784.
Nitzan, Y., Bermano, A., Li, Y., and Cohen-Or, D. (2020).
Face identity disentanglement via latent space map-
ping. ACM Trans. Graph., 39(6):225:1–225:14.
Patashnik, O., Wu, Z., Shechtman, E., Cohen-Or, D., and
Lischinski, D. (2021). Styleclip: Text-driven manip-
ulation of stylegan imagery. In Proceedings of the
IEEE/CVF International Conference on Computer Vi-
sion (ICCV), pages 2085–2094.
Radford, A., Kim, J. W., Hallacy, C., Ramesh, A., Goh,
G., Agarwal, S., Sastry, G., Askell, A., Mishkin, P.,
Clark, J., Krueger, G., and Sutskever, I. (2021). Learn-
ing transferable visual models from natural language
supervision. In Meila, M. and Zhang, T., editors, Pro-
ceedings of the 38th International Conference on Ma-
chine Learning, ICML 2021, 18-24 July 2021, Virtual
Event, volume 139 of Proceedings of Machine Learn-
ing Research, pages 8748–8763. PMLR.
Radford, A., Metz, L., and Chintala, S. (2016). Unsu-
pervised representation learning with deep convolu-
tional generative adversarial networks. In Bengio, Y.
and LeCun, Y., editors, 4th International Conference
on Learning Representations, ICLR 2016, San Juan,
Puerto Rico, May 2-4, 2016, Conference Track Pro-
ceedings.
Richardson, E., Alaluf, Y., Patashnik, O., Nitzan, Y., Azar,
Y., Shapiro, S., and Cohen-Or, D. (2021). Encoding
in style: A stylegan encoder for image-to-image trans-
lation. In IEEE Conference on Computer Vision and
Pattern Recognition, CVPR 2021, virtual, June 19-25,
2021, pages 2287–2296. Computer Vision Foundation
/ IEEE.
Shen, Y., Gu, J., Tang, X., and Zhou, B. (2020). Interpreting
the latent space of gans for semantic face editing. In
CVPR.
Shen, Y. and Zhou, B. (2020). Closed-form factorization of
latent semantics in gans. CoRR, abs/2007.06600.
Tov, O., Alaluf, Y., Nitzan, Y., Patashnik, O., and Cohen-Or,
D. (2021). Designing an encoder for stylegan image
manipulation. CoRR, abs/2102.02766.
Voynov, A. and Babenko, A. (2020). Unsupervised discov-
ery of interpretable directions in the GAN latent space.
In III, H. D. and Singh, A., editors, Proceedings of the
37th International Conference on Machine Learning,
volume 119 of Proceedings of Machine Learning Re-
search, pages 9786–9796. PMLR.
Keep It Simple: Local Search-based Latent Space Editing
281